Imaginative use cases, rapid technological progress, and boardroom drama have dominated the headlines about generative artificial intelligence (GenAI) since the launch of ChatGPT in November 2022. Largely absent from these conversations, however, is a discussion about how software companies and GenAI application developers should set the right pricing strategy and pricing models, two critical elements that determine the long-term trajectory of any new technology.
The strategic pricing decisions that companies make today will have far-reaching effects that will determine how quickly the adoption of GenAI accelerates, who benefits from it, how much money organizations can reinvest into improvements and competitive advantages, and even the future of human-machine interaction. The less consideration companies devote to pricing strategy and pricing models, the greater the risk that they will artificially limit the potential of their solutions by discouraging customers from experimenting. This will not only limit the reach and network effects that would make those solutions more valuable but will also leave open the door for new entrants with better pricing options.
The less consideration companies devote to pricing strategy and pricing models, the greater the risk that they will artificially limit the potential of their solutions by discouraging customers from experimenting.
Today’s GenAI Pricing Is More Expedient Than Strategic
The pressure to launch a solution compelled many innovators to pick a model and a number quickly for their pricing. As a result, even the most experimental companies have tended either to forgo monetizing their GenAI offers for the time being or have defaulted to one of two simple, familiar pricing models. The first is a token-based pricing model that charges users for consumption and is loosely aligned with the computing power needed. The second is a subscription model that charges a uniform fee per user per month but raises concerns that the underlying costs of computing power will make the offerings unprofitable for the vendor.
We recommend companies take a more strategic approach to GenAI pricing that starts with a thorough understanding of the three information sources for any major pricing decisions: customer value created, the competitive landscape, and the economics of the product or service. An integrated view of those sources gives firms the foundation to choose the pricing model that best reflects their short- and long-term objectives and a pricing architecture that captures and shares value in line with their broader business strategy.
The Strategic Approach to Pricing for GenAI
Choosing the most expedient pricing model ignores the reality that GenAI applications are fundamentally different from many other technologies, thanks to their sheer potential for value creation and their evolving ecosystem. Those differences underscore the need for the vendor to think through the following three questions carefully.
How do you create and share customer value? The exponential growth in the adoption of ChatGPT and other GenAI applications shows that it is currently impossible to define the upper limits on the technology’s potential value. While direct value to customers can vary widely, there are six key value drivers that we have observed in the evolving GenAI ecosystem (see Exhibit 1):
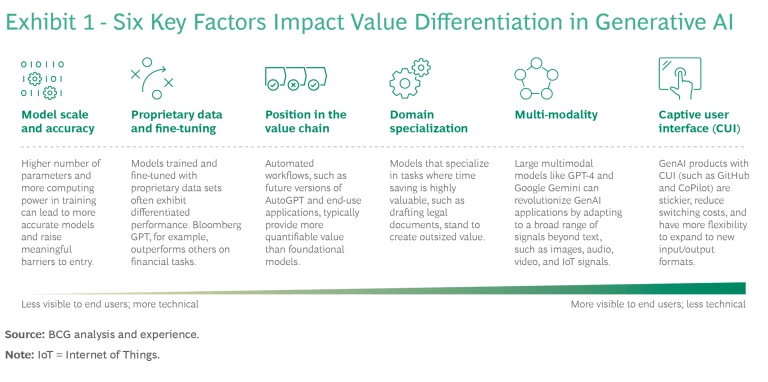
- Model scale and accuracy
- Proprietary data and fine-tuning
- Position in the value chain
- Domain specialization
- Multi-modality
- Use of captive user interface (CUI)
Model scale and model accuracy are common and well-known technical indicators of value, while the other value drivers are less technical and should be more visible to customers. A company can feed proprietary data into a GenAI-powered application to improve accuracy and create unique outcomes. For example, Bloomberg reportedly improved the accuracy of its GPT model for finance-specific tasks by up to a factor of four when it incorporated proprietary content.
The major differences between the foundation models, the GenAI-powered applications, and the automation workflows are the range of data that trains the models, the tasks that each application can accomplish, and the degree of human involvement. The foundation models and GenAI-powered applications either augment people’s jobs or free them up for higher-value tasks, while the automation workflows require little or no human supervision.
How differentiated are you within your competitive landscape? The competitive landscape for GenAI is rapidly evolving, too. (See Exhibit 2.) Foundation models, including large language models (LLMs), first garnered the most attention. A few big competitors—early movers like Open AI and hyperscalers like Google (Bard, Gemini), Meta (Llama), and Anthropic (Claude)—will determine the landscape for those large-scale, general-purpose models, in part because they require billions of dollars to train, refine, and operate.
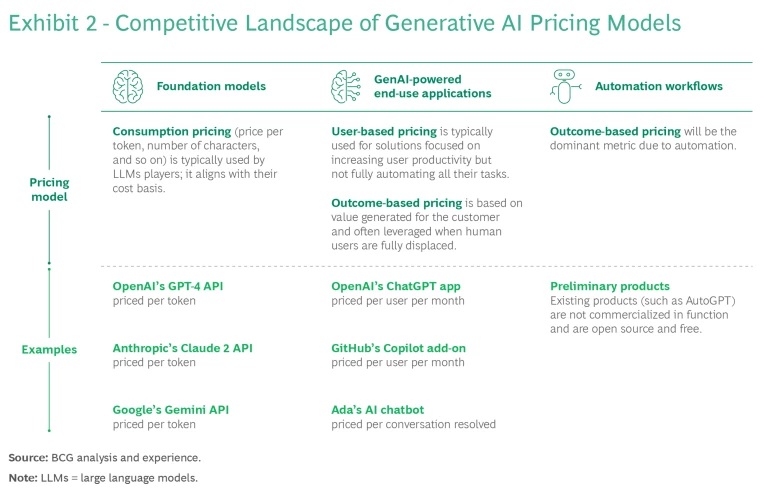
As new and existing technology players enter the market with their own GenAI offers, the landscape is becoming more fragmented in terms of both smaller models and more specialized applications and workflows. Smaller providers have an opportunity to differentiate themselves, provided they either have highly specialized capabilities or can segment their offerings to serve a diverse and fragmented customer base. Mistral AI’s 8x7B model, released in December 2023, beats GPT-3.5 on most benchmarks, although it is much smaller than OpenAI’s models.
How will your costs scale and evolve? The economics of GenAI solutions remain uncertain as the costs continue to evolve. By contrast with software and software as a service (SaaS) applications, whose marginal costs can be very low, the underlying cost to serve of GenAI models can be quite high, depending on the use case and pricing model. By some estimates, a high share of the revenue that Open AI has recently generated has gone toward covering computing costs driven up by a shortage of the GPUs needed to support the models. (See Exhibit 3.)
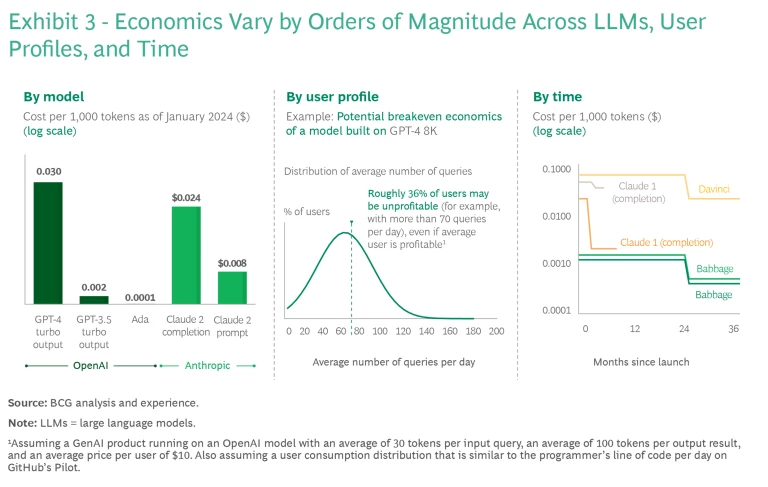
If a company charges a flat rate for access to an application and the costs scale primarily with the number of queries, then the distribution and cost scaling of queries per user is a major driver of profitability. A large share of heavy users can therefore make the model unprofitable in the near term. Strategic pricing decisions, however, not only require an understanding of current unit costs but also how those costs will scale and change over time. As Exhibit 3 shows, the costs per token for various models have declined. Those expenses are plotted on a logarithmic scale, which means that costs for lower performance models are exponentially lower, both in general and over time. There are multiple forces pushing unit costs down, including Moore’s Law, Huang’s Law, improvements in model training, and investments in accelerators. At the same time, new capabilities tend to result in new use cases that may consume more and higher-cost resources. A deep understanding of the tensions across these forces is essential to the economic lens when making pricing decisions.
There is no one-size-fits-all pricing strategy for GenAI. A model developer facing these economics will instead have several options. They could raise the price per user, keep the current price if they believe costs will decline significantly, redesign to offer a lower-cost model that meets a target price point, or adjust the price in a way that incentivizes customers to align their behavior with costs. Which option is right will depend on the market context (including the customer value and competitive landscape) and the vendor’s business strategy.
Choosing the Right Pricing Model for a GenAI Strategy
The pricing model shapes how a company monetizes and shares the value it creates. The most important factors in setting a model for a GenAI application are the pricing basis (the unit and timing of the price) and the offer architecture (how to package the innovations).
The pricing model shapes how a company monetizes and shares the value it creates.
Two predominant pricing bases have emerged for GenAI so far: consumption, which is usually measured per thousand tokens used, and subscription, which is usually measured per user per month. GitHub, for example, prices its copilot at $10 to $39 per user per month. We expect outcomes to emerge as a pricing basis as GenAI models become more specific and more integrated into businesses.
Companies’ choice of pricing model can also reveal information about the fate of their team members. If most successful GenAI applications are priced per user on a subscription basis, they are likely to enhance human performance. If the predominant models are based on consumption per task or per outcome, then the applications are more likely to replace humans or diminish their roles.
Companies can choose their pricing model strategically by integrating key insights from the three information sources (customer value, competitive landscape, and economics) into three parameters: the choice of data source to train the GenAI model, the operating environment, and the perceived value for customers. (See Exhibit 4.) Even then, the choice of model is not prescriptive but rather a conscious and careful decision. Companies must weigh several factors as they choose among the three models: consumption based, subscription based, and outcome based.
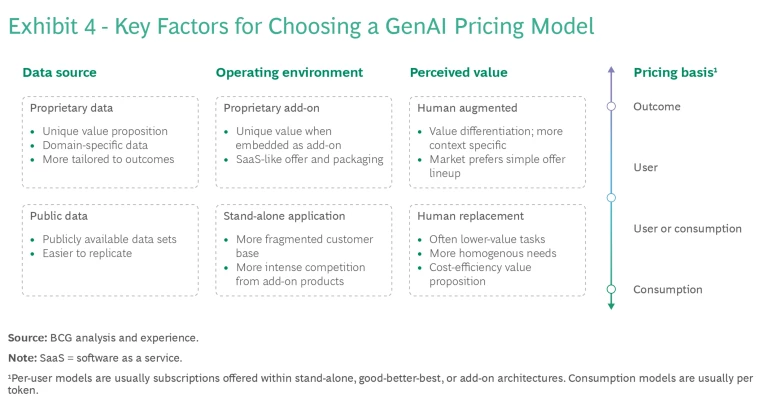
- Consumption-based model: Tokens, the main unit of consumption, are proxies for costs, and marginal costs are high for some GenAI models. While some companies may use this as a rationale to charge higher prices in the short term, we expect that costs will decline significantly in the future, akin to how semiconductor manufacturing costs declined in line with Moore’s Law. Success with a consumption-based model depends on a company’s ability to optimize all aspects of the cost equation in-house and to influence customer behavior to better align with costs.
- Subscription-based model: The less commoditized an application, the more a subscription-based pricing model makes sense. Microsoft, for example, set its Copilot “digital companion” at $30 per user per month for enterprise customers. Such flat-rate models are easy for both parties to understand, but variable costs can put the margins of these subscription models at risk in the short term. The use of flat rates in the early stages of other rapidly growing markets—such as internet usage and mobile telephony—has shown that unit costs can rise quickly if usage varies significantly among customers. While some vendors may start out with a simple pricing model such as price per user, they may have the opportunity to move to outcome-based prices as the market evolves.
- Outcome-based model: When choosing a pricing model, companies should not confuse outputs and outcomes. Doing so can lead to the assumption that consumption pricing aligns well with value and should be the predominant model for GenAI solutions. The simple example of an automated customer service chatbot shows the risks. A consumption-based model works when the pricing metric is the number of interactions the chatbot has with customers. An outcome-based model works when the pricing metric is, for example, the number of cases resolved that are rated “satisfied” by a customer. The latter group may be much smaller in volume but much more valuable for the company. An outcome-based model creates incentives for the developer to think about the business context, workflow integration, and model refinements that may be needed to deliver true value.
After the company has decided on the pricing basis (the unit and timing of price), it can move to the second major decision for its pricing model: the offer architecture, which is how it will package its innovations. There are three offer architectures to consider for GenAI solutions:
- Single GenAI platform: ChatGPT was packaged as a standalone free application when it was first released. This kind of model works best when the value is highly differentiated and driven by one or two “killer features” that everyone needs.
- Good-better-best offers: GPT and Claude both have higher (better) and lower (“good enough”) performance models with very different price points. Good-better-best architectures are a good fit for offers that have enough features to allow for differentiation across tiers and have achieved enough market maturity for customer segments to emerge.
- Enrichment of existing offers: For existing digital players who are adding GenAI capabilities, an add-on offer to existing platforms may make more sense than a standalone platform. Many companies are adding GenAI capabilities as features to existing products. The motivations behind this packaging structure are simplicity and the need to augment the core offer’s value proposition. When packaging GenAI within existing offers, however, it is important for the firm to adapt its pricing model to capture true differential value and costs. Such changes could mean building an allowance for a number of interactions or tokens into GenAI offerings and using a secondary pricing basis to capture value when power users exceed that allowance.
***
The transformative potential of GenAI is so vast that it requires a unique approach to pricing strategies. The market is so dynamic and the solutions are so differentiated that each vendor needs to focus on developing the pricing strategies that work best for their own needs. In this constantly evolving space, we have found three no-regret moves:
- Get crystal clear on what drives value and differentiation. The more differentiated the offer (in terms of performance, proprietary data, and domain specificity), and the more it augments rather than replaces the end user, the more you can price to value and use a subscription or outcome-based model.
- Go deep on your economics, now and in the future. The cost-to-serve profile of GenAI-based solutions can vary dramatically, depending on the underlying LLM, user profile, and evolution over time. Invest in understanding how your costs scale and what your economics will be at scale (in 12 to 24 months, for example). Short-term economics are important but not sufficient to help firms make a strategic pricing decision.
- Play the right pricing game. Two factors will ultimately drive the best pricing model: the market structure—especially its growth potential and the balance of power among customers and competitors—and the differentiation of the offers. Highly differentiated offers with few vendors and many customers are best supported with what we call the Value Game (one unique offer) or the Choice Game (multiple players with segmented customer bases).
Companies will be better equipped to navigate this challenging market when they have a clearer understanding of the pricing landscape and how to set a smart pricing strategy that does justice to the transformative power of their GenAI solutions.