As rail operators face falling demand in the post-pandemic era, many report that their rolling stock maintenance costs have continued to rise. US transit and commuter rail operators saw those costs increase by approximately 4% per year from 2010 through 2019, according to Federal Transit Authority data, outpacing the recent significant increases in inflation. Labor shortages, supply chain disruptions, and antiquated fleets are largely to blame. Moreover, rail operators lag other industries in embedding data and the latest digital technology into their maintenance and support functions.
Operators can combat escalating costs by better employing existing data to plan and execute maintenance, or by utilizing new technologies to generate new data sources in support of predictive maintenance.
Simply deploying existing data can have an outsized impact on costs, particularly since it entails low, if any, upfront technology expenditures. For example, a US transit agency used its existing data to develop failure curves for key systems. It applied the insights to improve schedule accuracy and parts availability, resulting in a 10% decline in rolling stock maintenance costs.
Operators can also experiment with the latest technologies to facilitate the collection and analysis of data from new sources, such as sensors and digital maintenance records. They can utilize such sensor data and analyses to preemptively diagnose potential failures and assess the potential to scale these solutions across fleets. An Asian rail operator used digitally enabled predictive maintenance to ensure the availability of all necessary parts when equipment arrived for scheduled maintenance. This allowed it to reduce the time its rolling stock spent in maintenance depots by 20% to 30%.
Success, however, requires a pragmatic approach. This begins with an assessment of the data, sensors, and other technologies currently used by an operator’s rolling stock maintenance functions. The maturity assessment outlined below can help operators lay the foundation for capturing more value from existing resources before planning investments in new capabilities.
How Digital Enhances Rolling Stock Maintenance
Most rolling stock maintenance efforts focus on five major train systems: brakes; chassis (including the wheels, axles, and truck); propulsion; doors; and heating, ventilation, and air-conditioning (HVAC) systems. To improve each system’s reliability and efficiency, operators can capture data from existing sensors and also install new sensors to gather additional types of data. (See “Sensors Support Maintenance Across Five Systems.”) For instance, infrared sensors and thermal cameras can detect when brakes start to overheat and require maintenance to reduce the risk of “sticking.” Similarly, accelerometers and inclinometers can monitor the stress on a train’s propulsion systems from continuous vibrations, accelerations, and decelerations.
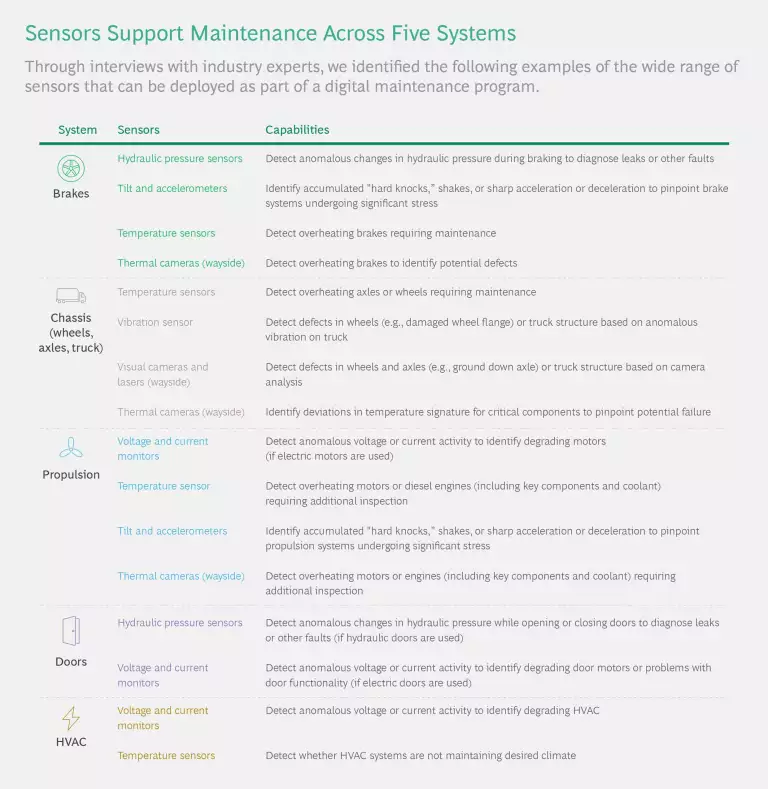
By utilizing existing data and creating new, high-value data feeds (via sensors) as part of a comprehensive digital maintenance program, rail operators can realize five key benefits:
- Increased Reliability. Predicting and better understanding failures empowers operators to perform maintenance proactively and more efficiently. The enhanced knowledge of defects accelerates efforts to diagnose issues. This leads to fewer maintenance events and faster repairs, ultimately reducing equipment downtime and providing higher levels of service.
- Reduced Need for Spare Equipment. Faster repair diagnostics and fewer unnecessary scheduled maintenance visits mean that rolling stock spends less time in maintenance depots. As a result, fewer trains (and a lower spare factor) are required to provide the same level of service.
- Reduced Maintenance Labor Spend. Operators can use various approaches to reduce shop floor labor costs. For example, they can apply analytics to reduce repair times or unnecessary maintenance (within regulatory guidelines). They can also pre-position parts at a maintenance site to decrease the time that mechanics spend waiting for materials.
- Improved Material Availability at Lower Cost. Operators can use digital maintenance to eliminate the cost of unnecessary scheduled maintenance, including overhauls, and of parts replacements resulting from inaccurate failure diagnostics. Airline maintenance operations set the standard for implementing this approach and capturing its benefits. They have made significant progress in optimizing their spare-parts inventory through AI and machine learning to predict spare-parts requirements and trigger order times with high accuracy. Few, if any, rail operators have deployed a similar capability.
- Improved Maintenance Planning and Forecasting. Early warnings from sensors enable more accurate modeling of rolling stock maintenance requirements and predict costly failures with sufficient lead time to preempt them. With improved forecasting, operators can better balance the workload across their labor force and optimize the use of maintenance facilities. In addition, tracking failures and asset data over time allows operators to utilize AI to better predict the maintenance needs of specific assets and the timing of maintenance work.
Fully realizing these benefits is not easy, however. For one, maintenance organizations must have the technical and data infrastructure (including clean data) in place to access and analyze sensor inputs effectively. But more often, it’s nontechnical issues such as the following that prevent operators from achieving the expected benefits:
- Rigid maintenance strategies or regimes that do not use data to refine maintenance practices (for example, de-averaging preventive maintenance across fleet models) or make other decisions
- Demand forecasting methods that do not use data to maximize uptime while reducing labor costs
- Siloed supply chain functions that do not incorporate the latest data to manage and deploy maintenance materials effectively
- Insufficient training and/or talent capabilities that prevent workers on the shop floor from using the full toolkit and recording data and information with high quality and integrity
Conducting a Digital Maintenance Maturity Assessment
The starting point for addressing these challenges is a holistic assessment of a rail operator’s current digital maintenance maturity. The assessment identifies how the operator can best deploy its existing data, sensors, and other technology, as well as where it may benefit from deploying additional sensors and other technology.
Several questions can help operators determine where they sit on the digital maintenance maturity curve:
- Do we use manual diagnostic and inspection processes to identify equipment needing repairs, with individual workers looking for defects? Or are we making use of sensors and data that can predict and preempt the need for repairs automatically?
- Are we monitoring trains in an integrated fashion—or by using tools in an ad-hoc fashion, component by component?
- Are we monitoring our fleets in real time across the network or only when trains sit parked in maintenance shops?
- Can we predict which equipment is likely to fail next solely by monitoring each train’s onboard systems?
Rail operators can assess their digital maintenance maturity using a common multilevel scale:
- Level 0, the lowest rung, describes rail operators that still conduct manual and experience-based diagnostics of failures based on visual inspections at set intervals, or by using data from past work orders to create maintenance protocols.
- Level 1 describes organizations whose workers examine select components for faults using diagnostic tools after trains arrive at a maintenance shop. For instance, if a train arrives with a propulsion failure, workers will download diagnostic data from a propulsion controller to determine which component needs to be replaced.
- Level 2 organizations use integrated diagnostic tools to collect and diagnose telematics data across major components in a standardized manner when trains come in for maintenance. After a train pulls in, workers begin the inspection using software to see which of the major components need to be repaired or replaced.
- Level 3 operators are those that conduct real-time monitoring of the condition of components and alert maintenance workers when failures occur. For example, the maintenance team receives real-time reports from trains when the HVAC system fails owing to a problem with temperature sensors. Because the team receives these reports, it does not need to conduct daily inspections to test the system’s functionality.
- Level 4 is the maturity that most operators should aspire to reach. At this level, an alert system uses predictive monitoring of sensor and external data to notify the maintenance team of the need to take preemptive action before equipment fails. For example, software applies sensor and usage data to predict that brake pad thickness is likely to fall below the safety threshold within the next 1,000 miles.
Operators should apply this scale to assess the sensors and other technologies used across the four core functions of the maintenance organization:
- Maintenance strategy is the approach to key elements of the maintenance program (for example, inspections, overhaul regime, and running repairs) and rolling stock engineering (for example, design decisions on key components and parts for rolling stock).
- Forecasting includes maintenance planning (such as job scheduling) that promotes efficiency (such as reduced shop floor downtime) while minimizing service disruptions.
- Supply chain and materials management encompasses the entire materials management process, from procurement and inventory to shop floor deployment.
- Maintenance operations and execution includes enablers and functions required for successful maintenance work, such as data, analytics, talent, workflow, and production planning.
By systematically assessing digital maintenance maturity across these four functions, operators can pinpoint specific gaps. In many cases, they can create value without necessarily advancing to higher levels of maturity. For example, a level 1 operator might already have an effective maintenance strategy if it tailors the overhaul intervals for each fleet based on failure curves constructed from historical data and conducts its running repair diagnostics using automated tools instead of manual inspections. However, the same organization might face challenges in its
supply chain
and materials management function if diagnostic tools for running repairs are not connected to the material management systems. Such a gap could result in downtime to allow mechanics to conduct repairs. Connecting the tools and systems would increase mechanics' “wrench time” without requiring major rolling stock upgrades.
Next Steps
Rail operators can apply the insights from the assessment to develop a prioritized roadmap for increasing their digital maintenance maturity. This roadmap should address resource availability, the optimization of essential maintenance processes, and targeted training programs. An effective roadmap identifies quick wins to create value. Operators can then apply the savings to fund medium-term investments in new technology to structurally transform their operations.
To take these actions effectively, operators must be willing to put aside traditional practices, such as scheduled maintenance, as well as adopt new tools and approaches. For example, digital maintenance is a key consideration in a total-cost-of-ownership approach to rolling stock procurement . By embracing a more proactive, digitally empowered approach to maintenance, operators can usher in a new era of fleet management—with more uptime, greater efficiency, and lower costs.