Generative AI will be a powerful enabler of competitive advantage for companies that crack the code of adoption. In a first-of-its-kind scientific experiment, we found that when GenAI is used in the right way, and for the right tasks, its capabilities are such that people’s efforts to improve the quality of its output can backfire. But it isn’t obvious when the new technology is (or is not) a good fit, and the persuasive abilities of the tool make it hard to spot a mismatch. This can have serious consequences: When it is used in the wrong way, for the wrong tasks, generative AI can cause significant value destruction.
We conducted our experiment with the support of a group of scholars from Harvard Business School, MIT Sloan School of Management, the Wharton School at the University of Pennsylvania, and the University of
The opportunity to boost performance is astonishing: When using generative AI (in our experiment, OpenAI’s GPT-4) for creative product innovation, a task involving ideation and content creation, around 90% of our participants improved their performance. What’s more, they converged on a level of performance that was 40% higher than that of those working on the same task without GPT-4. People best captured this upside when they did not attempt to improve the output that the technology generated.
Creative ideation sits firmly within GenAI’s current frontier of competence. When our participants used the technology for business problem solving, a capability outside this frontier, they performed 23% worse than those doing the task without GPT-4. And even participants who were warned about the possibility of wrong answers from the tool did not challenge its output.
When using GPT-4 for a task within the tool’s current frontier of competence, nearly all participants improved their performance. Those who used GPT-4 for a task outside this frontier performed worse than those who didn’t use the tool.
Our findings describe a paradox: People seem to mistrust the technology in areas where it can contribute massive value and to trust it too much in areas where the technology isn’t competent. This is concerning on its own. But we also found that even if organizations change these behaviors, leaders must watch for other potential pitfalls: Our study shows that the technology’s relatively uniform output can reduce a group’s diversity of thought by 41%.
The precise magnitude of the effects we uncovered will be different in other settings. But our findings point to a crucial decision-making moment for leaders across industries. They need to think critically about the work their organization does and which tasks can benefit from or be damaged by generative AI. They need to approach its adoption as a change management effort spanning data infrastructure, rigorous testing and experimentation, and an overhaul of existing talent strategies. Perhaps most important, leaders need to continually revisit their decisions as the frontier of GenAI’s competence advances.
The Value at Stake
Our findings make clear that generative AI adoption is a double-edged sword. In our experiment, participants using GPT-4 for creative product innovation outperformed the control group (those who completed the task without using GPT-4) by 40%. But for business problem solving, using GPT-4 resulted in performance that was 23% lower than that of the control group. (See Exhibit 1.)
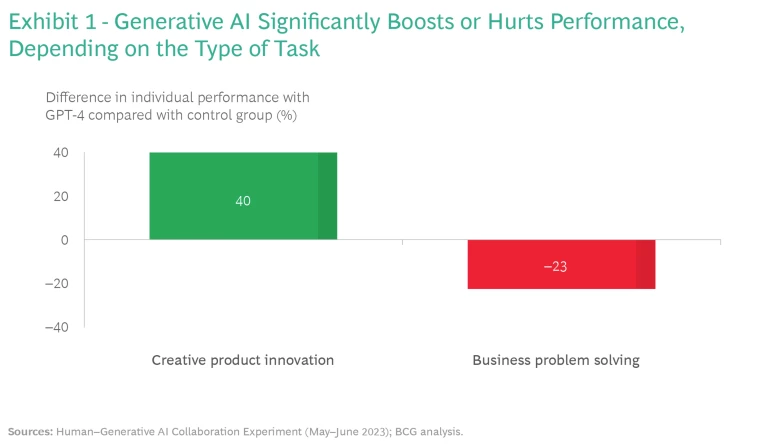
The creative product innovation task asked participants to come up with ideas for new products and go-to-market plans. The business problem-solving task asked participants to identify the root cause of a company’s challenges based on performance data and interviews with executives. (See “Our Experiment Design and Methodology.”) Perhaps somewhat counterintuitively, current GenAI models tend to do better on the first type of task; it is easier for LLMs to come up with creative, novel, or useful ideas based on the vast amounts of data on which they have been trained. Where there’s more room for error is when LLMs are asked to weigh nuanced qualitative and quantitative data to answer a complex question. Given this shortcoming, we as researchers knew that GPT-4 was likely to mislead participants if they relied completely on the tool, and not also on their own judgment, to arrive at the solution to the business problem-solving task (this task had a “right” answer).
Our Experiment Design and Methodology
Task Design
Our experiment was designed around two sets of tasks, each completed by a separate group of participants.
The first set focused on creative product innovation. Participants were asked to brainstorm ideas for new products to solve an unmet need, develop the business case for each, create testing and launch plans, and write memos to persuade others to adopt the idea. The following are some of the questions that participants had to answer:
- You are working for a footwear company in the unit developing new products. Generate ideas for a new shoe aimed at a specific market or sport that is underserved. Be creative and give at least ten ideas.
- Come up with a list of steps needed to launch the product. Be concise but comprehensive.
- Use your best knowledge to segment the footwear market by users. Develop a marketing slogan for each segment you are targeting.
- Suggest three ways of testing whether your marketing slogan works well with the customers you have identified.
- Write marketing copy for a press release of the product.
- The CEO, Harold Van Muylders, of Kleding (a fictitious company) would like to understand the performance of the company’s three brands (Kleding Man, Kleding Woman, and Kleding Kids) to uncover deeper issues. Please find attached interviews from company insiders. In addition, the attached excel sheet provides financial data broken down by brands.
- Using this information, if the CEO must pick one brand to focus on and invest in to drive revenue growth in the company, what brand should that be? What is the rationale for this choice? Please support your views with data and/or interview quotations.
The creative product innovation task was designed to play to GPT-4’s strengths as an LLM, primarily because it involved creativity, refinement, and persuasive writing, which are within GPT-4’s frontier of capability. The business problem-solving task was explicitly designed to be difficult for GPT-4 to complete. This task, which contains a clear right answer, was designed to be complex enough to ensure that GPT-4’s answer on a first pass would be incorrect. Participants could solve the business problem-solving task either by relying on their own judgment to tease out the nuances in the questions and data provided or by prompting GPT-4 to better “think through” the problem.
Measuring Baseline Proficiency
Before attempting the experimental task, each participant also solved a baseline task without the use of any AI tool. This task was designed to be very similar to the experimental task in terms of difficulty and the skills it tested for.
By evaluating performance on this baseline task using the same grading rubric as the experimental task (see below), we were able to create a sense of each participant’s baseline proficiency in the specific task type. This then enabled us to understand how GPT-4 use affected relative performance across individuals with different levels of baseline proficiency.
Grading Rubric
Each set of tasks had its own grading rubric:
For creative product innovation, participants were graded on a scale of 1 to 10, on four dimensions: creativity, persuasive writing, analytical thinking, and overall writing skills. Overall performance was calculated as the average of the four dimensions.
For business problem solving, participants were graded on the correctness of the response (that is, which channel or brand is most likely to boost revenue or profitability for the fictitious company). Performance was assessed as a binary grade (correct or incorrect).
Experimental Treatment Design
Each of the 758 participants in the experiment was randomly assigned to one of the two sets of tasks (creative product innovation or business problem solving), controlling for key demographic variables. Within each set of tasks, participants were then randomized into three groups:
- Group A: Those who used GPT-4 to solve the task after a 30-minute training on best practices on GPT-4 use (see the sidebar on training).
- Group B: Those who used GPT-4 to solve the task without any training.
- Group C: Those who did not use GPT-4 to solve the task (control group).
Incentive Structure
A cornerstone of this experiment is its proximity to real-world tasks performed by business professionals. For this experiment to fully capture how participants may behave in the real world, a substantial incentive structure was put in place to ensure that participants would do their best to solve each task.
To ensure this, participation in this experiment was noted in participants’ bi-annual performance reviews. Successful completion of the experiment was tracked and ultimately factored into participants’ annual performance bonuses. In addition, top 20% performers were specifically called out to their managers to further incentivize high performance.
Grading Methodologies
For both the baseline and the experimental tasks, the output from participants was graded by humans (a combination of BCG consultants and business school students with experience grading academic assignments). The human graders were “blinded,” in that they did not know whether the output was from participants who used GPT-4 or not. Furthermore, grading assignments were made in such a way that grader-specific fixed effects (some graders are naturally harsher than others) were controlled for, ensuring that the results were not biased in that way.
We also used GPT-4 to independently grade performance on all tasks, using the same rubric as the human graders. Human-generated grades largely coincided with GPT-4 grades, leading to the same takeaways from the experiment. In line with standard academic practice, we primarily relied on human-generated grades for the analyses presented here, except for those that concern changes in distribution between baseline and experimental tasks. For the latter analyses in particular, we relied on the GPT-4 grades to maximize consistency across baseline and experimental tasks (in the human-generated grading system, different graders may have scored the baseline and experimental submissions for the same participant).
For a more detailed description of the experimental design, please see our scholarly paper on the topic.
We also knew that participants were capable of finding the answer to the business problem-solving task on their own: 85% of participants in the control group did so. Yet many participants who used GPT-4 for this task accepted the tool’s erroneous output at face value. It’s likely that GPT-4’s ability to generate persuasive content contributed to this result. In our informal conversations with participants, many confirmed that they found the rationale GPT-4 offered for its output very convincing (even though as an LLM, it came up with the rationale after the recommendation, rather than creating the recommendation on the basis of the rationale).
The double-edged-sword effect holds across all levels of baseline proficiency. (At the start of the experiment, participants completed a baseline task without using GPT-4 that we then graded and ranked; see the sidebar on our design and methodology). This has an important caveat: The lower the individual’s baseline proficiency, the more significant the effect tended to be; for the creative product innovation task, these individuals boosted performance by 43%. Still, the effect was material even for the top-ranked baseline performers, among whom the upside and downside of using GPT-4 on the two tasks were 17% and -17%, respectively. (See Exhibit 2.) (Throughout, our discussion of participants’ performance is not indicative of their absolute levels of competence and talents with respect to these or other tasks.)
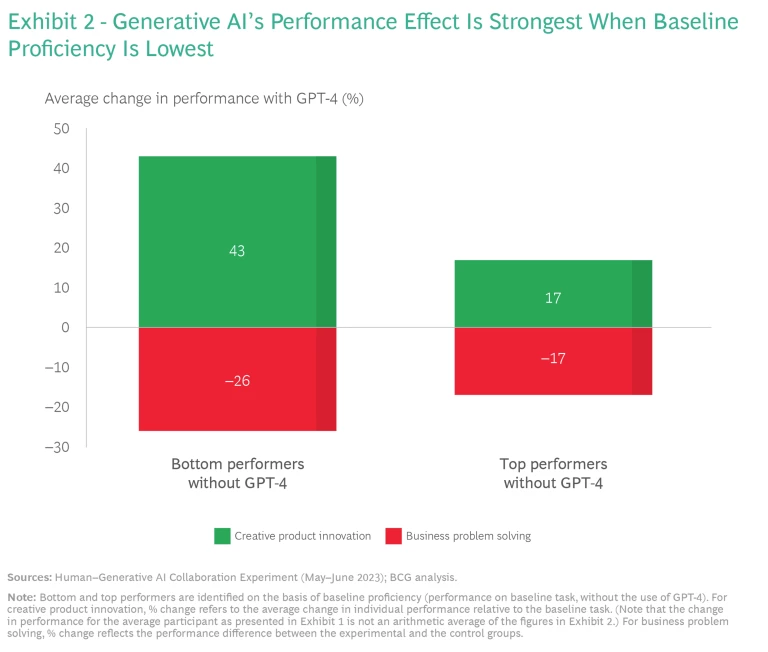
The strong connection between performance and the context in which generative AI is used raises an important question about training: Can the risk of value destruction be mitigated by helping people understand how well-suited the technology is for a given task? It would be rational to assume that if participants knew the limitations of GPT-4, they would know not to use it, or would use it differently, in those situations.
Our findings suggest that it may not be that simple. The negative effects of GPT-4 on the business problem-solving task did not disappear when subjects were given an overview of how to prompt GPT-4 and of the technology’s limitations. (See “Our Use of Training in the Experiment.”)
Our Use of Training in the Experiment
Participants in the business problem-solving task were informed of the challenges and pitfalls of using GPT-4 in a problem-solving context. They were shown an example of how GPT-4 can fail at reasoning and cautioned against relying heavily on GPT-4 for such tasks.
Even more puzzling, they did considerably worse on average than those who were not offered this simple training before using GPT-4 for the same task. (See Exhibit 3.) This result does not imply that all training is ineffective. But it has led us to consider whether this effect was the result of participants’ overconfidence in their own abilities to use GPT-4—precisely because they’d been trained.
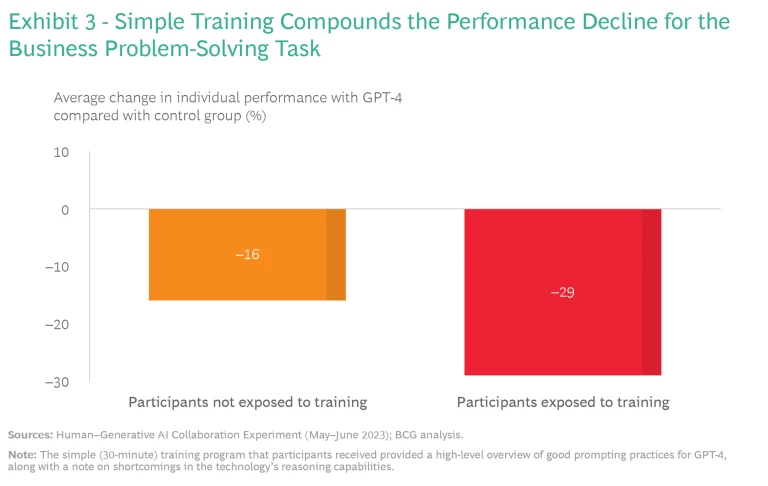
New Opportunities for Human Talent
Effects at the group level, like the ones discussed above, aren’t necessarily indicative of how generative AI impacts individuals. When we look behind the averages, we find that the use of GPT-4 has two distinct effects on individual performance distribution. (See Exhibit 4.) First, the entire distribution shifts to the right, toward higher levels of performance. This underscores the fact that the 40% performance boost discussed above is not a function of “positive” outliers. Nearly all participants (around 90%), irrespective of their baseline proficiency, produced higher-quality results when using GPT-4 for the creative product innovation task. Second, the variance in performance is dramatically reduced: A much higher share of our participants performed at or very close to the average level.
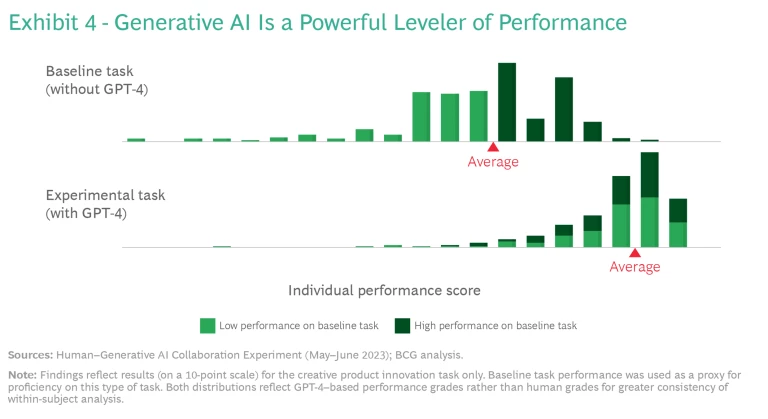
In other words, participants with lower baseline proficiency, when given access to generative AI, ended up nearly matching those with higher baseline proficiency. Being more proficient without the aid of technology doesn’t give one much of an edge when everyone can use GPT-4 to perform a creative product innovation task. (See Exhibit 5.) The fact that we observed this effect among our well-educated, high-achieving sample suggests that it may turn out to be even more pronounced in contexts that are more heterogenous, with a wider spread in proficiency.
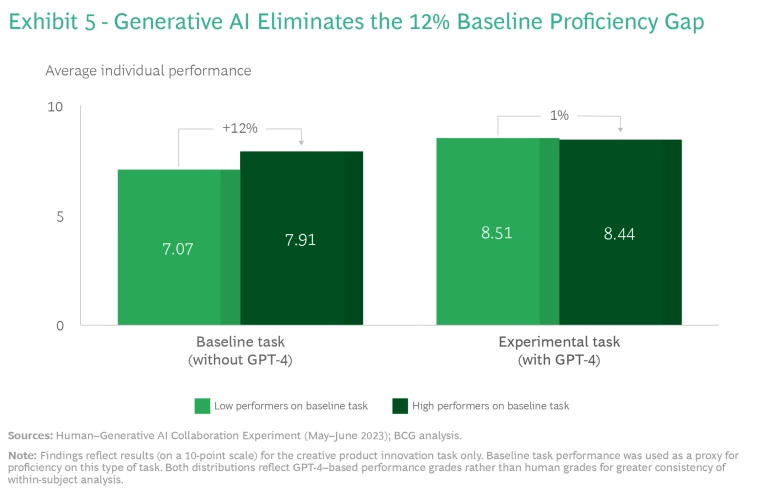
Digging deeper, we find that because GPT-4 reaches such a high level of performance on the creative product innovation task, it seems that the average person is not able to improve the technology’s output. In fact, human efforts to enhance GPT-4 outputs decrease quality. (See the sidebar on our design and methodology for a description of how we measured quality.) We found that “copy-pasting” GPT-4 output strongly correlated with performance: The more a participant’s final submission in the creative product innovation task departed from GPT-4’s draft, the more likely it was to lag in quality. (See Exhibit 6.) For every 10% increase in divergence from GPT-4’s draft, participants on average dropped in the quality ranking by around 17 percentile points.
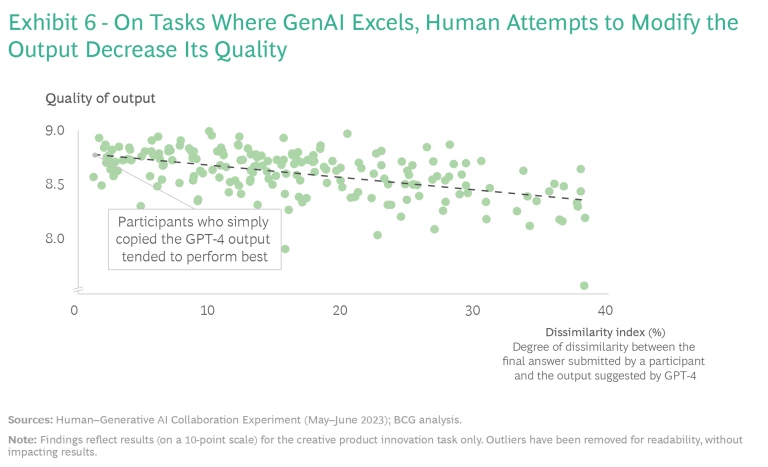
It appears that the primary locus of human-driven value creation lies not in enhancing generative AI where it is already great, but in focusing on tasks beyond the frontier of the technology’s core competencies.
Interestingly, we found that most of our participants seemed to grasp this point intuitively. In general, they did not feel threatened by generative AI; rather, they were excited by this change in their roles and embraced the idea of taking on tasks that only humans can do. As one participant observed, “I think there is a lot of value add in what we can do as humans. You need a human to adapt an answer to a business’s context; that process cannot be replaced by AI.” Another noted, “I think it’s an opportunity to do things more efficiently, to stop wasting time on things that are very repetitive and actually focus on what’s important, which is more strategic.”
However, it is worth keeping in mind the population of this study: highly skilled young knowledge workers who are more likely to be able to make this transition easily. Other professionals may feel greater fear or experience more difficulty adapting their role to the new technology.
The Creativity Trap
Even if you use GenAI in the right way, and for the right tasks, our research suggests that there are risks to creativity.
The first risk is a tradeoff between individual performance gains and collective creativity loss. Because GPT-4 provides responses with very similar meaning time and again to the same sorts of prompts, the output provided by participants who used the technology was individually better but collectively repetitive. The diversity of ideas among participants who used GPT-4 for the creative product innovation task was 41% lower compared with the group that did not use the technology. (See Exhibit 7.) People didn’t appreciably add to the diversity of ideas even when they edited GPT-4’s output.
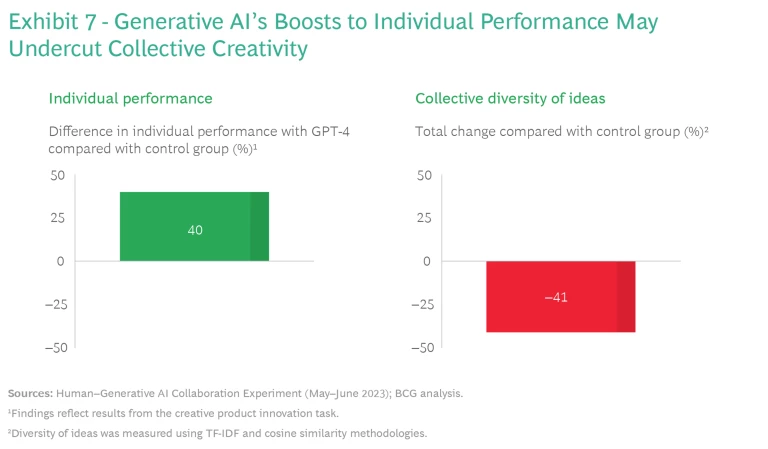
The second risk is drawn from a sample of our interviews with participants. Roughly 70% believe that extensive use of GPT-4 may stifle their creative abilities over time. (See Exhibit 8.) As one participant explained, “Like any technology, people can rely on it too much. GPS helped navigation immensely when it was first released, but today people can’t even drive without a GPS. As people rely on a technology too much, they lose abilities they once had.” Another participant noted, “This [phenomenon] is definitely a concern for me. If I become too reliant on GPT, it will weaken my creativity muscles. This already happened to me during the experiment.” Businesses will need to be mindful of their employees’ perceptions of and attitudes about generative AI , and how those might affect their ability to drive innovation and add value.
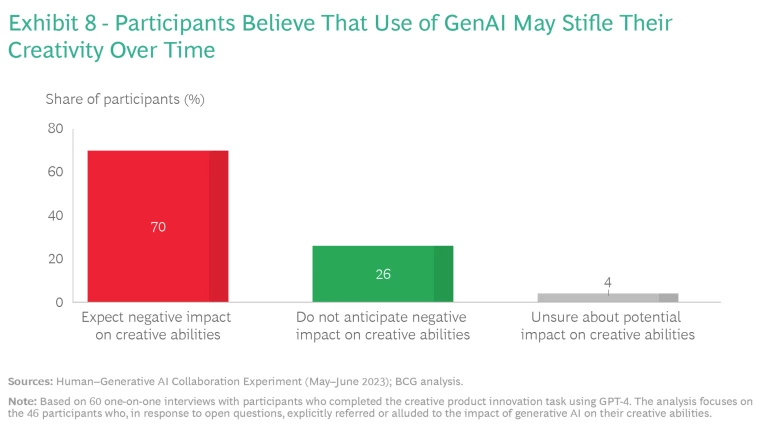
We don’t yet have data to confirm our participants’ perceptions; this is a topic for further study. But if employees’ concerns bear out, it could compound the group-level risk. Specifically, the loss of collective diversity of ideas may be exacerbated if employees experience some atrophy of individual creativity.
The Generative AI Change Imperative
Inspired by the findings from our research, we envision a series of questions, challenges, and options that can help business leaders make generative AI adoption a source of differentiation—and, as such, an enabler of sustained competitive advantage.
Data Strategy. Any company that incorporates GenAI can realize significant efficiency gains in areas where the technology is competent. But if multiple firms apply the technology across similar sets of tasks, it can produce a leveling effect among organizations analogous to the pattern observed among participants in our experiment. As a result, one of the keys to differentiation will be the ability to fine-tune generative AI models with large volumes of high-quality, firm-specific data.
This is easier said than done. In our experience, not all companies have the advanced data infrastructure capabilities needed to process their proprietary data. Developing these capabilities has been a key focus of AI transformations, but with the arrival of generative AI, it becomes all the more important: As we have argued elsewhere , the power of GenAI often lies in the identification of unexpected—even counterintuitive—patterns and correlations. To reap these benefits, companies need a comprehensive data pipeline, combined with a renewed focus on developing internal data engineering capabilities.
Roles and Workflows. For tasks that generative AI systems have mastered—which, of course, is an ever-expanding list—people need to radically revise their mindset and their approach to work. Instead of the default assumption that technology creates a helpful first draft that requires revision, people should regard the output as a plausible final draft that they should check against firm-established guardrails but otherwise largely leave as is.
The value at stake lies not only in the promise of greater efficiency but also in the possibility for people to redirect time, energy, and effort away from tasks that generative AI will take over. Employees will be able to double down on the tasks that remain beyond the frontier of this technology, reaching higher levels of proficiency.
The value at stake lies not only in the promise of greater efficiency but also in the possibility for people to redirect time, energy, and effort away from tasks that generative AI will take over.
Turning the lens on ourselves, we can already envision our employees spending less time manually summarizing research or polishing slides and instead investing even more effort in driving complex change management initiatives. The impact of generative AI’s disruption will of course vary dramatically across job categories. But at least some workers—including the majority of our participants—are confronting this prospect with optimism.
Strategic Workforce Planning. To get the AI–human dynamics right in complex organizations, leaders must grapple with four questions that have no easy answers:
- Which capabilities will you need? As with any other technology, it will take people to define what and how generative AI will be used. But it isn’t obvious which human capabilities are best suited to maximizing the tool’s value or how often these capabilities will change. We’re seeing this uncertainty play out in real time with respect to LLMs: The role of “prompt engineer” didn’t exist a year ago, but demand for this role during Q2 2023 was nearly seven times higher than it was in
Q1.2 2 BCG analysis based on global job postings in the Lightcast (formerly BurningGlass) platform through 8/24/2023. (GPT-4 was launched toward the end of Q1, on March 14, 2023.) And yet, prompt engineers may no longer be needed once generative AI itself has mastered the task of breaking down complex problems into optimal prompts (as it appears it soon will with autonomous agents). Even the selection of optimal LLMs for specific business applications, which is largely done by humans at present, may in the future be outsourced to AI systems themselves. - What is your hiring strategy? Because generative AI is a great leveler of proficiency on certain tasks, raw talent may not be a good predictor of high performance in a world of widespread GenAI use. For example, some people may have lower baseline proficiency for a type of task while being quite capable of partnering with generative AI to outperform peers. Finding these individuals will be an important goal for future talent strategies, but the underlying traits are not yet clearly identified.
How will you train people effectively? As our findings indicate, straightforward training won’t be sufficient. Effective training will likely need to explicitly address any cognitive biases that may lead people to over-rely on generative AI in situations where the technology has not yet reached the right level of competence.
We also see a potentially deeper issue: Even as certain tasks are fully handed over to GenAI, some degree of human oversight will be necessary. How can employees effectively manage the technology for tasks that they themselves have not learned how to do on their own?
- How will you cultivate diversity of thought? Our results suggest that GenAI detracts from collective creativity by limiting the range of perspectives that individuals bring to the table. This loss in diversity of thought may have ripple effects beyond what we can currently envision. One plausible risk is that it could shrink the long-term innovation capacity of organizations—for example, by making ideation more homogenous. It’s a slippery slope, as a decline in innovation capabilities means less differentiation from competitors, which could impede growth potential. The good news is that the ideas that humans generate on their own and the ideas that they generate when assisted by generative AI are vastly different. Setting aside the degree of diversity in each group, when we compared the output of the control and experimental groups, the overlap (semantic similarity) was less than 10%. The key for leaders will be to use both approaches to ideation—which ultimately will create an even wider circle of ideas.
Experimentation and Testing. Generative AI systems continue to develop at a stunning rate: In just the few months between the releases of OpenAI’s GPT-3.5 and GPT-4, the model made huge performance leaps across a wide range of tasks. Tasks for which generative AI is ill-suited today will likely fall within its frontier of competence soon—perhaps in the very near future. This is likely to happen as LLMs become multi-modal (going beyond text to include other formats of data), or as models grow larger, both of which increase the likelihood of unpredictable capabilities.
Given this lack of predictability, the only way to understand how generative AI will impact your business is to develop experimentation capabilities—to establish a “generative AI lab” of sorts that will enable you to keep pace with an expanding frontier. And as the technology changes, the collaboration model between humans and generative AI will have to change as well. Experimentation may yield some counterintuitive or even uncomfortable findings about your business, but it will also enable you to gain invaluable insights about how the technology can and should be used. We put our feet to the fire with this experiment—and we believe all business leaders should do the same.
Generative AI will likely change much of what we do and how we do it, and it will do so in ways that no one can anticipate. Success in the age of AI will largely depend on an organization’s ability to learn and change faster than it ever has before.
In addition to the collaborators from the academic team listed above, the authors would like to thank Clément Dumas, Gaurav Jha, Leonid Zhukov, Max Männig, and Maxime Courtaux for their helpful comments and suggestions. The authors would also like to thank Lebo Nthoiwa, Patrick Healy, Saud Almutairi, and Steven Randazzo for their efforts interviewing the experiment participants. The authors also thank all their BCG colleagues who volunteered to participate in this experiment.