Generative AI is one of today’s hottest business topics, with companies exploring its potential applications and benefits across industries and functions, including manufacturing. But despite the recent buzz, manufacturers should recognize that simply applying tools like ChatGPT on their own will not revolutionize factory operations.
Instead of replacing traditional AI, GenAI offers complementary use cases in the areas of assistance, recommendations, and autonomy that pave the way to the factory of the future. It does so through its capacity to generate content, such as text and images, tailored to specific tasks or inquiries. (See “How GenAI Works.”)
How GenAI Works
GenAI takes this approach further. Beyond merely classifying existing text, it can generate new text based on specified criteria—such as operator instructions that outline a process to resolve a particular root cause of a machine breakdown. Although the progression from classical ML to GenAI might seem incremental, it poses a fundamental technical challenge. In classical ML, the model merely needs sufficient training to confidently categorize a text fragment. In contrast, GenAI must construct a text fragment from individual words and letters, ensuring that it is grammatically correct, comprehensible, and accurately represents the process.
The number of potential outputs from GenAI is virtually limitless. Considering that there are roughly 170,000 English in current use, a mere five-word text has more than 140 septillion potential combinations. On the other hand, only a fraction of them would be grammatically correct and understandable. Among those, an even smaller fraction would accurately describe a given process to fix the root cause of a machine breakdown.
Consequently, the margin for error in GenAI models is incredibly narrow, necessitating extremely precise models. To attain this precision, GenAI must use “foundational models” instead of being trained only on context-specific data. Foundational models are trained on extensive datasets, such as all available text or images online, and are subsequently fine-tuned for specific applications. These models can be large language models (such as OpenAI’s GPT-4 or Amazon Q) or image or speech models. They seem to gain an understanding of reality from the extensive datasets. However, foundational models are observational learners that do not apply logic or reason as humans do. This means that there is no guarantee of plausible or accurate results from GenAI.
In our operator instruction example, the foundational model first learns what constitutes comprehensible and accurate text, with process descriptions being a small subset. Next, the model is fine-tuned by learning what operator instructions look like and how they correlate with given machine breakdown root causes. However, there is no assurance that the model will create correct or high-quality operator instructions. Ergonomics illustrates the problem. Because the GenAI model lacks insight into the process that fixes the root cause and the people who are the operators, it might overlook potential limitations, such as infeasible movements or inaccessible spaces. As a result, a quality assessment is always required to ensure that the recommended remediation is practical from an ergonomic perspective.
Gen AI’s greater technical complexity elevates the importance of establishing a robust technological foundation to harness its capabilities effectively. With a number of archetypes possible, manufacturers must understand the factors that determine an optimal choice. They can apply this knowledge to integrate GenAI into factory operations, considering the value-adding applications, change- and people-related initiatives, and required technological infrastructure.
Manufacturers Are Prioritizing GenAI for its Disruptive Potential
BCG recently surveyed manufacturers to understand their perspective on technology developments. (See “About the Survey.”)
Regardless of their affinity for digital technology, manufacturing executives ranked AI (including GenAI) first among technologies that could positively disrupt their operations. (See Exhibit 1.) The potential ROI warrants their enthusiasm. A BCG analysis found that the use of AI could enhance shop-floor productivity by more than 20%.
About the Survey
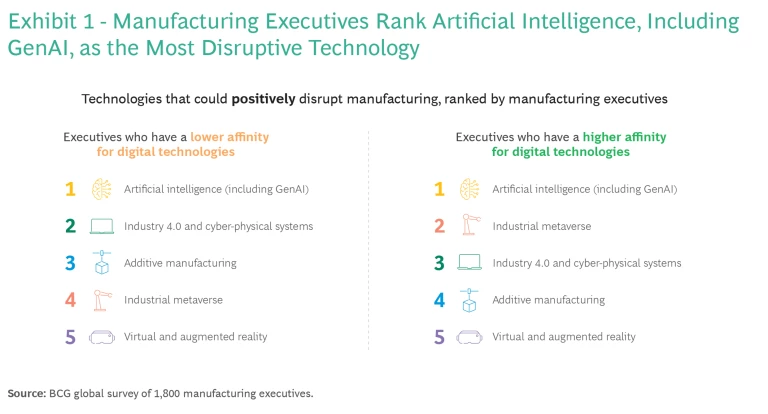
For one automotive supplier, deployment of AI resulted in a 21% boost in productivity, with ROI observed between one to three years across various applications. One application was an AI-powered scrap adviser that provided operators with optimized parameters, cutting scrap rates by 25%. Another was a pump health monitor that nearly eliminated breakdowns of a critical production pump, enhancing overall equipment effectiveness by more than seven percentage points. A third application involved implementing an AI-driven visual quality inspection system that detected aesthetic defects in products. This system reduced the need for quality control staff by 65% while improving the accuracy of the inspections.
AI tools have a variety of technological underpinnings and applications. Traditional AI applications, built on machine learning (ML) and deep learning (DL), have gained traction in recent years, primarily to facilitate data analysis, classification, clustering, and ranking. These capabilities support tasks such as anomaly detection and pattern identification. In contrast, the groundbreaking aspect of GenAI tools like ChatGPT is their capacity to create various types of new content—such as text, code, and images—in response to prompts in multiple formats.
How GenAI Helps Pave the Path to the Factory of the Future
Although GenAI introduces a range of innovative features, it is not ideally suited for tasks such as anomaly detection, production analytics, or setpoint optimization. For these tasks, the traditional AI that has been available in recent years is better equipped.
Nonetheless, GenAI has a complementary role that will significantly aid manufacturers’ efforts to realize the factory of the future . Its unique capabilities can enable manufacturers to automate and enhance factory activities and support their workforce in novel ways. Manufacturers have been using traditional AI to support transparency, predictability, and self-controlled systems, which are the key characteristics and maturity phases of this envisioned factory. (See “Three Levels of Digital Maturity in the Factory.”) Now GenAI’s content-creation capabilities enable three types of use cases in manufacturing—assistance systems, recommendation systems, and autonomous systems—that correspond to these maturity levels. (See Exhibit 2.)
Three Levels of Digital Maturity in the Factory
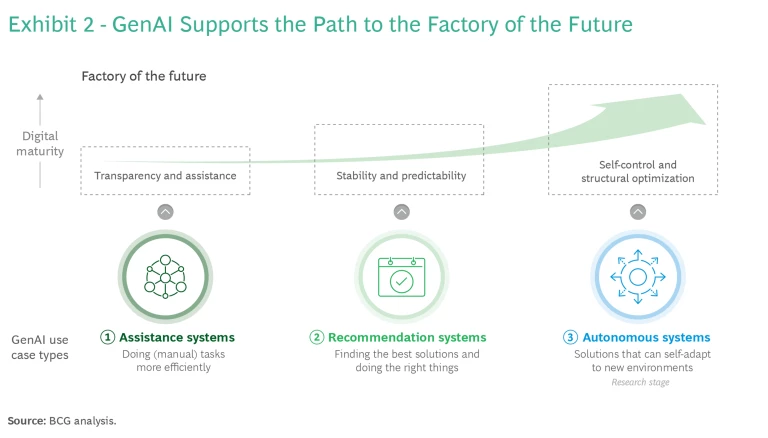
Assistance Systems. GenAI applications in this category raise the efficiency of hands-on tasks such as programming or machine maintenance. For example, automation engineers traditionally had to manually program and code automation solutions for machines and programmable logic controllers (PLCs). But a GenAI tool, akin to ChatGPT, can use text inputs to automatically generate code or code blocks. This reduces the time and effort required for automation engineering, thereby decreasing the related expenses. An engineer would need to focus only on reviewing, adjusting, and finalizing the code.
GenAI’s content-creation capabilities enable three types of use cases—assistance systems, recommendation systems, and autonomous systems—that correspond to maturity levels in the factory of the future.
A similar, yet even more powerful, impact is achieved by using GenAI to codify the knowledge and experience of workers who have decades of factory experience but may lack skills in modeling and analytics. GenAI assists in transforming workers’ experience-based intuition and know-how into data-driven, validated recommendations . For instance, it can validate a worker’s insights on adjusting machine parameters or resolving production anomalies by building a model in a programming language such as Python to derive such insights with data and analytics. This model is then available to assist with future problem solving and analysis.
Recommendation Systems. GenAI tools can provide recommendations that help workers to identify the best methods for specific tasks. An improved approach to predictive maintenance provides a prime illustration of how GenAI tools will complement traditional ML/DL-based AI. In the past, manufacturers sought to prevent breakdowns by performing maintenance according to fixed cycles or periods, or they made repairs when breakdowns occurred. With the advent of ML/DL-based AI, manufacturers were able to use data from different types of sensors to identify patterns, predict breakdowns, and then proactively conduct maintenance. GenAI enhances this predictive maintenance approach by automatically creating text or images that provide step-by-step instructions, including lists of required spare parts. Such a system allows the maintenance staff to spend more time performing tasks instead of preparing instructions—enhancing productivity and reducing costs. Even inexperienced technicians would be able to repair or maintain equipment more effectively with support from a GenAI tool.
GenAI assists in transforming workers’ experience-based intuition and know-how into data-driven, validated recommendations.
Autonomous Systems. The most advanced systems use GenAI’s abilty to attain self-regulation capabilities and to adapt to unfamiliar situations Developers are currently exploring GenAI solutions that would allow machinery to self-adapt to, for example, new environments. Consider the autonomous deployment of robots. Today, operators must manually execute certain material handling activities, especially nonrepetitive processes, because automating such activities is very difficult and requires intensive effort. Looking ahead, GenAI, in combination with real-world robotics datasets, will enable multimodal robots to translate operators’ language prompts (for instance, “Bring me spare part 47/11”) into a sequence of actions that the system then executes to perform material-handling tasks. This advance would reduce the need for task- and environment-specific training, data labeling, and frequent retraining. It thus has the potential to reduce engineering expenses, replace manual activities, and boost productivity. Another example is the generation of synthetic training data for AI-based systems, such as quality control using computer vision. This application expedites the ramping up of the systems by eliminating the need to collect real training data during production.
Deploying the Right Technology Foundation
To successfully implement and scale AI in manufacturing, it is not enough to pinpoint value-added applications. It is also imperative to set up the right foundations with respect to both people and technology. The people-related requirements for GenAI deployment, with respect to building the capabilities needed to develop and operate AI-based applications, are similar to those required for traditional AI deployments. However, the technology foundation is more complex for GenAI, making it crucial to emphasize the technology infrastructure and operating model.
The typical technology infrastructure for the scalable use of AI in manufacturing consists of six building blocks: data sourcing, data processing, GenAI applications, computing, connectivity, and cybersecurity. GenAI affects the requirements for several of these. (See “The Building Blocks of the Technology Infrastructure.”)
The Building Blocks of the Technology Infrastructure
Data Sourcing. Because AI tools are fundamentally data-dependent, manufacturers need to have the requisite sources. Data comes from three layers:
- Field Layer. Sensors, PLCs, and internet-of-things (IoT) devices.
- Control Layer. Manufacturing execution system (MES) and supervisory control and data acquisition (SCADA) systems.
- Transactional Layer. For example, the enterprise resource planning (ERP) system.
GenAI Applications. Within AI applications, the intelligence layer incorporates algorithms and AI models that adhere to “MLOps” standards covering model development, testing, validation, deployment, and servicing. The engagement layer has general and specific applications that allow AI users to engage with insights. Given GenAI applications’ unique features, additional tuning might be needed for foundational models to communicate in an organization’s “language.” Independent programs, called “agents,” are also necessary to coordinate different systems and requests.
Computing. Because AI applications often handle vast data volumes, they require significant computing power. The efficacy of AI tools largely depends on the processors—primarily central processing units (CPUs) and graphics processing units (GPUs)—which can be on-premises or cloud-based. GenAI tools typically demand more memory bandwidth and power, therefore requiring multiple GPUs for timely query responses.
Connectivity. Effective networking is crucial since many AI algorithms rely on near-real-time communication and data availability. Achieving this requires interplant cloud-based connectivity, intraplant wired or wireless communication, and inter-asset communication via suitable IoT protocols.
Cybersecurity. AI processes a large volume of often-sensitive data. A robust cybersecurity strategy is imperative, encompassing identity and access management, data protection, detection and response, risk analysis, and recovery planning. Because GenAI creates content, it is crucial to ensure that users can access only information they are permitted to receive. To restrict information access and enable customized responses, companies must implement security guardrail management beyond the basic data protection capabilities.
The greater complexity of the technology foundation required to efficiently apply GenAI means that different technology operating models are possible. These operating models can be distinguished primarily across three dimensions:
- Model Source. Manufacturers have three primary options for sourcing the large language model (LLM) required for a GenAI application. The first is to use a model hosted by a vendor, which they can fine-tune to better cater to their specific needs. Alternatively, they can use an open-source foundational model, which can also be fine-tuned if required. The third route, for those seeking greater control and customization, is to develop their own LLM.
- Platform and Infrastructure Setup. Again, manufacturers can choose from three main setups. One option is a shared cloud-based solution, offering multi-tenancy. Another is a single-tenancy, cloud-based solution with a private environment on the cloud, ensuring a more secure environment. The third option, for organizations that prioritize data sovereignty and tighter control over their infrastructure, is an on-premises solution.
- Application Operation. Manufacturers can operate a GenAI application on their own infrastructure. Alternatively, they can select a provider to host and operate the application.
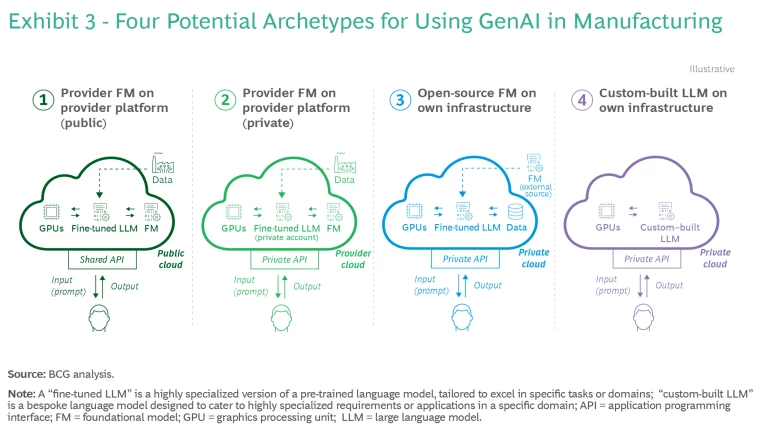
The feasible combinations of these options yield various archetypes for GenAI applications in the manufacturing environment. Exhibit 3 illustrates four potential archetypes.
Opting for a fully provider-based solution is the most straightforward method for implementing GenAI applications in manufacturing. Alternative methods include using an open-source model with optional fine-tuning or building a tailored solution in-house and operating it on the company’s infrastructure. The latter approaches offer greater customization flexibility and reduce data risks. On the downside, they demand substantial investment in infrastructure, such as for graphics processing units (GPUs) that perform calculations for AI models. Moreover, they require a wide array of expertise and capabilities to design, operate, and maintain in-house applications.
When solely considering infrastructure costs and amortization over time, the breakeven point for alternatives to a fully provider-based solution depends on numerous parameters. These include the input/output query length (measured in tokens), the acceptable response time for a query, and the distribution of queries throughout a shift (which determines the possibility of query batching). Achieving this breakeven typically requires several thousand queries per day.
A BCG analysis considered the running costs per query for a GenAI solution with approximately 20,000 queries per shift that is operated on-premises using the company’s own GPUs and servers. It found that the costs are twice that of a provider-based solution and that breaking even would require more than 60,000 queries per shift. The complexity and cost might explain why the majority of GenAI applications and pilot projects in manufacturing today lean toward a provider-based approach, capitalizing on the provider’s economies of scale. Nevertheless, the specific configuration for GenAI applications should be assessed on a case-by-case basis for each organization, as needs and situations vary widely.
How to Succeed with GenAI in Manufacturing
Effective engagement with GenAI requires developing a basic understanding of the technology and its capabilities and limitations. This can be achieved through, for instance, immersion sessions and technology demonstrations. Companies can apply the knowledge gained to identify the right value-creating applications and technology infrastructure as well as derive a corresponding target vision and road map. But these are not the only enablers of successful adoption. As with traditional AI implementations, many organizations will need to devote significant attention to issues relating to people, such as capabilities, governance, and communication.
Considering the multifaceted nature of the effort, manufacturers should take five steps to integrate GenAI into their operations:
1. Diagnose. Analyze the status quo. This includes identifying existing value leakages and opportunities for GenAI applications as well as people- and technology-related gaps.
2. Design. Determine the target picture, strategy, and road map. For example, evaluate and prioritize the most beneficial GenAI applications and determine the people- and tech-related measures to close existing gaps (such as capability and upskilling programs and make-or-buy decisions). To select the right GenAI model to support the prioritized applications, consider latency, accuracy, and operating cost. Given the importance of latency in technical systems, it is critical to balance this criterion against accuracy and cost.
3. Engineer. Develop GenAI solutions and related measures. This could entail assessing potential solution providers and tech vendors, developing your own applications, building technology infrastructure, and creating programs for internal capability building.
4. Implement. Test pilot applications for GenAI solutions and related people and technology measures such as implementing pilots to achieve early results and validate impact or creating momentum to foster the broader adoption of GenAI in the organization.
5. Scale. Roll out proven solutions and initiatives across production sites. At the same time, broaden the portfolio of GenAI use cases by launching additional pilot projects.
GenAI holds significant potential for manufacturing, and select companies are already tapping into its capabilities. However, it will not deliver across-the-board benefits; for certain digital applications in the factory of the future, GenAI is less suitable or offers a less favorable ROI than traditional ML/DL-based AI, which includes creating transparency through data manipulation and visualization, detecting anomalies, analyzing production, optimizing setpoints, and forecasting. Consequently, manufacturers will need to employ both GenAI and traditional AI to optimize their factory operations. Given the complexity, establishing the appropriate technology infrastructure and operating model is critical for success. Manufacturers that identify the opportunities and master the challenges of complementary implementation will stand to gain the most from deploying GenAI in the factory of the future.