As the world strives for a sustainable future, no industry is more in the crosshairs than oil and gas. Every new project is scrutinized and more difficult to get underway than the next, so it’s critical that O&G companies maximize value from existing operations. One clear opportunity to manage this feat is to do more with the mountains of data that O&G companies have at their disposal by applying emerging analytics and AI capabilities to unlock new sources of value and cost savings.
Harnessing data to improve efficiencies and unlock value requires transformative change and close coordination between the business side and IT—two groups that do not always work together well. Focusing on data and digital innovation as well as on cross-functional collaboration could also help attract young, tech-savvy talent looking for an innovative and inspiring work environment.
Finding the Value
To align the business and IT, we recommend that both sides jointly define a North Star for their data and digital architecture. Key to this exercise is the creation of a data trinity : the data use cases that are important to the business, the data assets that enable those use cases, and the architecture that makes the data assets accessible. Proper prioritization of high-value use cases and data projects shapes the architecture modernization initiatives necessary to implement them.
This is a complex exercise, but turning to data and AI to unlock value is critical and real examples from the O&G industry do exist. Moreover, BCG analysis shows that investors recognize data as a rich vein of potential profits and cost savings. For example, HSBC has stated that “the opportunity for the digital oilfield to have a major impact across most parts of the value chain is significant.” Barclays estimates that digital could lower the cost of production by more than $3 per barrel, amounting to about $150 billion in value. And the World Economic Forum estimates that “operations optimization” could unlock as much as $275 billion in value, with 90% of it coming from optimizing drilling and production and from leveraging the data from end-to-end connected assets to feed advanced analytics algorithms.
Subscribe to our Digital, Technology, and Data E-Alert.
Choosing Data Use Cases
The first step is selecting the data use cases that are most important to the business. High-value use cases cover a range of value pools and methods that address many of the challenges that O&G companies face today, such as the following:
- Applying AI based on existing data (such as in exploration, reservoir modeling, or drilling activities) to improve exploration success rates, maintain asset yields, or reduce cost
- Improving workforce effectiveness and reducing risks with, for example, remote and unmanned operations and monitoring or better resource planning and scheduling
- Reducing carbon footprints (such as by optimizing refining or transportation)
- Leveraging industry networks by, for example, collaborating online for engineering, procurement, or construction
- Streamlining labor-intensive processes, whether in administration (such as by using e-procurement) or smart automation of order processing
What these use cases all have in common is that they depend on data from a variety of processes—many of them AI enabled. Mastering that data gives O&G companies a “right to win” in today’s marketplace. Recently, we worked on cases that illustrate this well:
- A Middle Eastern company combining geological and engineering data into an optimized, automated drilling sequence that avoids collisions with existing wellbores
- A major European O&G company using an AI engine to optimize the production schedule based on a broad variety of data, maximizing returns from the company’s production assets
- A major integrated oil company leveraging smart maintenance with a combination of equipment, operations, and maintenance data, drastically reducing maintenance budgets and downtime
Successful implementation of data-driven use cases requires reimagining the end-to-end value chain with data and digital technologies. Creating a joint business and IT view of the value chain shows where key business capabilities reside and helps clarify which use cases are enabled by those capabilities. Developing strong, reusable, and shared capabilities helps launch successful use cases.
Unlocking Key Data Assets
Based on this initial assessment, companies must identify the specific data assets needed to fuel their use cases. (See Exhibit 1.) Success ultimately depends on unlocking existing data assets along the O&G value chain and then applying analytics and AI within a consistent, robust, and scalable architecture.
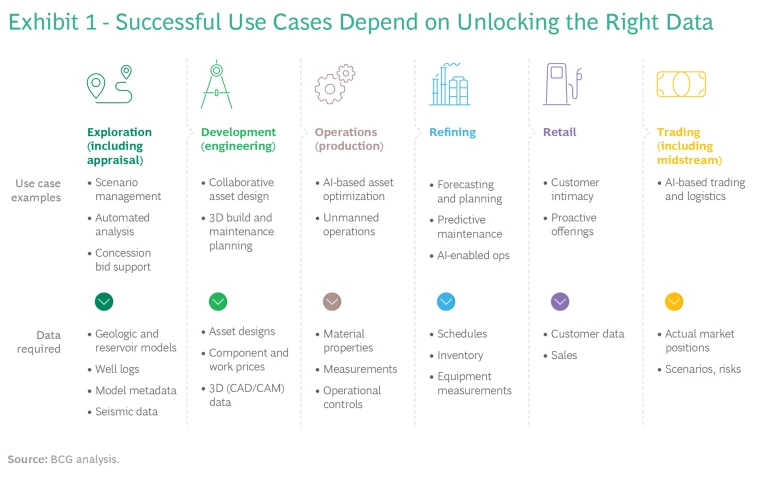
Then—and this is critical—companies need to plan the rollout of use cases so that, to the extent possible, the first wave leverages and improves data assets in a way that will benefit subsequent use cases, accelerating their deployment and business impact over time.
To unlock their data assets, companies must manage eight data challenges that are largely specific to the industry:
- Variety of Data. O&G data ranges from the very technical (such as drilling, log, seismic, lab, and sensor data) to complex models (reservoir models and wellbore shapes, for example) to 3D engineering designs to logistics (regarding people and equipment as well as hydrocarbons). All of this on top of the business’s “normal” transactional or master data.
- Proprietary or Inaccessible Formats. Data comes in proprietary sensor- or system-defined formats (such as 3D geological and reservoir models) that require conversion and might not be accessible through direct means. Similarly, engineering design data tends to be quite specific to the software that generated it.
- Dataset Sizes and Volumes. Datasets tend to be huge—up to petabytes for a single seismic survey. This stretches the capabilities of even cloud service providers.
- Scarcity and Interpretation. Technical data is often limited, incomplete, and even inconsistent, making it subject to interpretation. There is not always a “single source of truth.”
- Stakeholder Requirements. Governments, regulators, subcontractors, and joint venture partners all provide and use data. Often they have specific contractual commitments (such as export constraints and confidentiality guidelines) regarding what they can share and how. These requirements must be properly managed.
- Ambiguous Definitions. Internal and external organizations apply different data definitions and standards, impeding reuse and sharing of datasets. Uniform definitions are the goal, but reliable metadata to facilitate interpretation is the second-best option.
- Organizational Complexity. Typical O&G companies have decentralized structures with local joint ventures. The latter often have IT priorities that compete with central IT directives. If not resolved, these competing priorities could compromise data ownership and the ability to use data.
- Location and Geography. Data is often generated and used in locations with little bandwidth, preventing O&G companies from using cloud technology effectively and requiring smart replication methods.
Governing the Data and Updating the Architecture
These challenges require a mature approach to data governance covering the life cycle of different data types and addressing the constraints and complexities intrinsic to each one. Governance should be based on a system-agnostic view built on a single, company-wide categorization of all data into “data domains.” The level of detail may be different for different companies, reflecting variations in organizational levels and functional ownership. Data domains are the basis for any initiative regarding data definition, data quality, data access, or data-specific business rules, including concerns such as data privacy and confidentiality. Exhibit 2 shows an illustrative subset of a data domain map.
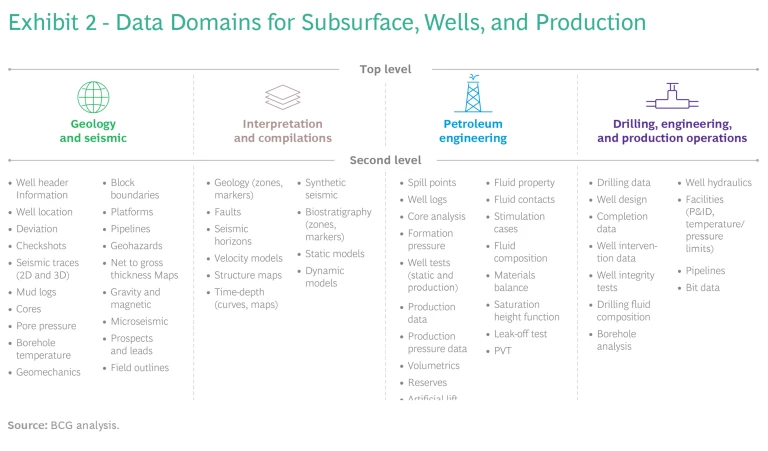
Next, O&G companies must move from a monolithic to a modular technology stack in order to free up their data and take advantage of new technologies. A data and digital platform lowers costs and improves speed and agility by decoupling front-end capabilities from data, liberating data from core transactional systems, and enabling smart-business engines with machine-learning and AI capabilities.
With this goal of a decoupled architecture in mind, O&G companies need to build a reference architecture to access and use the data assets. As with the data use cases, companies must prioritize and sequence their architecture modernization initiatives so that the first wave of improvements provides a foundation for the upgrades necessary to enable future use cases. Ultimately, O&G leaders must build a data platform that can scale horizontally to leverage the data sources in a consistent way across a variety of cases and potentially complex local IT landscapes. Horizontal scaling combined with a multicloud approach is necessary in order to cope with the huge amount of data and to allow geographically dispersed teams to collaborate effectively.
Successful Implementation
The final challenge is to get the execution right. Digital is, to a large degree, a change management effort. As a rule of thumb, typically 10% of success depends on architecture, 20% on data, and 70% on change management. There must be an integrated, multidisciplinary effort across digital app development, data governance, and architecture and operating model changes. To realize value at scale, digital needs to enable fundamental changes in how people work and how decisions are made in new end-to-end workflows. Starting with a few powerful lighthouse use cases—with well-defined value potential and a high degree of management support—is a tested way to move forward and ensure organizational learning and buildup of momentum. This, in the end, is what ensures that value is actually delivered.
O&G companies are currently reaping large profits from their operations, but it’s important that they innovate to stay relevant and competitive in a rapidly changing economic environment. They will need to become much more data driven, leveraging data and AI to drive high-value use cases. But successfully leveraging data is a complex effort that cannot be done by IT alone. It is a major change to how companies work. Setting up the basic data governance, defining a new data and digital architecture, and driving the first use case pilots require significant time, effort, and commitment.