Every nation today needs to devise a robust artificial intelligence (AI) strategy. It is not about competing with AI giants like the US and China head-on, but about finding a specific and competitive foothold in this fast-evolving digital landscape where data is the new oil. AI, like Prometheus's gift of fire, is acting as the spine for a broad spectrum of ground-breaking technologies - from data analytics to robotics and the Internet of Things (IoT). Its influence is sweeping across vital sectors such as healthcare, education, energy, manufacturing, and transportation. We are just at the rupture of the AI revolution, with its full impact yet to materialize. It's like watching a thrilling mystery unfold - exciting, unpredictable, and full of opportunities. The recent global frenzy over generative AI models – the new rockstars in the tech world - underscores AI’s disruptive prowess and its potential to rewrite the art of the possible. Nations now stand at a strategic crossroads; a window of opportunity is open for them to make judicious choices about their positions in the AI race.
It's a high-stakes game, and the prizes for playing it well are monumental: enhanced GDP, increased productivity, job creation, improved quality of life, and citizen welfare. This endeavor is akin to a carefully orchestrated symphony - with each instrument, each note, representing key enablers and value creators of national AI strategies. A well-coordinated, strategic sequence could lead to exceptional results; a misaligned action, however, could undermine the overall strategic trajectory. Therefore, the call for action is urgent and should echo in every corner of national policy-making chambers.
Currently, the private sector has led the way in AI. The AI Index compiled by Stanford University reports that, in 2022, industry players released 32 of the 38 machine learning models that can be considered significant, while only 3 were released by academia
If the private sector has been the primary beneficiary of developments so far, a national AI strategy will pay dividends to the general public beyond protecting them from the risks highlighted above. On a practical level, governments can leverage AI to target resources and improve service delivery. If governments fall too far behind the private sector, citizens will grow more frustrated with the delivery of public services, and private actors will be exclusively setting the standards. While trends of the latter are starting to emerge globally , this imbalance risks prioritizing private interests without careful attention to the public good. More broadly, governments should drive the development of AI standards, norms, and priorities – nationally and globally – not relegate those decisions to the private sector. Importantly, they should do so proactively to keep pace with private sector-driven AI innovation.
ASPIRE: Framing a National AI Strategy
Countries approach AI from different starting points. They have different priorities for economic growth, social progress, educational achievement, and so on. They bring unique technological, engineering, and other strengths as well as limitations and weaknesses. They also take different approaches to balancing the interests of the government, private sector, and individual citizens. Understanding its starting point and approach to these trade-offs forms the basis for shaping a country’s strategy. In supporting national governments in their AI strategies, BCG has developed the “ASPIRE” framework which further defines and codifies six foundational elements: (1) Ambition, (2) Skills, (3) Policy & Regulation, (4) Investment, (5) Research & Innovation, and (6) Ecosystem.
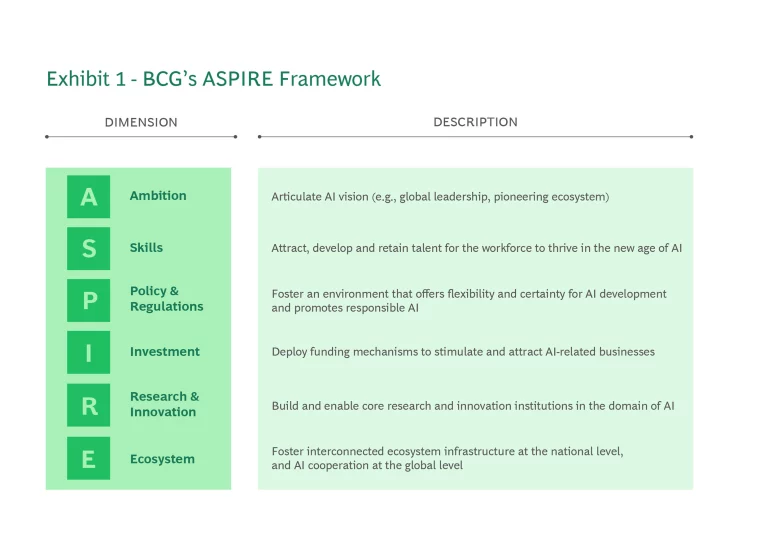
(1) Ambition
Ambition is the seed of strategy. It imagines what is possible, determines overall objectives, and directs the allocation of resources. In our review of approximately 50 existing national AI strategies, we identified three central archetypes:
- National Enabler. These countries promote AI to create and nurture local champions, improve their socio-economic condition, enhance the quality of life, and pursue other national objectives primarily by encouraging responsible AI and reskilling their workforce. The Nordic countries, small but highly educated, exemplify this approach.
- Specialist. These countries develop specific expertise that they promote globally. France and India are following variations of this approach, with France focusing on exportable R&D and India acting as an “AI garage,” providing outsourced services.
- Industry Leader. These countries aspire to be global leaders in a broad set of AI capabilities from R&D to implementation. This archetype demands a strong foundation, including advanced R&D, cloud infrastructure, large data sets, and a proven record of commercialization. In addition to China and the US, the UK and South Korea are vying to break into this realm.
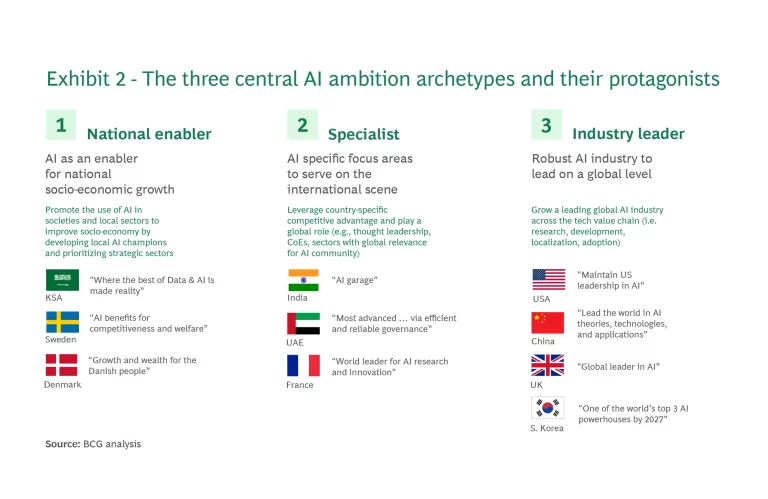
Most countries fit into one of these archetypes, although strategic details and progress along their chosen AI paths vary substantially.
One such variation lies in the economic sectors each country will select as priority beneficiaries of AI’s transformative power. This prioritization does not rule out AI adoption across all sectors; rather, it entails a strategic allocation of resources towards high-potential sectors – often 5 to 7. Three criteria can guide this decision and ensure the concentration of efforts is optimized:
- The level of proven data & AI impact globally in the sector
- The alignment of the sector with national priorities
- The readiness of the sector to adopt AI
By employing this approach, countries ensure their AI strategy contributes to sectors of national importance and where AI could be meaningfully utilized to generate impact.
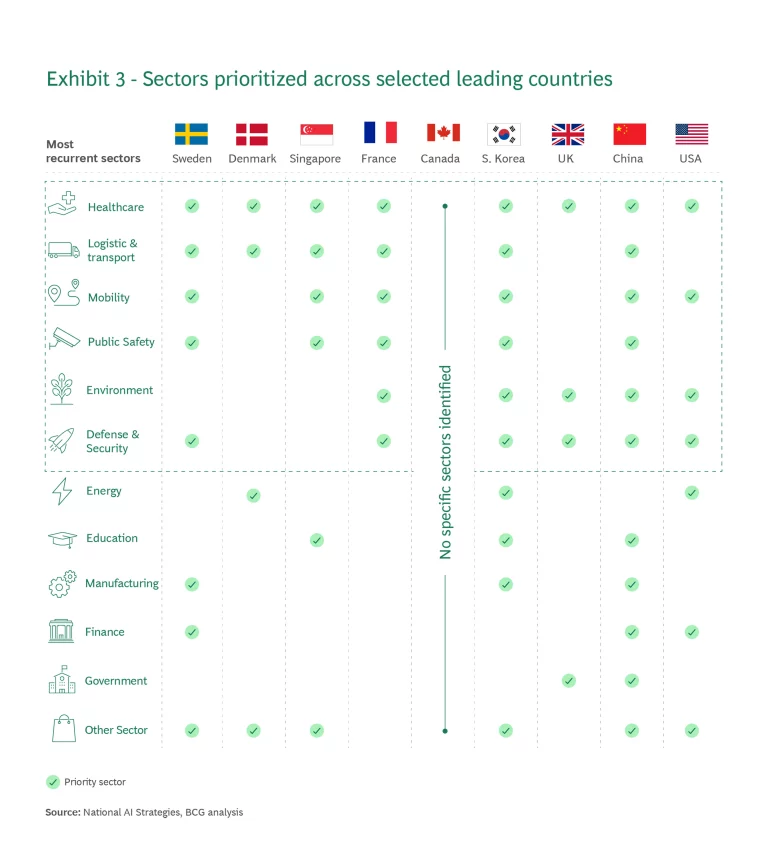
(2) Skills
A strategy is only as effective as the people possessing the proper skills to execute it. A national AI strategy should outline an approach to building the skill base through education, reskilling, and training. But developing and keeping talent with the necessary AI skills is a global challenge. Countries must deal with:
- Limited knowledge of data and AI, including basic literacy, slowing ecosystem-wide adoption. For instance, 67% of leaders globally say their company is exploring ways of leveraging GenAI, yet 66% of them report that their employees do not have the skills to use the technology successfully
2 2 Salesforce (2023): New Study Reveals Only 1 in 10 Global Workers Have In-Demand AI Skills . Key levers for addressing this systemic issue include enhancing access, motivation, and talent development. Importantly, governments and academic institutions around the world are embedding data and AI into their formal curriculums (over 1,000 master’s degrees in AI alone and over 1,800 in data science and big data)3 3 Based on Mastersportal (AI and Data Science & Big Data sub-disciplines), retrieved in July 2023 . Higher education institutions are also adapting their non-technical degrees to develop AI talent in sectors: degrees combining data science or AI with the likes of business administration or social science are increasingly popular, as well as compulsory electives for students as illustrated by Saudi University KFUPM’s AI+ curriculum embedding mandatory AI modules within engineering programs. Efforts are not limited to higher education: UNESCO identified 11 countries that have endorsed and implemented government-led K-12 AI curricula, which include Austria, China, India, and the UAE4 4 UNESCO “ K-12 AI curricula: a mapping of government-endorsed AI curricula” (2022) . In addition, a growing number of talent development initiatives are being conducted outside formal education systems, with countries embracing the full range of development opportunities besides universities, including training programs, on-the-job training, and self-learning. We see countries like the UK, Singapore, and the UAE implementing initiatives to build basic AI literacy in both students and adults. Some countries have expanded their initiatives beyond national borders. Finland, for example, provides free online basic literacy courses for anyone globally with the goal "to demystify AI". Its program has been accessed in 170 countries, and 40% of its students are women5 5 Elements of AI: Elements of AI . - A global shifting labor market, posing over 85 million jobs for displacement by AI and machinery by 2025
6 6 World Economic Forum (WEF) “Future of Jobs Report” (2020): The Future of Jobs Report 2020 . Countries need to raise awareness of AI and related job opportunities and develop talent through reskilling and on-the-job training. Data and AI are the world's fastest-growing job categories, with 97 million new roles expected by 2025, exceeding the number of those displaced. It is critical for countries to push retraining and transition to accommodate these shifts and incentivize the private sector to participate in the effort. - A global supply-demand talent gap. Around the world, demand for AI talent is growing faster than supply, and more than 50% is located in just three countries (USA, China, and India)
7 7 Everest Group (2022): Global Data, Analytics, and AI Talent Locations | Market Insights . Beyond local initiatives, most countries will also need to attract foreign talent, including through dedicated programs like Singapore’s Tech@SG. It is essential to recognize that this talent extends beyond the technology itself. An often-overlooked trait is the ability to bridge the worlds of deep technology and business. People who can translate technology into terms that business executives can understand, and convert business challenges into technology solutions, remain a rare breed. - Global competition for leadership driving talent availability and retention challenges. Countries should focus on attracting AI talent in the context of their integrated global innovation and recognition efforts, with many using physical employment opportunities as a starting point to build their talent pools. Building a sustainable ecosystem, for example, through work flexibility and immigration policy, is key to integrating overseas talent into the local economy. Meanwhile, encouraging locally driven innovation, including through startup support, builds worldwide recognition of a country’s thriving data and AI sector. This, in turn, makes it easier to attract talent.
(3) Policy & Regulation
Regulating AI has come into focus as deployed AI systems have put users, businesses, and government at risk. Both businesses and governments have a role to play.
- Companies are starting to self-regulate through responsible AI, hoping to create a competitive advantage. But the same study found that just 16% of the companies surveyed had mature responsible AI (RAI) programs . Of course, businesses put varying degrees of effort and intent behind ensuring the responsibility of their AI efforts (a point reinforced by recent turnover among some leading names’ AI Ethics teams).
- The central importance of governments in creating an overall national strategy, and designing AI legislation to orchestrate public, individual, and private interests is hard to overstate. From a state perspective, taking actions such as controlling cross-border data flow is key to protecting national security and interests –a priority for China. China’s recent Measures for Generative Artificial Intelligence draft is a good illustration of this priority with requirements such as the alignment of GenAI systems’ output with national values. Meanwhile, safeguarding citizen interests requires a focus on personal data protection and security. This has been a long-standing focus for the European Union firstly embedded in the General Data Protection Regulation (GDPR) and more recently in the EU AI Act, the world’s first comprehensive AI law. The Act addresses the ethical implications of AI: it defines a classification of AI systems into four risk categories, each associated with a set of rules and restrictions, including requirements around data quality, transparency, oversight, and accountability. This Act not only covers specific use cases such as facial recognition, which falls into the highest risk category and is effectively prohibited by the Act but also general-purpose models such as large language models. Finally, the private sector will benefit most from light-touch legislation favoring open data and prioritizing data value realization – as we see in the USA, although state-level legislation inspired by GDPR is on the rise (e.g., the California Consumer Privacy Act). Take data privacy as an example, as data is the raw material for AI systems. If data privacy laws are too lax, the public will rightly question whether AI’s value outweighs its risks. Some estimates indicate that up to 2.5% of GDP
8 8 OECD: Data Governance can be unlocked by relaxing data-sharing limitations and encouraging access to data, but tapping into this socio-economic potential requires clear legislation fostering data as a national asset. Countries have addressed the challenge differently. Some, like China, take a highly restrictive approach to data regulation and cross-border data flow. On the other end of the spectrum, countries like the US and Canada employ a light-touch approach and encourage free data flows. The European Union and Singapore, among others, have crafted their models leveraging selected restrictions to balance interests across data economy stakeholders. If AI ethics and data privacy have often been cited as some of the most complex and critical areas of legislation, recent developments in GenAI are also shedding light on the issue of intellectual property rights. - Countries have created various governmental bodies to deal with data, establish a supportive but watchful regulatory environment, and create rapport with the private sector. Some, like Saudi Arabia, have highly centralized government authorities. Others, like the US, are much more decentralized with the implementation of broad initiatives delegated to several agencies. The UK positions itself in the middle ground, as highlighted in the 2023 government whitepaper “A pro-innovation approach to AI regulation”. There the government sets a national framework and principles, and while the Office for AI plays an oversight role, it leaves its implementation to sectoral regulators. Beyond data itself, these bodies also need to tackle the technologies that will be creating value from it and address increasingly pressing challenges. Are governments actively setting rules, standards, norms, and principles to foster responsible AI? Are they ensuring that algorithms being used in the country are understandable, auditable, and do not present risks for users including harm and discrimination? Are they conducting appropriate audits and other forms of oversight?
Overall, regulating AI – whether by simply setting core ethics principles or through proactively introducing protective mechanisms – is necessary to ensure sustainable development of the AI innovation ecosystem, maximization of value from AI solutions, and protection of citizens from harm, undesired use of AI, and discrimination. Both private companies and government have roles to play in developing principles and ethics around AI. UNESCO developed recommendations on the AI ethics focused on human rights and inclusiveness, and was adopted by all 193 Member States. Regulating a fast-changing field like AI is an ongoing process. Leading countries have typically started their journey with soft legislation focused on principles to guide AI players and enable rapid growth of the data and AI economy. Then as understanding and applications become more sophisticated, mature jurisdictions like the US, EU, and China have moved toward more restrictive models coupled with pro-innovation initiatives like dedicated sandboxes (low-risk high support environments setup by government entities to fuel cross-sector innovation.).
Recent disruptions, like the suddenly accelerated adoption of GenAI systems, have triggered different reactions worldwide. While certain countries, like Italy, have chosen a temporary ban as a precautionary measure, others, like Portugal, have swiftly seized the opportunity to integrate this technology into their government services. In times of fast-paced innovation, governments often find themselves in a reactive stance towards private sector advancements. A strong national AI strategy can equip policymakers and legislators with directions, principles, and guidance on key trade-offs that can promote efficient and harmonized decision-making in the face of rapid technological advancements.
(4) Investment
A nation’s willingness to invest in AI needs to match its ambition.
- National governments have allocated extensive budgets for AI development. Examples include China, France, South Korea, the UK, and the US which have each announced and committed to investing more than USD 1 billion in AI, leaving other nations far behind. Governments, for example, can stimulate investment activity through supportive fiscal and regulatory mechanisms. This help establishes a culture that incentivizes support for data and AI ecosystem growth and attracts international and domestic interest. Leading nations utilize diverse funding vehicles and structured investment support to activate their data and AI ecosystems. Funding vehicles include debt (e.g., loads, credit lines, etc.), equity, and direct spending. Collectively, these instruments span the entire value chain from pre-seed to buyout / later-stage funding, ensuring a healthy ongoing financial pipeline for ecosystem participants.
- Technology companies’ debt ratios have been rising (more than 100% over the last year for European technology startups)
9 9 Bloomberg (2023): Europe Tech Startups Doubled Debt Financing in Fundraising Shift showing an increased appetite for debt vehicles, including startup loans, project investment loans, microfinancing, and credit guarantees which provide countries the opportunity to activate their data and AI sector. They are facilitated by multiple providers, including banks and financial institutions, government lending, and NGOs, as well as non-financial private institutions (specifically in the USA). Countries have implemented specific initiatives using targeted debt vehicles to develop data & AI with a specific focus on company size (e.g., Microfinance Ireland, which provides short-term loans to startups), on a mandate, on industries, or location.
It is important to note that investment support extends well beyond the monetary. It starts with attracting businesses and funders through fiscal, financial, and regulatory incentives, local market information access, and ecosystem promotion. Countries can also increase the ease of starting businesses, and establish investment protections to create a pro-investment climate. They can offer business advisory and legal support. Finally, they should facilitate connections among stakeholders, building a pro-partnership environment, and developing clear paths for investors and efficient approaches to match-making.
- Equity funding has also continued growing in the past years, despite a recent slowdown. Between 2013 and 2022, corporate investments in AI globally grew by a factor of 13, reaching an all-time high in 2021 with approximately USD 275B invested
10 10 Stanford University HAI "AI Index Report 2023": Measuring Trends in Artificial Intelligence (this includes M&A, minority stake, private investments, and public offerings) . Recent AI advancements such as GenAI are expected to fuel further investments, with over USD 12B worth of deals announced or completed in the first quarter of 202311 11 Pitchbook (2023): Generative AI Startups Jockey for VC Dollars . Notably, large technology companies have been pledging investments in GenAI startups: it is the case of Salesforce which is doubling the size of its Generative AI Fund from USD 250M to 500M12 12 Salesforce (2023): Salesforce Ventures Doubles Down on Generative AI Fund . Equity investments are sourced from both public and private entities with levels varying by country. The USA and UK are mostly private sectors driven: in 2022, the USA was leading private investments in AI worldwide with approximately USD 47B invested by private actors. China and Singapore receive the most public contributions, including through sovereign funds and government Venture Capitals (VCs). Countries like France and Germany employ a hybrid model, including co-investment initiatives and government investments in VCs. - Leading countries also provide direct funding including growth and innovation grants, seed and startup support, as well as individual and employee support such as for hiring and training. These can be complemented by incentives for businesses focusing on tax breaks, subsidies, and special economic zones: Singapore for instance provides tax exemptions of up to 100% to qualifying R&D projects. Finally, ecosystem growth can be facilitated by national budgets allocated to specific ministries as illustrated by the Canadian national AI strategy which allowed direct funding of 3 national AI centers of excellence.
- It is important to note that investment support extends well beyond the monetary. It starts with attracting businesses and funders through fiscal, financial, and regulatory incentives, local market information access, and ecosystem promotion. Countries can also increase the ease of starting businesses and establish investment protections which are two of the primary decision factors for investors when gauging if a country has a pro-investment climate. Offering business advisory and legal support is also common across leading countries including Germany, South Korea, Canada, and Singapore. Finally, they should facilitate connections among stakeholders, build a pro-partnership environment, and develop clear paths for investors and efficient approaches to match-making. Government-supported hubs, such as Hub71 in the UAE and Station F in France, provide a platform for all relevant stakeholders, matching funding entities with ventures, among others.
(5) Research & Innovation
Governments can also deploy their R&D budget and agenda in support of AI by targeting areas that play to the nation’s strengths or are critical to addressing its most pressing challenges. A nation’s ambition archetype – national enabler, specialist, or industry leader – will largely shape the scope and priorities of its AI R&D agenda. It will also guide the respective roles of government, academia, and private players, and how their contributions are orchestrated. There is no one-size-fits-all for national priority-setting. Leading countries follow diverse approaches. Some, like Switzerland, are already leaders in general R&D, so include AI organically in auxiliary national strategies. Others, like Singapore, have a dedicated AI strategy with a strong R&D focus. Finally, countries like the US make AI R&D a specific priority domain with its own extensive strategy, focusing on moonshot initiatives and requiring heavy investments.
Generally, countries should focus on supporting basic research, public-private partnership, and collaboration with global AI organizations.
- Specialist governmental bodies should go a step further in creating a research and innovation infrastructure by developing centers for basic and applied research, investing in technology infrastructure, and strengthening collaboration between industry and academia.
- Meanwhile, industry leaders should invest heavily in applied research, integrating innovation and industrialization, and commercializing innovation in advanced sectors. Regardless of a country’s ambition, collaboration between the public and private sectors and between companies and universities is key.
Typically the public sector can kick-start R&D by creating demand for AI innovation and providing financing. Academia is responsible for research, knowledge transfer, talent development, and international stakeholder attraction. The private sector develops its own research, fosters collaborations, funds R&D, and captures value. The orchestration of these stakeholders takes different forms across leading countries. Some favor a self-orchestrating ecosystem, others delegate organization to area-specific champions. All leading countries, however, seek ways to proactively develop their ecosystems throughout the AI R&D cycle.
(6) Ecosystem
International Ecosystems: Global Cooperation Efforts
Despite countries competing to become global AI leaders, cooperation between one another has never been more critical to address the global challenges raised by AI and better capitalize on its opportunities. As such, AI cooperation has become a key enabler for countries around the world.
- The ethical implications of AI, ranging from data privacy and algorithmic bias to autonomous decision-making, transcend national borders: effectively addressing them demands close international cooperation. This priority was reflected by recent efforts from international organizations and intergovernmental forums which constitute critical alignment platforms for countries to jointly address AI ethic challenges and develop shared principles, standards, and frameworks to be implemented at the national level. For instance, UNESCO published its "Recommendation on the Ethics of Artificial Intelligence" in 2021, which defines principles and policies guiding AI policy-making worldwide. Similarly, the OECD established its AI Policy Observatory and published AI recommendations that served as a basis for the G20 AI Principles adopted in 2019.
- Cooperation also underpins the economic potential of AI. By fostering AI cooperation, countries can facilitate the development of interoperable AI systems, harmonize regulatory frameworks, and promote open data sharing. Collaborative initiatives that foster AI cooperation can create a level playing field, enhance market access, and promote fair trade practices, thereby unlocking new avenues for economic collaboration and driving innovation across borders. To capitalize on its economic potential, countries are now specifically embedding AI in digital trade agreements (e.g., UK-Singapore Digital Economic Agreement).
- As a multi-purpose technology, AI has the potential to help address key global challenges such as climate change, poverty, and public health. Effectively leveraging AI to address these issues requires close international cooperation to enable the pooling of resources and expertise, knowledge transfers, and data sharing. International organizations can again provide an important platform to foster such cooperation; it is the case of the ITU’s AI for Good forum focused on identifying innovative AI solutions to advance the Sustainable Development Goals (SDGs). However, moving from dialogue to activation will require close involvement of academic, private, and non-profit players.
Local Ecosystems: Fulfilling Infrastructure Needs
Beyond international cooperation, the presence of a local ecosystem adjacent to AI is crucial. Many of the most effective AI applications are not stand-alone but rather depend on an interconnected ecosystem infrastructure. Self-driving cars, for example, have built-in sensors and processing units, cameras, and wireless connectivity built by separate companies that must work seamlessly together. These ecosystems vary in complexity, depending on a nation’s ambitions, but will all involve startup development, industrial adoption, infrastructure, data access, and government support. As with research and innovation, collaboration is the cement that holds ecosystems together.
In addition to cross-cutting ecosystem infrastructure providing security and interoperability, the following three overarching layers of infrastructure are particularly important in driving leading countries’ AI success:
- Computing and Cloud: Sophisticated data and AI projects require scalable cloud infrastructure with hyperscalers. But global hyperscale cloud resources are failing to meet the growing demand. To address this, leading nations are establishing cloud hubs and expanding the presence of hyperscale cloud providers in the country to run the data and AI workload locally. For example, the Dublin Cloud Computing Hub hosts 70 data centers operated by hyperscale
13 13 University College Cork (UCC): Data Centres: A View from Europe cloud providers, with state-of-the-art IT infrastructure, fiber optic speed networks, and advanced technology telecommunications. - Data: Limited data accessibility and low data quality are key challenges in data and AI enablement. Today, approximately 45% of Smart City applications require cross-industry consolidated data, and organizations lose on average USD 15 million annually due to poor data management
14 14 Gartner: How to Create a Business Case for Data Quality Improvement . Countries can address this by providing open access to national data platforms, consolidating sector-specific data, developing standardized specifications and guidelines to enhance data quality and classification, and providing toolkits to entities on their data journey. An example is Singapore’s Data.gov.sg, a one-stop portal including big data from 70 government agencies. This data ranges from raw (e.g., list of taxis in a city) to high-level statistics (e.g., public transport stats). - Data and AI Application Enablement: Sophisticated data and AI projects require automation and acceleration tools. Countries can address this by advancing sectoral analytics capabilities, consolidating AI tool repositories, and leveraging best-in-class automated platforms. The US Navy, for example, collaborated with Google AutoML1 on a 6-month effort to leverage their AI tools, accelerating the development of a corrosion detection platform with 90% accuracy
15 15 Google Cloud: Five Tips for Prioritizing Investments in Government Technology .
From Aspiration to Action
A strategy is only as good as its execution. How then can countries put a national AI strategy into action? Our recommendation is to start with four concrete steps before engaging with the specific dimensions of the ASPIRE framework.
First and foremost, governments need to establish a clear and dedicated ownership structure that possesses the authority and capacity to lead the national AI strategy. This entity could be a central coordinating body, a specialized department, or an empowered agency for instance. Its mandate includes mobilizing the entire government machinery and ensuring the necessary technical expertise to support the strategy's development.
Secondly, the designated entity should foster collaboration and engagement with a wide range of local stakeholder groups: technology, communications, labor, education, financial, and economic development, as well as key national sectors, who will be involved in the drafting of the strategy to ensure alignment at the national level.
This wider group of stakeholders is key to the third step. For the strategy to reflect a country’s broader societal aspirations, it must be inclusive. To achieve this and ensure special interests do not have an undue influence, consultations must be made a priority, involving academia, businesses, civil society organizations, and experts from law, sociology, and other adjacent fields. Consultations should also go beyond national borders, capturing inputs from international experts, leading countries, and prospective partners, and aligning the strategy with global trends and standards.
Lastly, as the strategy reaches maturity, awareness will become a priority both at the local level, ensuring all parties understand not only the aspirations of their country but importantly how they will benefit from these efforts and what role they will play. Such awareness should also be raised at the international level to promote the country’s agenda, accelerate participation in global AI cooperation efforts, and establish the country as “open for business” when it comes to data & AI. Furthermore, given that AI is a rapidly evolving technology, it’s paramount for governments to possess the agility to periodically revisit and refine their national AI strategies. As advancements occur and more insights are garnered, updating the strategy becomes pivotal to stay relevant to the latest global trends, build upon earlier accomplishments, and refine the nation’s unique value propositions and strengths in this space.
These steps constitute an integral part of the strategy activation journey and will be critical to making AI aspirations a reality. As emphasized across this piece, the public sector can play a catalytic role by providing the necessary ingredients for success. While national governments can create conducive innovation environments, it’s the proactive collaboration with the private sector and the broader stakeholder map that will drive AI to flourish responsibly in the 2020s and beyond.