Open AI launched ChatGPT less than a year ago, and already companies in every industry are exploring how generative AI can augment the capabilities of their customer care centers.
The large language models (LLMs) upon which ChatGPT and other text-based generative AI applications are built give these apps the power to respond to prompts with human-like text and voice, answering complex questions with seeming ease. The general public has quickly begun testing generative AI’s capabilities, and the technology is rapidly gaining acceptance, lauded for the variety and nature of the responses it provides.
This makes it a natural for customer service operations; indeed, we estimate that the technology, once implemented at scale, could increase productivity by 30% to 50%—or more. And according to a 2022 BCG survey of global customer service leaders, 95% expect their customers to be served by an AI bot at some point in their customer service interactions within the next three years.
Yet questions and challenges remain. LLM-based chatbots are trained on data that may contain intrinsic biases and can generate inaccuracies, a real problem in corporate contexts when an occasional error can result in significant costs to a company’s bottom line and reputation. This explains, in part, why all the current full-scale deployments of generative AI in a customer service setting have some level of human oversight or provide noncritical services, such as offering vacation ideas on travel websites.
But that will change, and soon. Companies looking to incorporate this powerful new technology into their customer care functions need to determine which use cases will deliver the most value and the steps to take to set themselves up for success while avoiding pitfalls. Here, we analyze both the promise and the challenges of generative AI and offer a path forward for companies eager to advance their customer service functions.
The Promise
The contact center—the hub of most customer service operations—has come a long way in the past couple of decades. Tools such as interactive voice response (IVR), agent assist, robotic process automation, and chatbots have already made customer service agents more productive. These technologies have not triggered a significant decline in the number of agents at most contact centers, largely because, as their old tasks were automated, contact center workers took on new roles and responsibilities, such as educating customers on how to use digital services.
LLMs have the power to significantly expand what can be automated.
But LLMs have the power to significantly expand what can be automated, performing critical customer service tasks that are far beyond the capacities of earlier technologies. These models are trained on vast amounts of data and can recognize, classify, and create sophisticated text and speech with speed and precision.
Driven by a host of enablers—new AI algorithms, increases in computing power, and cheaper cloud-computing infrastructure—emerging AI-powered customer care applications will be able to provide answers and solutions to customers faster and in a much more human-like manner. And when the data on which they are trained is adjusted, these algorithms can be fine-tuned to better suit specific industry and company needs.
Companies are already putting LLMs to work in their customer care centers. For example, this year Octopus Energy, a global specialist in sustainable energy, added generative AI capabilities to its customer service platform to help teams draft rich and thorough email responses more quickly than was previously possible. According to the company, emails drafted by the AI application achieved 18% higher customer happiness scores compared with email responses generated by humans alone. The application already responds to a third of all customer inquiry emails, creating capacity for agents to support more complex, high-growth products like electric vehicles and home electricity generation.
The Path to Maturity
Over time—and likely quite quickly—generative AI will become more and more embedded in the customer service function. (See Exhibit 1.)
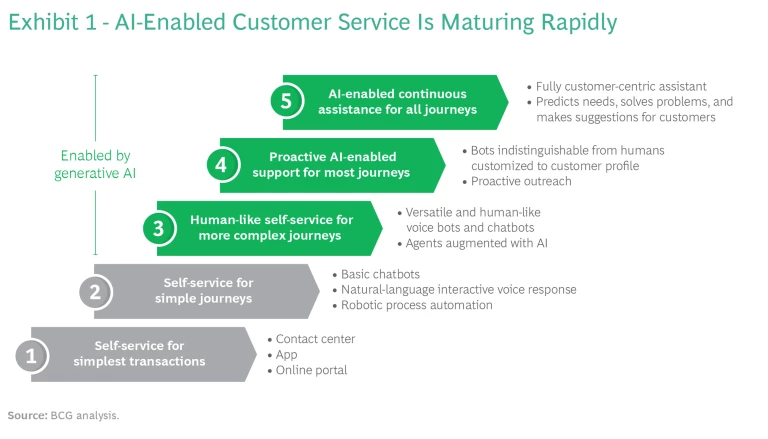
It will soon reach stage 3 of the journey we outline, driving predominantly reactive use cases that will continue to include humans in the loop. It will be able to resolve increasingly complex customer queries. This capability, along with the ability to interact with customers just like a human agent in both tone of voice and responsiveness, will continue to improve the customer experience.
At stage 4, AI will be able to assist customers with most of their queries. Businesses will transition from reacting to queries to proactively solving problems, thereby improving the customer experience even more. AI-enabled assistants will reach out directly to customers, offering preventive solutions to common problems rather than responding to queries after the problem occurs. Traditional AI and predictive analytics will decide on the prompts and the messages to deliver to the customer while generative AI will deliver those prompts and messages in a nonintrusive, human-like, and personalized manner. As confidence in AI-enabled customer contact grows and trained actions become more accurate and bias-free, it will require far less human oversight.
Eventually, at stage 5, AI-enabled support will be available for virtually every user journey. Generative AI could support service bots customized to the specific needs of individual customers, acting as a personal assistant that fully understands customers’ relationship with the company, anticipating their needs and concerns, and interacting with other systems elsewhere in the company to develop a full picture of the customer life cycle.
Companies have yet to proceed through all of these stages, but many are already imagining how a fully AI-enabled customer care center might work.
Companies are already imagining how a fully AI-enabled customer care center might work.
Overcoming the Challenges
Despite its promise, generative AI has several well-known limitations.
Among the most significant are the occasional factual inaccuracies they might provide. This is a particular danger in the context of customer care, where answers to customers’ questions, offered with a high degree of confidence, might be wrong. Moreover, LLM-based applications can incorporate biases inherent in the data on which the foundational model was trained and in the fine-tuning of models based on specific contexts; such biases could lead to unfair treatment of certain customers. A further risk: the technology could reveal proprietary information and intellectual property or inappropriate customer data.
Currently, the most effective strategy for minimizing these risks is to keep human agents in the loop, checking the content produced by AI before it reaches the customer. Whether to do so will likely depend on the type of customer interaction. Some interactions could be carried out by LLMs independently; other, high-value, premium services will likely require direct human oversight. Some companies are trying to reduce the risk of error by building hybrid tools that use a mix of LLMs and more traditional AI and automation technology to combine the precision of traditional tools with the human-like intimacy of LLMs.
As customer-service applications based on generative AI become more mature, companies will gain confidence in their performance, reducing the need for human oversight and allowing customers to interact with them directly. This will depend on the relevance, quality, and security of the training data; the ability to eliminate inaccuracies and bias; and the specific use case to which the AI is applied. Only when companies have fully understood and managed the risks and limitations of each use case should they move to the next level. They must also work carefully to ensure that as the technology develops, it maintains the “human touch” and capacity for empathy needed to be effective in the customer care context, regardless of the maturity level attained. Finally, they will soon have to understand the implications of their customers’ own increasing AI maturity . (See the sidebar, “Rise of the [Negotiation] Machines.”)
Rise of the (Negotiation) Machines
Rise of the (Negotiation) Machines
Imagine an AI-powered negotiation bot that uses its scale across thousands of interactions to infer and manipulate the pricing strategy of all competing companies in an industry. Companies will need to put in place safeguards and controls to avoid fraudulent activity and unfair preferential treatment—and even potentially devise ways to interact with AI-powered customers and companies differently.
Decisions, Decisions
In light of generative AI’s benefits, companies are moving to incorporate the technology into customer care at unprecedented speed, despite its risks and challenges.
But how to proceed? That is the critical question. Should companies buy an industry-specific ready-to-use solution or a system from one of the major tech companies offering platforms that incorporate LLM capabilities? Or should they invest time and resources in fine-tuning their own model?
To find the answers to these questions, companies should start now with some relatively simple but high-value use cases that will allow them to test the technology and learn what works and what doesn’t from technical, functional, and business perspectives. Exhibit 2 lays out the variety of use cases across the typical customer service journey—from initial customer contact to final response and resolution—that will likely be augmented by generative AI.
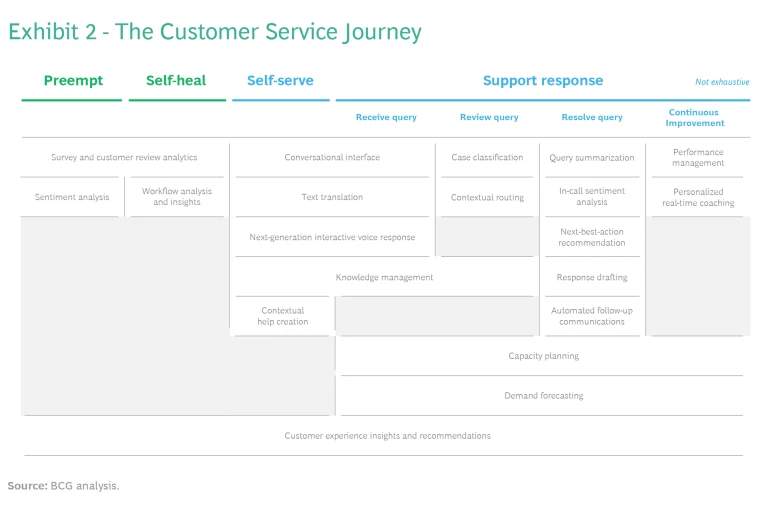
That’s the approach JetBlue has taken. The US-based airline has partnered with ASAPP, a technology vendor, to implement a packaged generative AI–enabled solution to drive the automation and augmentation of its chat channel, helping its contact center provide customer service. As a result, the contact center has been able to save an average of 280 seconds per chat—which yields a total of 73,000 hours of agent time saved in a single quarter and means that agents have more time to serve customers with complex problems.
Meanwhile, a North American technology company is progressively deploying a generative AI “sidekick” that helps customers and support engineers complete technical support requests, gain access to product information, and automate routine tasks. The initial version of the tool will provide support on the relatively simple requests that make up about 30% of total support tickets, such as how-to guides and basic product configuration information. As the technology matures, the company hopes to expand the range of use cases to cover more complex requests such as fault finding and fixing.
The decision to buy either an off-the-shelf customer-service application based on generative AI and use it without fine-tuning or a foundational LLM and fine-tune it based on an individual organization’s data depends on the complexity of the use case and the industry context.
For general-purpose, nonspecific use cases, fine-tuning is unnecessary; it’s a complex task that doesn’t always ensure greater accuracy and usually requires a major investment in time, money, and technical expertise.
At present, the most suitable approach includes building a customer-facing application based on a combination of traditional AI, such as machine-learning systems, LLMs, and prompt engineering. This final element involves developing and optimizing the information and constraints provided to LLMs to improve the accuracy of the answers—defining company-specific keywords within the prompt itself, for example. This process allows companies to achieve better levels of control, moderation, and personalization.
But in highly regulated industries and in those with heightened data security requirements such as financial services and defense —and generally for more complex and personalized use cases—fine-tuning of existing models will become a more popular option to ensure faster response times to more complex customer requests with greater control of the output.
Subscribe to our Artificial Intelligence E-Alert.
The recent rapid advances in generative AI are already transforming the ways in which companies manage their critical customer service functions. Now, companies must anticipate how the technology’s considerable capabilities could even more profoundly disrupt their business models.
We predict that today’s large customer service functions—which now typically interact with customers separately from the rest of the business—will become nimble, data-driven organizations that work closely with the rest of the business to create truly differentiating customer experiences. As generative AI systems learn more about a company’s products, operations, and customers, they will likely be able to predict customer behavior and reach out to customers in anticipation of their needs and desires.
As generative AI advances, it may also learn to use such information to reach deeper into other aspects of the business, such as production and resource planning and even working directly with suppliers.
Whatever happens, it will happen fast. Are you ready?
The authors thank Juan Martin Maglione, Marcus Wittig, and Stuart McCann for their contributions to this article.