Over the course of just a few months, generative AI (genAI) has produced unprecedented interest worldwide, particularly with OpenAI and Google’s conversational AI solutions, ChatGPT and Bard. The rapid adoption of these technologies, with ChatGPT amassing more than a million users in under a week, has set new benchmarks in the tech landscape. New genAI solutions are now being rolled out weekly as businesses rush to take advantage of an unavoidable tech revolution.
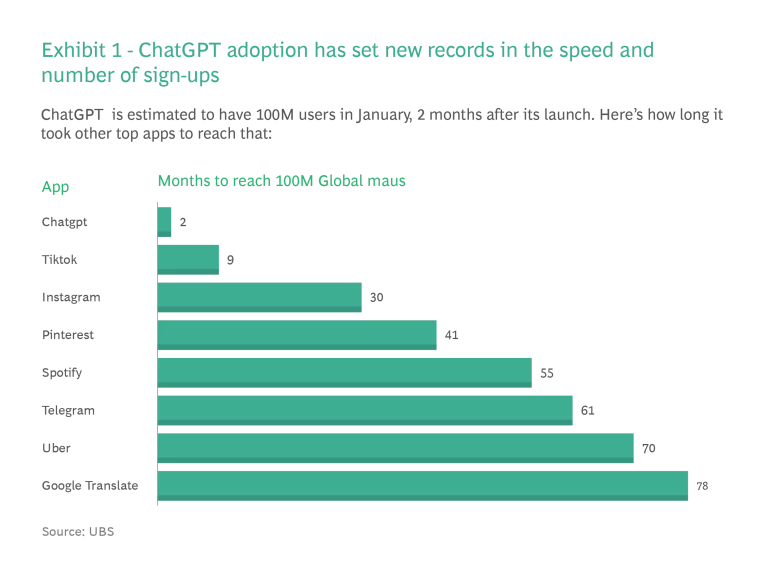
GenAI’s potential in areas such as conversational shopping, customer support, search, personalization, and knowledge synthesis is undeniable. However, the excitement generated by these new opportunities should not drown out an important fact: businesses must not abandon the fundamentals of great product design. At the end of the day, whether an organization is B2C or a B2B, humans are the ultimate end-users of its generative AI solution. And these solutions need to work for people to use them repeatedly. Retaining a focus on building holistic, user-centric genAI solutions is, therefore, critical to unlocking its value to customers.
Pillar #1: Emphasize user centricity
In BCG’s study of numerous genAI solutions, we have observed that while powerful, these solutions often lack user-centric design. Initial genAI solutions to book hotel rooms, order groceries or make table reservations, require users to install plug-ins or add keys to chat-based AI systems, creating an additional layer of complexity to the new way of doing things. If genAI solutions are not easy to use, users will default to the solutions they know, such as search engines or service apps. To develop a product that stands the test of time, it must deliver value to users, solving their problems efficiently and intuitively.
Key points to remember when building genAI applications and services are to start with a strong strategy, incorporate human-centric design, build iteratively, and make solutions easy to access and return to.
Set a strategy: Begin by defining the organizational objectives for your genAI solutions. Whether your goals are centered around marketing value, experience improvement, data generation, or financial metrics, it is vital to connect these objectives to the aspirations and pain points of your users. Getting to the core of your opportunity areas lies in active listening and user research to define requirements. Your genAI solution is only as successful as its ability to solve real problems.
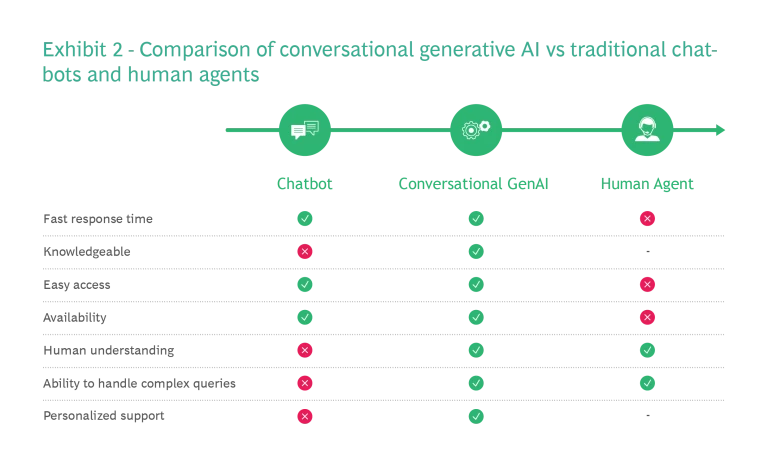
Testing and feedback: Establish tight feedback loops and conduct user tests to gather insights for iteration and optimization. Ethnography and prototype testing can provide valuable insights on user perspectives, such as:
- User lens: Ethnographic research offers the ability to look at a product from the point of view of a user, and not just as a technology solution.
- User experience: Collect feedback on UX, and in the case of conversational AI, how to strike the right conversational tone, number of questions to response ratio, chat lengths, and use of media formats.
- Hooks: A deeper understanding of what would get users to return to the solution, and double down on these value-adding features.
- Edge-cases: Identifying edge-cases and scenarios that have not been accounted for in the design process, which also provide the opportunity to troubleshoot new areas to set up guardrails around.
Build fast and adapt: Adopt an iterative approach to building and scaling genAI solutions, enabling rapid evaluation and the ability to course-correct. Given the rapid evolution of this nascent technology, organizing work into short sprints with lean teams ensures agility and adaptability.
Pillar #2: Rethink solution development in the GenAI era
Traditional approaches to solution design need to evolve from linear to open design principles when working with genAI solutions. In traditional digital solutions, the user experience and customer journey are defined by the product builders in a linear fashion. This logic has been applied to traditional chatbots and makes for a frustrating and limiting experience where the bot cannot handle unstructured requests.
However, common to many generative AI solutions is that their relationship to the user is non-linear. For human interactions (even with a bot) to feel natural, conversations need to be open and free, which challenges the traditional approach to building digital products. This is what conversational AI models like ChatGPT and Bard have succeeded at; they excel at natural interactions by mimicking human conversations and creating a sense of freedom. However, although this newfound freedom poses design challenges for building applications around genAI, genAI systems are still software systems: you still need build an integration and deployment pipeline for the engineering team, you still need to do QA and deploy services to a production environment. GenAI systems still need to be maintained once they are running live in production.
Based on our experience in developing, testing and building genAI-based solutions, here are some key recommendations:
Demonstrate value: Users may not be aware of the full capabilities of your genAI solution. Allow the AI to demonstrate its value by showcasing additional features beyond the user's initial request. These capabilities can be communicated in different formats, such as on the landing pages of the solution (e.g., ChatGPT in OpenAI), through disclaimers around the chat window, or throughout the chat with the AI highlighting, where relevant, its capabilities (as well as its limitations).
Time to value: Maintain user interest by delivering value early in an interaction. Traditional solutions rely on gathering detailed information through user inputs. For instance, when ordering food, an app needs data on location, cuisine, restaurant, specific food items to be ordered, delivery instructions, user details, credit card information etc. In contrast, genAI allows for more natural dialogue. Offer recommendations or useful information upfront and incrementally answer the final request through an interactive conversation. This concept of ‘earning the right to ask for information’ establishes a give-and-take dynamic between the user and the AI, creating a balance in the amount of information shared by and with the user. Doing so will help improve a crucially overlooked statistic; approximately 40% of users drop out of conversations with chatbots after the first text, with a further 25% leaving after the second.
AI-led interaction: GenAI holds the power to shift the interaction dynamic from being simply question and response based to be more proactive, by being AI-driven. Conversational AI models can ask follow-up questions based on predicted behavior patterns or the context of the conversation. This shift in interaction dynamic opens possibilities for deeper customer relationships and engagement, deeper customer understanding enabling businesses to align their offerings with customer objectives. A study of chatbots in the banking industry found that genAI-powered bots had 55% better engagement rates and that 72% of customers rated personalization as highly important.
Relationship and retention: GenAI has the potential to transform the way users interact with businesses and their solutions. Each chat session can be seen as a building block in a relationship, with every new session building upon previous ones to deepen the understanding of a user’s needs. This understanding is a key requirement to enable personalization which builds loyalty and retention in ways that were previously reserved only for personalization-focused tech solutions, such as Spotify and Netflix. Leveraging generative AI's memory capabilities, such as those seen in LangChain, creates a personalized experience, and strengthens information relevance and user loyalty. Increased usage enhances the recommendation engine, creating a higher switching cost for users who do not want to lose benefits accumulated as a result of having a conversation history.
Modular blocks: Conversational chat can be organized around modules and combined in various permutations to create unique user journeys. These conversational blocks may include (but are not limited to):
- User request: This request often initiates the relationship and opens a dialogue with your genAI solution. With this request, a user can take a conversation in any direction.
- Contextual analysis: Once your user’s request has been submitted, it is key for your genAI engine to understand the context and provide an appropriate response, while simultaneously identifying potential information gaps that need to be closed to optimize the response.
- AI-driven response/follow-up: This is your opportunity to showcase your genAI solution’s value. If the user has not provided enough context or information in their request, it is important to demonstrate value and balance the number of follow-ups with the time to value principle. This is also an opportunity to end each message with a call to action or an open-ended question to initiate a new conversation into an adjacent topic.
- User response and/or follow-up: This is where you receive proof that your AI-driven response has delivered (or shown promise to deliver) value to the user, and that the user is interested in continuing to engage with your solution.
- AI-driven unprompted chat initiation: Once you have established a relationship between the user and your conversational AI solution, make the most of your AI’s memory to follow-up and re-engage the user in a meaningful way. The importance is to strike the right balance between usefulness and spam.
- AI-driven closing: When the conversation comes to a natural end, your genAI solution can close the conversation by reminding the user that it is always available and ready to provide support, thereby conditioning the user to return.
The flexibility of modular design allows various permutations, creating a unique journey for every individual user, depending on context, conversation initiation, and topic of interest.
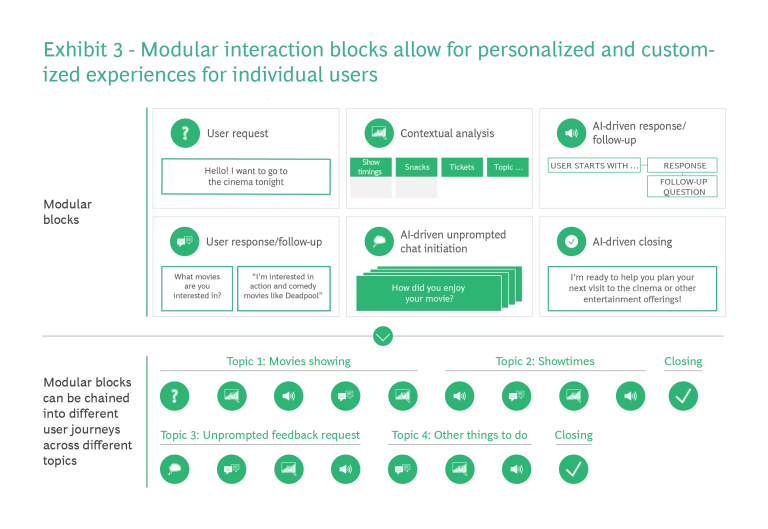
Touchpoints and access: GenAI solutions require a behavioral shift from users, similar to the early days of search engines when people had to adopt new habits to benefit from their potential. To ensure adoption and accessibility, businesses should consider leveraging existing touchpoints where their brand already exists, such as websites, apps, and social media channels, while also exploring new channels, such as virtual avatars, holograms, and robots, to expand reach and engagement. By exploiting the unique advantages of existing touchpoints, businesses and organizations can maximize the success of a genAI solution roll-out.
Pillar #3: Setting appropriate guardrails
The use of conversational AI solutions introduces new challenges in terms of user behavior and ethical considerations. Unlike traditional digital products with defined boundaries, generative AI empowers users to make requests that may not align with planned scenarios. This openness brings forth potential risks, both financial and reputational, that businesses must address.
Ensuring safe and responsible implementation of genAI is not just a product decision, but an organization-wide decision. Setting up appropriate guardrails is therefore a strategic decision. At BCG X we have developed a responsible AI philosophy , which our team is ready to help you with to set up the appropriate organizational structures, processes, tools and guardrails, starting from the leadership suite all the way down to the product solution.
Generative AI is a rapidly evolving technology that holds immense potential for businesses across industries. As a business leader, it is not a question of whether to adopt genAI but rather how to apply it effectively. By prioritizing user centricity, rethinking solutions development principles, and setting appropriate guardrails, businesses can harness the power of generative AI to create lasting value for both their organizations and users.
At BCG X, we have a proven track record of building and scaling more than 1000+ AI solutions and 200+ successful ventures. Our focus on user-centric design, strategic thinking, and responsible implementation ensures that our clients rapidly unlock the true potential of genAI. Together we can shape a future where AI solutions deliver tangible results and value to users and businesses.