Advances in generative AI have brought the internet to an inflection point. In the not-too-distant future, large language model (LLM)-powered virtual assistants could become a universal gateway to the internet. For company leaders, this will mean making fundamental choices about how they engage with consumers.
On one side of the spectrum, companies will be able to relinquish control of their consumer interface to an LLM-powered virtual assistant (or other conversational AI) using APIs such as plug-ins. OpenAI’s ChatGPT plug-in enables consumers to make meal reservations and order groceries via third-party sites like OpenTable and Instacart; other LLM providers are likely to follow suit.
At the other end, companies can retain control of their interface with a custom generative AI model on their own website and app. The implementation will vary, with companies choosing to build or fine-tune. Bloomberg built its own model that it plans to integrate into its services and features. Expedia incorporated OpenAI’s model into its own application—users stay on the company’s site but plan trips using ChatGPT.
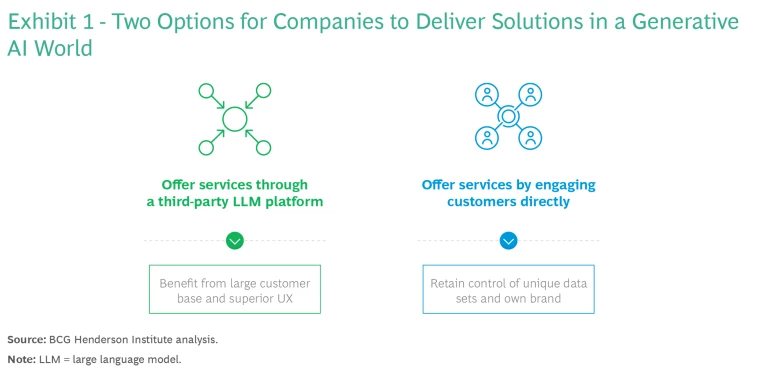
Both strategies—relinquish and retain—have benefits and risks. And the benefit of one option is often a risk of the other. It is also likely that various options will be beneficial for different use cases, based on specific needs and risk tolerance. We’ve researched these evolving market dynamics to offer insight into choosing among and activating each strategy. (See Exhibit 1.) Now is the time to do so—before the future is defined by early movers.
Embracing the Third-Party Platform
LLM providers are making a platform play, offering companies the ability to engage their customers through the LLMs’ platform. The customer interface is a chatbot like ChatGPT; in the future, the chatbot could be replaced by a powerful virtual assistant. The most apt comparison is WeChat, China’s chat app-turned-super app—used by 1.3 billion people monthly to access a wide range of products and
But the LLM models could also go a step further in terms of user experience. It may be possible in the future for consumers to search for services in a more conversational, automated way. For example, someone planning a vacation can tell the virtual assistant her preferences (dates, destination, budget, so on), and it will create an itinerary, manage any requested adjustments, and make the reservations.
The virtual assistant would make choices about which options to show the customer, versus an algorithm sequencing all available options. And the virtual assistant could become a “trusted agent” and keep users on the platform by doing the work, from search to final transaction—all without users needing to sift through options on their own. This shift will dramatically alter the dynamics of search engine optimization and customer engagement strategies.
How Traditional Companies Can Benefit
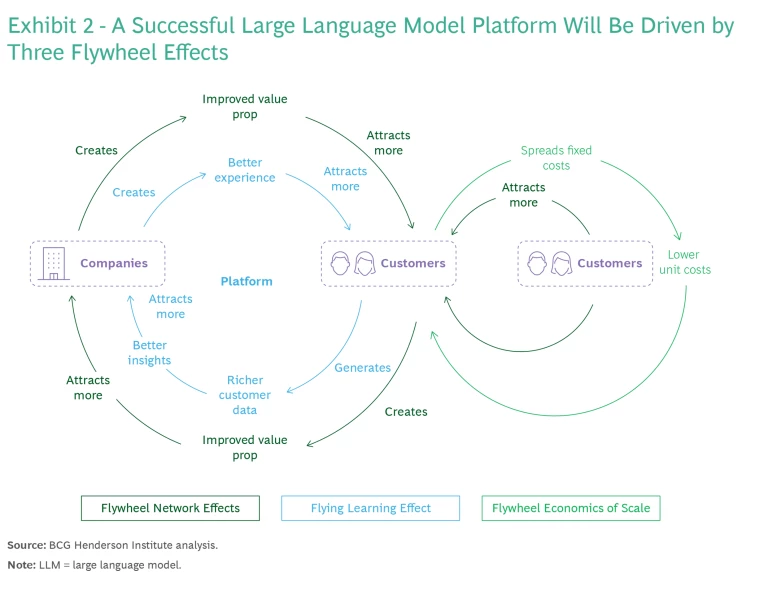
Integrating a third-party LLM-powered virtual assistant with a plug-in or other API is the quickest and easiest option to reach new customers in a generative AI world. The use of platforms to offer services is a proven way for companies to easily engage with a large and established customer base—one that appreciates having a wide variety of services accessible from a single location. Although conversational AI (such as chatbots) still have significant ground to make up compared to established platforms like WeChat and Amazon, the novelty of the experience is driving customer engagement. And that engagement is accelerating at record pace the three powerful flywheels that drive platform success—scale, learning, and network. The success of the platform is also likely to drive the success of companies on the platform. (See Exhibit 2.)
Scale Effect. The cost of large, generalized models (which are the most likely models to be used for virtual assistants, because of their broad functionality and superior conversational ability) is notoriously high. (See “Building a Best-in-Class Large Language Model.”) But we expect that LLM providers will be able to distribute their substantial R&D and running costs over what will be a large userbase, giving them valuable economies of scale. As a result, companies that want to engage with customers with virtual assistants can do so without building the models themselves.
Building a Best-in-Class Large Language Model
Building a small, single-task model is often more cost effective, ranging from $100,000 to $5 million and up depending on the complexity of prepping the data and the functional requirements of the desired task. For example, a well-known regional bank trained a small, task-specific language model for internal loan adjudication purposes and spent between $150,000 to $200,000 and up end-to-end for their foundation model implementation.
In contrast to building a model, the cost to modify (for example, fine-tune) an existing model is the most affordable option, ranging from $10,000 to $100,000 and up.
The key ingredient to train or fine-tune these models is access to high-quality proprietary data. The data also needs to be cleaned, sometimes labeled (for particular use cases), and ideally anonymized for use in fine-tuning or training an LLM. This is no small ask: BloombergGPT was trained on a massive 363 billion token dataset using Bloomberg’s extensive, pre-existing financial dataset (which includes proprietary Bloomberg data), the FinPile dataset (a compilation of financial documents from the Bloomberg archives), and external sources such as press reports.
Learning Effect. The excitement surrounding generative AI is encouraging users to experiment with applications such as ChatGPT and Bard. Both chatbots have benefited from the learning effect (also known as the direct network effect) generated by this surge in experimentation: They improve as more people use them. For companies that decide to offer services through an established platform, this learning effect provides a significant advantage—they’ll have access to superior user experience and best-in-class conversational interfaces.
Same-Side and Cross-Side Network Effect. As more companies join LLM platforms, consumers will find greater value and new users will gravitate to the platform (the same-side network effect), which in turn drives more companies to integrate their services with the platform (the cross-side network effect). These network effects present a significant opportunity for companies to engage with a wide user base and attract high volumes of customers.
Risks for Traditional Companies
Many companies today are concerned about the operational risks of using an LLM’s interface. For example, providing services through an LLM-powered virtual assistant could potentially expose a company’s proprietary data to the LLM vendor. However, many of these risks can be mitigated with technology implementations and vendor contracting.
But companies also face strategic risks that may not currently be on their radar. One key risk, commoditization from intermediation, emerges when an intermediary between a company and its customers reduces emphasis on the company’s unique selling points. Much like search engines, virtual assistants will have to prioritize which services are displayed to the customer and can take commissions on sales. The result is often lower margins and standardization of services—making brand recognition and promotion of premium offerings more difficult. This risk grows as more companies join the platform. The question of how an LLM-powered virtual assistant will select (or help the customer select) one company’s service or product out of a list of common services and products is unknown, putting companies at higher risk for commoditization.
There is also an inherent risk in relying too significantly on a third-party sales channel. This risk is illustrated by the vacation planning example above. When a customer books through a third-party virtual assistant rather than with the airline or hotel chains that provide the actual service, the virtual assistant provider has control over the engagement logs and how services are selected, and heavily influences customer buying behavior. As a result, companies could lose direct connections with customers, and the critical engagement data that enables them to build brand loyalty and cultivate ongoing customer relationships.
Engaging Customers Directly Through Customization
Companies that have access to valuable, domain-specific, proprietary data may choose to double down on their competitive advantage—creating their own LLM-driven customer experiences with generative AI. The tradeoff is typically in the homegrown user experience, compared to LLM-powered virtual assistants where providers are pouring resources into optimizing human engagement. Specialized models designed in-house need to be user-friendly enough to support their customer offerings and encourage customers to return.
The good news is that many small models, such as Alpaca (a 7-billion-parameter language model created at Stanford University) and Dolly (a 12-billion-parameter language model created by Databricks), are not as cumbersome and costly to customize as those required for the more expansive virtual assistants. And creating specialized models, for example, those built through fine-tuning or retraining, with proprietary data can provide superior performance for a specialized task. The better the data is, the better the model is at performing the task that the data is related to—though possibly at a cost of its language capabilities.
It is also possible to add functionality and value to raw data by adding a layer of analysis. BloombergGPT (a 50-billion-parameter language model), for example, outperformed general purpose models for highly specific financial tasks, such as financial risk assessment.
How Traditional Companies Can Benefit
Companies that choose to create their own customized experiences can maintain exclusive access to their valuable, proprietary data and ensure it remains secure. In-house control allows companies greater flexibility to create unique functionalities and user experiences without depending on another company’s technical roadmap. In the case of BloombergGPT, the user gets more refined and accurate financial data, and in return, Bloomberg gets more tailored user-interaction data that can be used to continuously update their LLMs.
When companies keep direct access to their customer base, they can benefit from the rich data gleaned from customer engagement. This allows companies to better understand their customers and cultivate stronger, mutually beneficial relationships. It also strengthens companies’ ability to build customer trust by providing a sense of security and confidentiality, while promoting their brand name. For more sensitive interactions, such as viewing a bank statement, this is particularly valuable; consumers typically prefer to use a service offered directly from the bank itself.
Risks for Traditional Companies
The obvious operational risk surrounding this option will be the simple fact that investing in in-house capabilities can be cost prohibitive. But companies don’t need to take the most expensive approach and build from scratch: they can fine-tune free, open-source models or bring in someone else’s model and incorporate it into their own website.
Leaders also need to consider the less-obvious strategic risks. For one, they’ll need to keep up with the requirements to build and maintain best-in-class capabilities in-house. (See “Building a Best-in-Class Large Language Model.”) Specialized models need to have “good enough” functionality and usability to attract and retain customers. But the definition of “good enough” for customers will evolve alongside the experiences of best-in-class models and platforms. And the data science and engineering talent needed to manage these models is currently a scarce resource.
In addition, the R&D necessary to maintain a best-in-class model likely won’t be feasible for most companies, as LLM research becomes more proprietary. Making that task more difficult is the fact that some best-in-class model providers don’t allow companies to customize the model for their own purposes.
Companies that choose this option also risk missing out on a critical customer engagement channel. If companies don’t put any of their services on a popular LLM-powered virtual assistant, they could become alienated from their customer base—many of whom may have grown accustomed to using that assistant instead of coming to the companies’ website.
Choosing and Activating Your Strategy
The generative AI world is one of constant motion, making it challenging to track how the market dynamics are evolving. It may be tempting to integrate an LLM’s plug-in today, no questions asked. And for some companies—for instance, those with a small market share, a small customer base, or low-quality data or lack of access to strong proprietary data, and that don’t have a strong user experience—this will be a smart strategic move.
But with every benefit comes a risk. And companies with a strong customer base and unique offering may be better served by maintaining control of their user experience and providing a virtual assistant service in-house.
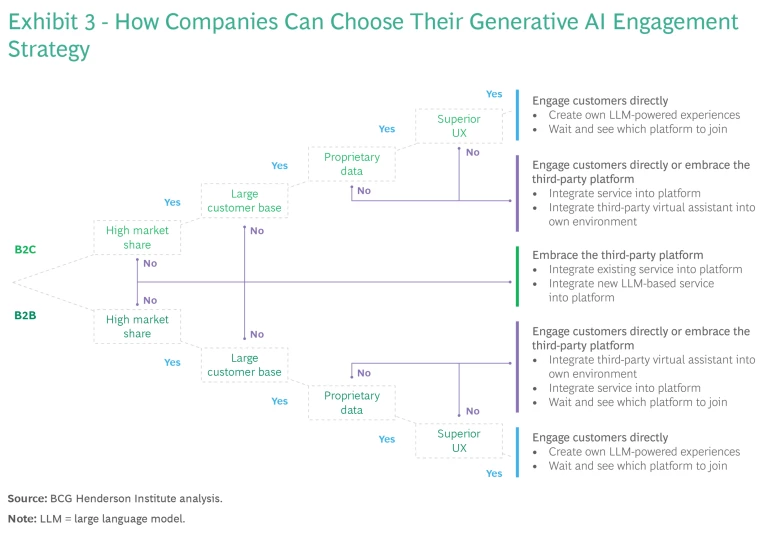
Most companies will likely explore both strategic options. Once they choose, there are also a host of nuanced operational paths that a company can pursue to balance the risks and benefits of each choice; for example, building their own model, incorporating a best-in-class website into a direct-to-consumer interface or fine-tuning a best-in-class model to enable more customization, and then providing that model through a third-party platform. (See Exhibit 3.) We already see this unfolding: Bloomberg built a custom model, Salesforce finetuned OpenAI’s model to create EinsteinGPT, and Expedia has both integrated with ChatGPT (on its website) and is incorporating ChatGPT into its mobile app.
Leaders will also need to consider several components that can impact their implementation decisions: customer base and market advantage, high-quality data, talent, and computing power.
Customer Base and Market Advantage
Companies that choose the direct-to-consumer option (whether through building or fine-tuning) may not need to make significant operational changes, particularly if they already have strong market advantage and a large customer base. But for companies that choose to begin offering services through a third-party LLM solution, it’s important to closely track each platform’s progress in the market; to stay up to date on where their customer base is engaging; and to avoid lock-in, to be able to take advantage of changes in the platform pecking order.
LLM providers are currently competing over providing a superior user experience and securing first mover advantage to attract a critical mass of users that will appeal to companies. It’s not yet clear who will come out on top of the LLM platform play, but to protect their market advantage, companies should push for integrations to be portable between platforms, and advocate for advantageous revenue sharing, as well as data and IP protection agreements.
Data Quality
Unique, high-quality data is a primary source of competitive advantage in a generative AI world. LLM model functionality is largely driven by the quality and content of the data used to train it. Currently, the data used for these models is largely text, but in time, most models will become multi-modal, incorporating data from video, audio, financial transactions, and more. Whether providing a service through a third-party assistant or directly to the consumer, having the best data is the key to providing unique services and enhanced user experiences that attracts users.
Companies should consider all the forms of data they have at their disposal and be creative in how they use it, especially when choosing to create specialized models. Good data makes it easier directly to engage customers directly, reducing the risk of commoditization by a third party. In instances where a company with high-quality data chooses a third-party LLM platform, executives should be careful to participate in a manner that protects against commoditization.
BCG Henderson Institute Newsletter: Insights that are shaping business thinking.
Talent
Securing data talent is vital when choosing to build LLM-driven experiences in-house, even to make simple modifications to existing LLMs. The skills required to pre-train and fine-tune for specialized purposes are rare, even among data scientists. It doesn’t help that in the past year, more and more research on how to build or modify LLMs has become proprietary, and the available research is already starting to become outdated. That means that data scientists will need to be upskilled to work with these models, even as the public information available to upskill them potentially becomes limited.
Computing Power
Training and inferencing LLMs can technically be done using today’s CPUs and GPUs. But it will push this hardware to its capacity. At the same time, investing in upgraded hardware that is dedicated solely to training LLMs may not appeal to company leaders at first blush given the cost—it’s a high price tag for something that only happens occasionally.
But this perspective fails to account for the incremental costs of running the LLM, on the same hardware. Companies pay for every cycle that’s run in the cloud, and they pay for the energy costs of every cycle that’s run in their own data center. Customer-facing applications can end up with thousands to millions of queries per day—and a million queries can cost up to $120,000 in computing power. Leaders should consider making an upfront investment to avoid this snowball effect.
Executives will need to act quickly to set their generative AI engagement strategy. On the one hand, early engagement with LLM providers will better position traditional companies to set contracting terms such as revenue sharing and search optimization methods. In addition, engaging early may enable executives to influence the technology road map for their advantage—negotiating for technical features that will benefit their organization.
On the other hand, the open-source models that exist today are still quite good. But as research gets more proprietary, open source might fall behind. Companies that want a direct-to-consumer approach can’t wait for that to happen, or for other companies to launch solutions that could tempt customers to switch. Today’s inflection point will be tomorrow’s field of competition.