There is little question that
generative AI (GenAI) will transform
how biopharma companies develop, produce, and commercialize new treatments. We have already seen big reductions in drug R&D timelines. The potential across the rest of the value chain is clear.
But implementing
GenAI
is not easy in a complex industry with intensive regulatory oversight. GenAI’s sprawling capabilities present challenges with respect to where and how it should be applied, when and how to scale up its use, and how to manage the resulting large-scale change. Establishing the conditions for rapid experimentation and scaling requires a tight convergence of technology and strategy, with deliberate choices grounded in the circumstances of the individual organization.
An Abundance of Potential, Choices, and Constraints
Much of GenAI’s potential grows out of three game-changing characteristics:
- The ability to process (and learn from) terabytes of structured and unstructured data (such as academic literature, clinical-trial information, batch records, quality investigation reports, electronic medical records, and claims databases)
- The ability to generate completely new content: text, media (image, speech, and video), design, and code
- Intuitive, conversational user interfaces that reduce AI’s adoption hurdles, making the technology widely accessible
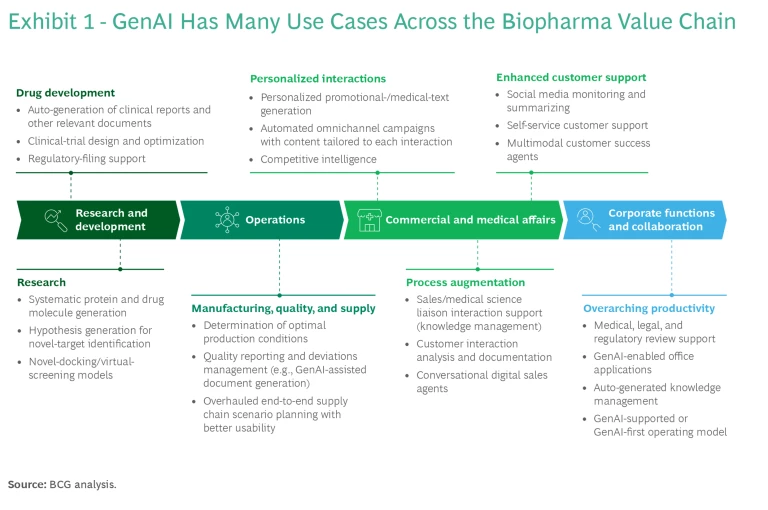
In biopharma, GenAI can be applied at all stages of the value chain— operations , commercial and medical affairs, and corporate functions , in addition to R&D—with the potential to substantially increase efficiency and effectiveness by transforming existing processes. (See Exhibit 1.) But GenAI also carries risks, which can be magnified in an industry like biopharma, where patient safety is paramount. Still, the breadth of opportunity presented by the technology, combined with the biopharma industry’s unique mix of characteristics, means companies will wrestle with both complexity and an abundance of choices in how to approach GenAI.
Consider data. Biopharma already taps into a highly varied data landscape and applies deep scientific and market expertise to extract value. With its ability to process unstructured data, GenAI adds a vast array of new data sources to the mix. As the use of GenAI spreads, unstructured data becomes much more valuable, and companies need to rethink their data management strategies to encompass those sources.
The biopharma value chain is much less risk tolerant than that of other industries. Again, think about data access and management. Companies must address the practical constraints of using GenAI to manage regulatory-grade data generated from clinical trials in transactional systems, as well as less sensitive data used in business systems, such as finance and HR.
Companies must also be able to explain results and how solutions were developed. The EU has already made clear that AI systems used in its member states must be “safe, transparent, traceable, non-discriminatory and environmentally friendly,” as well as “overseen by people, rather than by automation, to prevent harmful outcomes.” Given the risk profile associated with biopharma workflows, companies need to adopt and follow a policy of “ responsible AI ” and establish associated governance procedures throughout their organizations.
Companies will wrestle with both complexity and an abundance of choices in how to approach GenAI.
AI-enabled processes in biopharma must also be integrated with human activities (think quality assurance, wet labs in R&D, or sales calls in commercial). To reap the full benefits of GenAI, companies will need to reimagine core processes, in some cases end to end.
Complexity and constraints can be catalysts for invention. BCG has catalogued more than 130 potential use cases across the biopharma value chain, including target patient identification using unstructured health record data; end-to-end supply chain scenario planning; customized promotional- and medical-text generation; conversational
digital sales
agents; self-service customer support; and automated office applications. GenAI does not supplant or replace existing AI and in most cases will work in concert with existing models. There are also places where GenAI
may not be the best solution
. Prioritization of use cases that have clear, measurable goals will be critical, as will remembering that use cases can evolve over time, from incremental to transformative in impact.
The Most Promising Use Cases
Biopharma use cases range from “table stakes”—those with low barriers to adoption that use off-the-shelf models to improve efficiency and enhance operations across corporate functions (such as HR, finance, IT, and legal)—to those that truly strengthen competitive positioning and build long-term advantage. BCG estimates that GenAI solutions in biopharma could generate up to a 30% improvement in productivity for some functions.
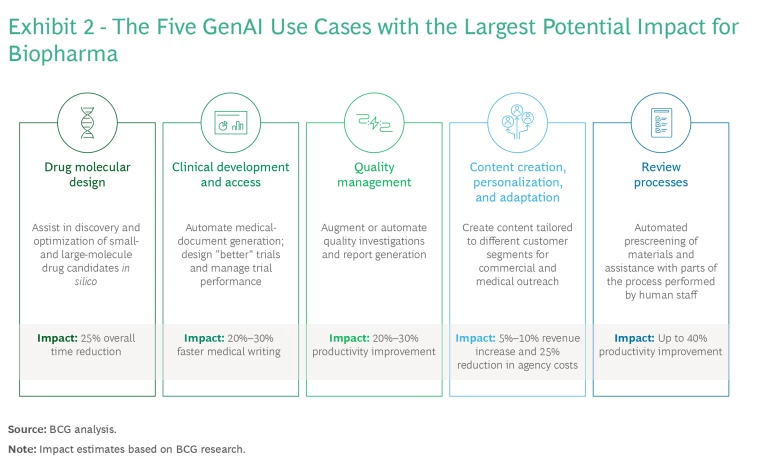
At the high-impact end, we are seeing leading biopharma companies converge around five “golden” use cases where we believe much of the differentiated value of GenAI will ultimately be concentrated. (See Exhibit 2.) These include the following:
- Faster Drug Molecular Design. GenAI can accelerate early-stage drug breakthroughs by assisting in the discovery and optimization of small- and large-molecule drug candidates in silico, which also expedites later stages of research as new discoveries move closer to clinical trials. AI has been successfully used in this context for many years, resulting in time reductions of 25%. GenAI can be expected to accelerate drug design further.
- Accelerated Clinical Development and Access. GenAI speeds up clinical development and access in two principal ways. The first is automating medical-document generation in clinical development (for example, protocols, clinical-study reports, and regulatory affairs submissions), which can reduce medical-writing time by as much as 30%. Second, GenAI can improve clinical-trial design and help manage trial performance by generating simpler, more effective protocols and using simulations to prevent delays.
- More Efficient Quality Management. As part of the quality assurance process in manufacturing, GenAI can augment or automate quality- and regulatory-related analysis and report generation, such as by managing deviations from standard procedures and supporting annual product quality reviews. The technology can surface patterns and recurring issues in large volumes of documentation from diverse sources (such as quality systems, manufacturing systems, and suppliers’ data) and help generate preliminary reports on quality outcomes that are essential for regulatory compliance . BCG estimates that augmenting these routine tasks could increase staff productivity by up to 30%.
- More Effective Content Creation, Personalization, and Adaptation. GenAI can help collect and organize complex information from different data sources to create truly personalized interactions (in support of frontline sales and medical teams, for example). It can also adapt content to different cultural contexts and channels. Applications include training, supporting interactions with health care professionals, and streamlining patient support services. We expect such efforts to generate revenue increases of up to 10% and to reduce external agency costs by 25% or more.
- Facilitated Review Processes. With greater content personalization, medical, legal, and regulatory reviews will become a bigger bottleneck. GenAI can facilitate the review process by automating the prescreening of materials and assisting in the parts of the process performed by human staff (identifying risks, referencing claims, and refining drafts, for example). In our experience, GenAI can increase the productivity of these high-frequency tasks by up to 40%.
Six Guiding Principles for Implementation
Realizing value from GenAI is not automatic. It takes careful assessment, planning, and implementation. While more basic GenAI applications, which rely on off-the-shelf models to improve productivity and efficiency, are important for keeping pace with other organizations, scaling the right high-impact use cases will be the major driver of value. Biopharma companies need to be realistic about where they can truly achieve step-change improvements (especially with processes that are not fully automated) and where the advertised potential seems heavy on hype.
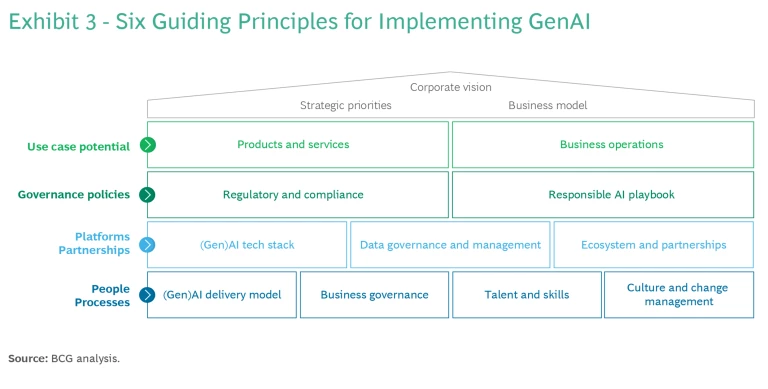
Here are six guiding principles for achieving value quickly and scaling the benefits of GenAI in biopharma efficiently. (See Exhibit 3.)
1. Assess Use Case Potential
Biopharma companies should prioritize GenAI use cases based on their value potential, feasibility (a function of data availability, complexity, cost, and time to value), and the capabilities that the technology will help build. Use cases need to be anchored in transformed biopharma workflows that employ the right combination of GenAI, traditional AI or machine learning, and human involvement. The exploration of GenAI use cases also needs to be tightly integrated with the core business, bringing together tech and business functions.
2. Establish Strong Governance Policies
GenAI comes with risks, among them IP and copyright infringement, cybersecurity and data privacy vulnerabilities, biased outputs, opaque results that may be hard to explain, and false results (known as hallucinations). The risks are only heightened when the data used includes highly sensitive patient information. Fortunately, the risks can be mitigated with strong guardrails and governance built around responsible AI policies and procedures, but these must be embedded in the company’s culture and operating model.
Responsible AI can be woven into existing governance processes in different ways. Biopharma companies should determine responsibility for GenAI policy development (an ethics board, for example). They should build a responsible AI framework (with rules for data inputs, model transparency, and the use of insights derived from outputs) and implement a data classification framework with risk-based mitigation measures that are stratified by degree of data sensitivity and consequences for incorrect or biased output or predictions.
Patient safety and regulatory compliance are paramount. The regulatory landscape is rapidly evolving, and internal guidelines will need to change as regulatory bodies, such as the European Medicines Agency and the US Food and Drug Administration, publish new frameworks. There may be some potential for cross-industry collaboration in helping to shape and define responsible AI guidance and policies in biopharma.
3. Select and Deploy Platforms
To get the most from GenAI, companies need enterprise-wide infrastructure and platforms that give people access to the tools and give the tools access to data. Biopharma companies will have to make platform choices that span the entire tech stack, including secure cloud infrastructure, data platforms, models, and applications.
Foundation model choices include off-the-shelf public models, managed models (for example, one that a cloud service provider makes accessible via an API), and private models built in-house, but there are clear tradeoffs involving cost, speed, and customization. Nvidia, for example, is offering a set of generative AI cloud services that enable customization of AI foundation models to accelerate drug discovery and research in genomics, chemistry, biology, and molecular dynamics. A number of startups and incumbents have embraced the service, but each company needs to look at such offerings in light of whether its own internal unstructured data confers a competitive advantage.
Biopharma companies will have to make platform choices that span the entire tech stack.
To achieve maximum impact, GenAI also needs to be integrated with existing AI tools. An essential first step is deploying a strong analytics platform that can enable rapid experimentation. Most companies will need to strengthen their
data capabilities
to meet multiple priorities:
- Building and bringing together unstructured proprietary data sets and tapping into external data ecosystems
- Establishing a data platform as a “single source of truth” for the enterprise and making enterprise-wide data readily available for model training and fine-tuning while adhering to responsible AI guidelines
- Creating an environment for safe experimentation, with appropriate cybersecurity and data privacy measures in place
AI is evolving quickly. It is important to build in flexibility and ensure the ability to draw on different foundational models in the future. Companies should assess the readiness of current data platforms and core systems to accommodate emerging GenAI capabilities. Piloting lower-risk use cases to start can help in evaluating your platform and revealing lessons to keep in mind as you expand your GenAI capabilities.
Choosing whom to work with, where, and in what roles requires determining which capabilities should be built in-house and which sourced from outside.
4. Seek the Right Partnerships
The right partners can significantly increase speed to value, but companies need partnering arrangements that are rooted in business strategy and goals. They should explore partnerships that will help them obtain the needed data, technology, talent, and assistance with implementation. Choosing whom to work with, where, and in what roles requires determining which capabilities should be built in-house and which sourced from outside. Data ownership and IP considerations are paramount, but there are tradeoffs. Internal model development ensures protection of data and IP, for example, but it can take time, while drawing on the larger tech ecosystem is faster and more efficient.
5. Give People the Skills They Need
At each step of the value chain, staff will need training in how to use both AI and GenAI in their daily work. Researchers, manufacturing and quality control personnel, sales and medical-science teams, and employees in corporate functions will all need help building their knowledge. Include your ecosystem partners in this effort, but most companies will want to build critical capabilities internally to ensure that they have embedded institutional expertise.
6. Put in Place the Right Processes
New technologies and tools may lead to a lot of experimentation, but by themselves they will not generate value. In addition to demanding new people and skills, implementing GenAI has big implications for the organization and the operating model. (See Exhibit 4.) In our experience, about 70% of the value from GenAI is determined by how well a company manages change. Most companies will need a hands-on change management program to guide the organization through the transition.
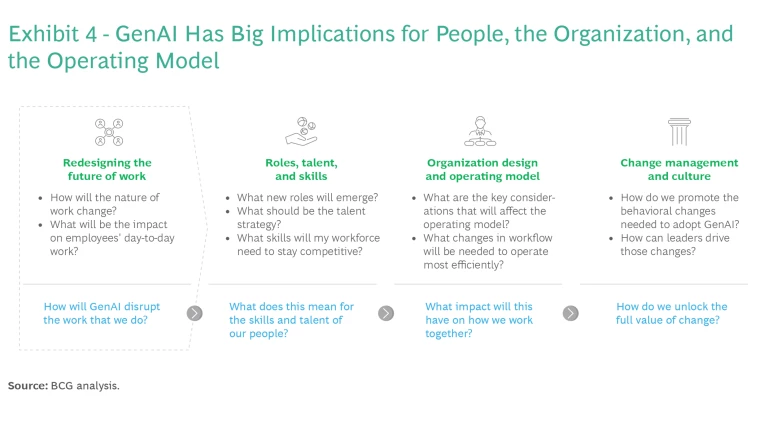
One approach is to select two or three functions and business units to serve as test beds for initial GenAI solutions and change management practices before scaling them more widely. Senior management should also identify “believers” in various functions who will help drive the change, especially if it is implemented from the top. Management can conduct a talent assessment to identify GenAI “champions” who will lead initiatives, staff teams, and recruit others to the cause.
Getting Started
To get started, companies can focus on these specific steps:
- When assessing potential, elevate three to five use cases to the enterprise level so they are sure to receive the funding and focus required to succeed. While experimentation is critical, proliferating use cases and proofs of concept in multiple parts of the business will not serve a company well. Focusing on a small number of high-impact use cases via a standardized development cycle will provide the most traction.
- To establish governance policies, create an AI ethics office or similar function to oversee responsible AI policies that focus on safety without limiting innovation.
- When selecting and deploying platforms, create a secure environment that allows for use cases to be built on different foundational models. Models are not the same and should not be treated as such. Prompts and fine-tuning should be adapted to the specific model. In addition, models will continually change so it’s important for your platform to have built-in flexibility. Concentrate on speed first, then define scale as you learn from implementation.
- When seeking partnerships, link them to value, identify what is required to deliver the use cases as quickly as possible, and look to obtain the needed foundational models, tech, data, talent, and assistance with implementation. AI-based drug discovery startups are already partnering with established biopharma companies to accelerate R&D.
- Put your people on the right track by focusing from the start on building the necessary capabilities. As with existing AI efforts, in the end the value will come from the ways in which people actually use the new solutions. Remember that GenAI is not standalone but instead works in conjunction with traditional automation, predictive AI, and human-based processes. Focus on comprehensive solutions and the accompanying skills, not just GenAI.
- To manage the organization’s evolution toward the right processes, start by reimagining core business processes end to end, concentrating on how people and technology can work together to integrate GenAI and expand automation over time. Bring the C-suite along on the journey. It’s essential to make this a corporate priority rather than just an IT task.
Biopharma’s high degree of complexity, regulation, and documentation requirements makes it a natural fit for GenAI’s ability to augment business processes. The same factors put a premium on well-planned execution. The companies that can bring strategy and GenAI technology together to work toward defined goals and outcomes will quickly gain ground over slower-moving competitors.
The authors thank Carolina Fejgielman, Marie Humblot-Ferrero, Aryana Jacobs, Stefan Leve, Anne Witt, and Keyur Patel for their contributions to this article.