Artificial intelligence has been making inroads in drug discovery for a good part of the last decade. We recently published an analysis that showed that biotech companies using an AI-first approach have more than 150 small-molecule drugs in discovery and more than 15 already in clinical trials. This AI-fueled pipeline has been expanding at an annual rate of almost 40%.
Given the transformative potential of AI, pharma companies need to plan for a future in which AI is routinely used in drug discovery. New players are scaling up fast and creating significant value, but the applications are diverse and pharma companies need to determine where and how AI can most add value for them. In practice, this means spending the time needed to understand the full impact that AI is having on R&D, which includes separating hype from actual achievement and recognizing the difference between individual software solutions and end-to-end AI-enabled drug discovery.
It may be tempting to think that AI can be delivered through a new tool or a single team. From our experience with many companies, this is rarely the case. Instead, achieving full value from AI requires a transformation of the discovery process. To take advantage, companies must make investments in data, technology, and new skills and behaviors throughout the R&D organization. They can look to the AI-first drug discovery startups that are leading the way for lessons and a roadmap for the journey ahead.
A New Wave of Drug Discovery Value
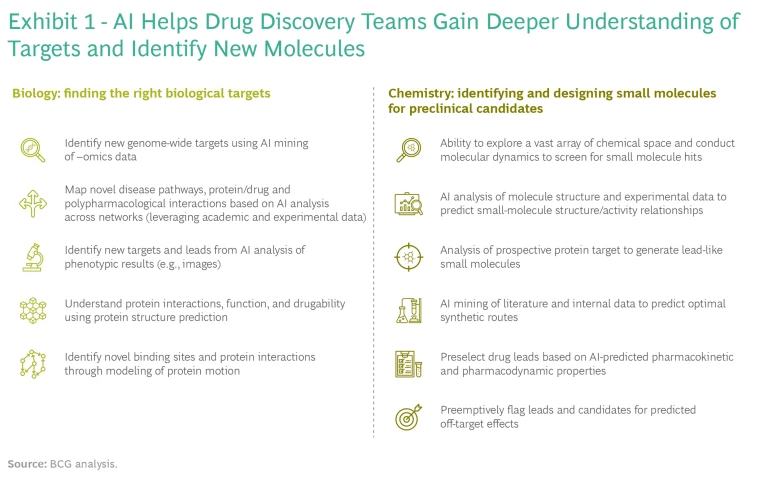
AI can deliver value in small-molecule drug discovery in four ways: access to new biology, improved or novel chemistry, better success rates, and quicker and cheaper discovery processes. The technology can address many challenges and constraints in traditional R&D. Each application brings additional insights to drug discovery teams, and in some cases can fundamentally redefine long-standing workflows. Because these technologies are applicable to a variety of discovery contexts and biological targets, understanding and differentiating among use cases is critical. (See Exhibit 1.)
Much of the historical progress has been led by AI-native drug discovery companies that offer software or a service to pharma players. These firms use data and analytics to improve one or more specific use cases at various points in the value chain. Examples include target discovery and validation using knowledge graphs and small-molecule design using generative neural networks. Large pharma companies have been able to gain access to these capabilities through partnerships or software licensing deals and then apply them in their own pipelines.
The last few years have seen several AI-native drug discovery companies build their own end-to-end drug discovery capabilities and internal pipelines, launching a new breed of biotech firm. In addition, many of these players are also exploring innovative business models . For example, Atomwise and Schrödinger formed a joint venture with a shared portfolio, and Roivant Sciences acquired Silicon Therapeutics to combine distinct platform technologies.
The move from traditional service and software models to asset development partnerships and pipeline development has led to soaring investment. Third-party investment in AI-enabled drug discovery has more than doubled annually for the last five years, topping $2.4 billion in 2020 and reaching more than $5.2 billion at the end of 2021. These figures exclude the amounts that pharma companies are investing in their internal capabilities and investments by tech giants, which have also been active in expanding their AI investments into biology and drug research. For instance, Alphabet recently launched Isomorphic Labs based on AI breakthroughs at its DeepMind AI operation, Nvidia has invested in the Clara suite of AI tools and applications, and Baidu’s AI drug discovery unit has struck a major deal with Sanofi.
The impact of AI on traditional drug discovery is in its early stages, but we have already seen that when layered into a traditional process, AI-enabled capabilities can substantially speed up or otherwise improve individual steps and reduce the costs of running expensive experiments. Indeed, AI algorithms have the potential to transform most discovery tasks (such as molecule design and testing) so that physical experiments need to be conducted only when required to validate results.
The AI-First Model
AI-native biotech companies offer a glimpse of this AI-first model. Many have stacked capabilities end to end, reshaping the drug discovery and development process and harnessing the operational benefits of a redefined value chain. To accelerate discovery and ensure that they focus on competitively differentiating capabilities, these AI biotechs often use an ecosystem of partners, including academic researchers to provide target expertise, contract research organizations (CROs) to do wet-lab experimentation, and other industry partners to codevelop and commercialize assets.
When layered into a traditional process, AI-enabled capabilities can substantially speed up or otherwise improve individual steps and reduce the costs of running expensive experiments.
Using the ecosystem approach, one emerging biotech has built AI capabilities that include precision targeting, generation and optimization of clinical candidates, and clinical-trial optimization based on predictions of the best therapy using patient samples. The firm does have in-house experimental capabilities but focuses these on generating data to support its AI models and building expertise in selected therapeutic areas for internal assets. In other areas, it accesses broader scientific expertise and clinical experience through partnerships.
Another AI biotech has built a suite of offerings that include multiomics target identification and a chemistry platform, as well as clinical-trial prediction tools. In combination with compound synthesis services from CROs and expertise from academia and larger pharma codevelopment partners, these tools have allowed the firm to cut the time needed to identify three preclinical candidates to between 12 and 18 months, compared with the three to five years typically required by traditional players.
Companies that control the full AI-enabled discovery process crucially own the IP underpinning their assets. They leverage a network of partners, including CROs and contract development and manufacturing companies, but retain molecule ownership. Their assets potentially have significant commercial value through out-licensing, joint ventures (typically after clinical proof of concept), and therapeutics marketing. The maturing AI-first model has accelerated the shift among AI-native players from software or service providers to asset-owning biotechs in their own right.
While we are still some years away from seeing AI-discovered or partner-developed assets reach the market, our analysis shows a building wave of these treatments entering preclinical trials in the last five years, portending a similar pattern for clinical trials. If even a fraction of the cost and time benefits of AI technology is realized, this would represent a fundamental reshaping of the economics of discovery, allowing pharma companies to take more “shots on goal.”
A Roadmap for Pharma Companies
The AI revolution in drug discovery will not happen overnight. Even as AI-driven innovations show impressive results, established pharmaceutical companies retain many advantages. These include capital, scientific expertise, development know-how and experience, regulatory expertise, and established branding and commercial teams. That said, some of the pillars of incumbency are showing early erosion. Massive fundraising and less cost-intensive in vitro work are lowering the capital barriers for startup discovery programs. Meanwhile, AI natives are filling out their ranks with scientists and medical experts, replicating the advantages of big companies employee by employee.
It's possible—though not easy—to combine the best of both worlds. In our experience, adapting a classical drug discovery process and delivering on the promise of AI require long-term action on five strategic and operational tracks. (See Exhibit 2.)
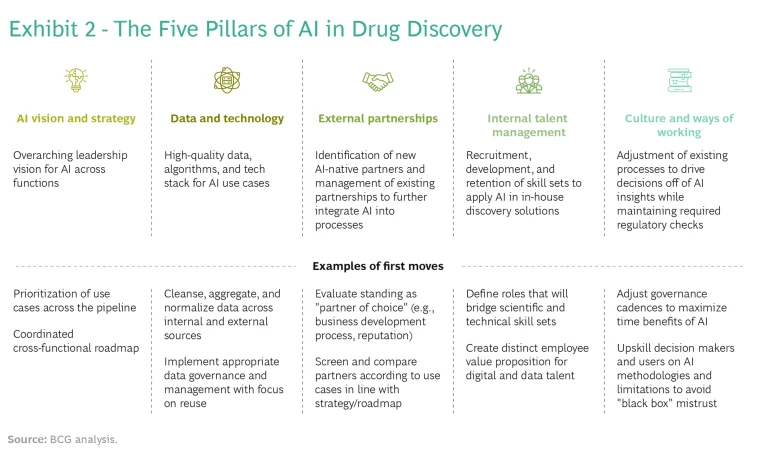
AI Vision and Strategy. Companies need to develop an AI roadmap that identifies specific, high-value use cases that are aligned with specific discovery programs. Focus and prioritization are key: companies should identify a small number of use cases (typically, five to seven) spread across programs or stages of discovery. These use cases should directly reflect the company's R&D strategy or financial goals—otherwise, AI will be seen as a sideline. Use cases must have support from senior leadership and “pull” from discovery and development teams.
Data and Technology. Don’t wait for large data and technology platforms to become available to get started. Before building an entire tool or platform, focus on attaining a proof-of-concept algorithm: the minimum sufficient analysis that confirms your ability to extract valuable insights from your data in a specific scientific context. If the insights are sufficiently valuable, you can then invest in industrializing the tool and adding a friendlier user interface. For example, one company developed, in about ten weeks, an AI-based tool for optimizing the formulation conditions for proteins based on a combination of existing in-house data and externally available stability data—a typical timeframe for go or no-go decisions on a proof-of-concept algorithm. This type of exercise can help embed data governance and cleansing processes throughout the organization, building the foundation for the next application, and companies can quickly redeploy resources when there’s no identified ROI.
External AI Partnerships. Partnerships are, and will continue to be, an effective way to accelerate adoption of AI-led discovery techniques and create strong value propositions. Given the wealth of biological and chemical targets available, drug discovery is not a zero-sum game. In fact, the amount of capital, talent, and new data being put to work in AI drug discovery is far greater than any one company can devote on its own.
Pharma companies, though, will need to adopt new behaviors and ways of working to become partners of choice for AI players. Ask yourself such questions as the following: How easy do you make it to share data quickly and securely with external organizations? Do you have a culture of openness and scientific collaboration? How long does it typically take to get a partnership negotiated and off the ground? Such factors may not seem critical, but they can make a big difference to potential partners that may have a choice of whom to work with.
Subscribe to our Biopharma E-Alert.
If you already have established partnerships, evaluate the lessons—and the bottlenecks—they have revealed so far. Seek feedback from your partners on what they would do differently if they were running your portfolio.
Internal Talent Management. Even if you are not building use cases internally, you will need digital talent to help steer projects with partners and perform due diligence on potential partners. Data scientists and engineers are a different breed and don’t always fit neatly into medically focused organizations and cultures. One company developed an employee value proposition specifically for scarce digital talent. To help retain these employees, it made sure to assign new hires to high-visibility projects and promote the results. These efforts enabled the company to stand out from deep-pocketed tech companies and other employers offering equity packages with high-growth potential.
AI algorithms have the potential to transform most discovery tasks (such as molecule design and testing) so that physical experiments need to be conducted only when required to validate results.
But pharma companies require more than software and data science skills. They will also likely need to train senior decision makers on how AI-generated recommendations are reached, if only to prevent revalidating proposals with traditional approaches. Medical scientists must be conversant (but not necessarily fluent) in the analytical approaches needed to understand and pressure test what is emerging from the algorithms.
Culture and Ways of Working. Companies will not reap the benefits of AI unless they adapt their processes to the faster pace of in silico work. In many situations, this involves fewer and shorter cycles of hit identification and lead optimization. As a result, companies may run many more discovery programs in parallel than they have in the past, requiring a shift in culture and ways of working. For example, companies may need to increase the frequency of portfolio board reviews to meet the speed of an AI-driven workflow. They will need to invest time in helping decision makers question and gain trust in outputs. New AI-based governance models will likely be necessary to ensure that biases are systematically investigated and removed from AI-led processes.
The primary function of lab work—the bedrock of classical drug discovery—will also change. Experimental work will go from a starring to a supporting role, focusing on areas in which results from in silico drug discovery need to be validated (for regulatory purposes, for example) and areas in which AI technology does not (yet) work reliably. Experimentation will also be needed to fill gaps in data sets to make the AI processes more robust.
What Pharma Companies Can Do Right Now
To get started (or to continue an ongoing exploration), pharma companies should consider a few key steps.
Create a clear strategic vision and ambition. Decide where to apply AI and be clear about the changes you expect. Determine whether to use AI to optimize the current discovery process or to transform the discovery program using an AI-first model. Clearly define the outcomes you seek, whether it’s explicit cost and time savings, the generation of novel targets, or progress on previously “undruggable” diseases. Lack of clarity on objectives risks individual initiatives ending up as bench experiments or small-impact trial cases with limited potential. It's also important to bear in mind that the landscape is evolving rapidly, so your vision and ambition should be re-evaluated regularly.
Set a roadmap for action. Companies should identify and prioritize a handful of high-value, high-impact use cases to pursue within a 12- to 24-month timeframe. Ideally, these will build on existing discovery or clinical-development efforts in which AI can accelerate predefined outcomes consistent with the strategic vision.
Initiatives that “test” and “evaluate” AI’s impact should be minimized, as these are often rooted in skepticism over the new approach. Instead, gear the AI roadmap toward identifying opportunities to adopt and scale. When picking use cases, it's critical to differentiate among three types of development: those best suited for in-house development, those that can be implemented with third-party services or software suites, and those requiring external partners. Key questions include: Where do we have a data advantage? Where have we shown internal value proofs? And where have we already built the necessary scientific, AI, and machine-learning muscle?
Be bold but don’t underestimate resistance to change. Scaling up AI can be challenging. Teams tend to be set in established processes and comfortable with the tools that have proven successful for years. Companies need to make a statement of commitment to AI by targeting entire workflows or assets that force a full review of ways of working. For example, instead of simply adding a prediction tool to existing lead optimization processes (which may limit the visible impact and dissuade teams from testing new procedures or workflows), consider incorporating multiple AI use cases into an end-to-end new-asset discovery process, which requires rethinking how traditional governance models are deployed. It's also critical to bring the entire organization on the journey. Management should stress the transformative R&D ambition from the get-go, share value proofs and lessons from internal teams, and build a wave of excitement and momentum over time to cut through resistance.
AI offers major technological advances that may represent a paradigm shift in drug discovery and, ultimately, clinical development. We believe that advances that now feel like a sea change will rapidly become table stakes in discovery speed, novelty, and commercial potential. Many use cases are already maturing to the point where the impact is well understood. Acting boldly, with a clearly articulated strategy that resets a few key opportunities in your discovery efforts, can set you on the right path. The companies that move quickly will be the biggest winners.