Generative artificial intelligence (GenAI) has the potential to make governments much more efficient and effective. The impact of GenAI on the public sector will be significant. For instance, in our first article in this series, we revealed that the potential productivity improvements from GenAI could be worth $1.75 trillion per year by 2033 globally across all levels of government. GenAI tools will augment the work of public servants, reducing the time required to complete certain tasks and enabling them to focus more time on higher-value work. Similar to other industries, this will fundamentally transform how the public sector operates and the nature of government employees’ tasks and activities.
Getting started with GenAI does not require major upfront technology investments. In most cases, an iterative approach—focusing on experimentation and ongoing capability development, matched to the organization’s AI maturity—works best. By strategically implementing GenAI in targeted areas, public sector leaders can drive impactful improvements in service delivery, operational efficiency, and citizen engagement while minimizing risk and exposure and building the skills and capabilities to scale up their transformations.
This article is the second in a three-part guide for governments about unlocking the potential of GenAI. The first article, “ From Opportunities to Value ,” introduces the productivity gains and changes in services available through GenAI technology. The third article, “Practices and Policies for Risks and Responsibility,” will provide a roadmap for avoiding unintended harmful consequences. Here we look at key enablers that can help public sector departments and agencies unlock the benefits of Gen AI throughout their organizations.
How Government Entities Can Unlock the Potential of GenAI
Pilot projects provide an invaluable learning opportunity in the early stages of the GenAI implementation journey. These typically involve a small team developing a proof of concept and minimum viable product. As teams gain experience and confidence, these efforts can be scaled up to enterprise-level robustness, reliability, sustainability, and performance. (See Exhibit 1.)
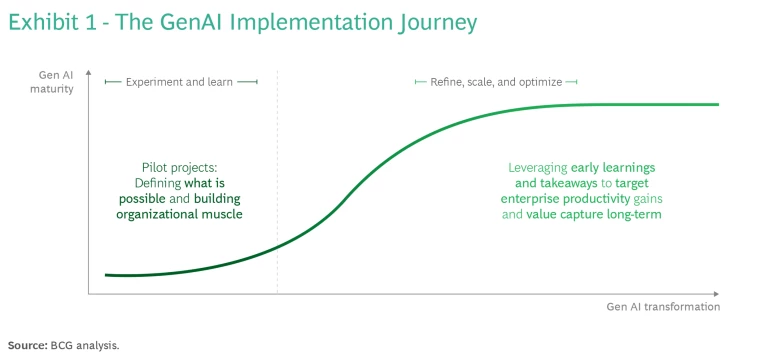
Unlocking the potential of GenAI requires six key organizational enablers:
- Leadership
- People and skills
- Partnerships
- Technology
- Data
- Policies and governance
We examine the first five of these elements in this article. Our next article in this series will deep-dive on the sixth and final enabler.
Leadership
Experiment and learn. Senior leaders need to instigate and drive GenAI adoption across the public sector. Organizations should focus on practical training for staff and hands-on experience, using the tools on the job to quickly bring people up to speed on how GenAI can be used and how to manage the risks. Government leaders will be called on to navigate new, complex ethical and technical challenges. They will be required to make decisions about the technology’s implementation and use, how it will be monitored, and how potential risks will be managed. To do this, they will need a solid understanding of GenAI tools, their transformational potential, and their risks and limitations.
Already, many employees may be concerned about the potential impact of GenAI on their jobs and career prospects. Recent BCG research has found that the sentiment of leaders towards GenAI versus frontline employees differs sharply. Leaders are likely to be more optimistic than employees (62% vs. 42%) and less likely to be concerned (22% vs. 39%) about the implications of GenAI. Thus, government leaders must be prepared to address staff concerns, openly discuss how GenAI might affect the future composition and size of the workforce, and invest in upskilling and reskilling of staff whose roles and activities may change to varying degrees. Messages should focus on safe and ethical use and hands-on experimentation, with incentives and support that reinforce this in the organizational culture. Establishing guardrails for the safe and responsible use of AI also helps staff understand expectations and boundaries and provides some reassurance that they are operating within an approved policy framework.
One way to address staff concerns is to help them develop greater awareness and familiarity with the tools. As individual employees gain experience, their optimism grows. Regular users of GenAI are less likely to be concerned about it (22% vs. 42%), partly because they see its potential value and the limitations. (See Exhibit 2.) Public sector leaders should actively support staff discussions about GenAI, encourage enthusiasts to speak up and offer demonstrations, and involve themselves directly.
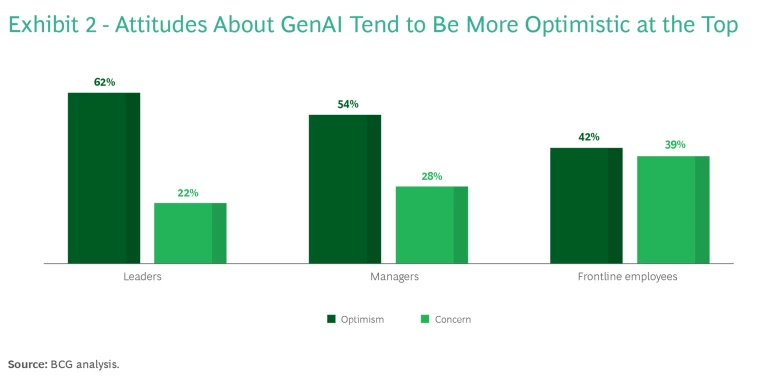
Early GenAI projects should be designed to help staff members see the art of the possible firsthand. For example, staff at the Yokosuka City Government in Japan are trialing GenAI to take meeting notes, summarize existing materials, write text, and check for errors. These relatively low-risk use cases enable staff to better understand how GenAI can be used in their day-to-day work, allowing them to become comfortable with the tools. In Singapore, the government is giving staff from public sector departments the opportunity to be directly involved in the development of new GenAI tools through its AI Trailblazers program. Each team will receive targeted training prior to their involvement and will work with government leaders to deliver new GenAI applications.
Refine, scale, and optimize. Leaders will also play a critical role in bringing GenAI use to every part of the organization. For example, they can boost adoption and drive culture change by strategically using behavioral nudges. Specific nudges might include:
- Making GenAI tools easily available and accessible
- Highlighting and celebrating GenAI applications in internal communications
- Conducting internal forums where staff can showcase their GenAI demos
- Disseminating information about examples, best practices, and lessons learned
- Gamifiying training with badges and certifications
These efforts foster an appetite for innovation, accelerate the adoption of GenAI solutions, and ultimately create a culture where responsible use of GenAI is seen as attractive, intuitive, and safe.
People and Skills
Experiment and learn. In the short term, cross-functional squads are the best way to drive pilot use cases. These squads should include business owners and users, machine learning and AI engineers to design and optimize GenAI solutions, data scientists focused on transforming and managing data, IT experts who can guide integration, and GenAI policy and governance advisors to ensure solutions are designed and implemented responsibly.
Organizations should start by picking lower-risk projects they can readily master, such as document preparation or synthesis. Gradually, they can gain more proficiency and recognize the capabilities they don’t yet have to address more complex tasks, such as offering new services to the public. This continual reassessment can help a department decide when and where to invest in larger-scale data platforms and other GenAI tools and processes.
Refine, scale, and optimize. GenAI will significantly impact public sector roles, and new skills will be needed. Repetitive tasks, knowledge synthesis, and the process of content creation will all be improved and even transformed. Many roles will be redesigned to reflect changes in the way people work. (See Exhibit 3.) For example, the US Department of Defense is currently developing a GenAI tool to automate the preparation of procurement documentation. The tool can generate complex procurement documents in 15 minutes that previously took days to create. This will allow procurement staff to focus on higher value-added tasks such as developing vendor relationships, engaging with bidders, and optimizing procurement spend.
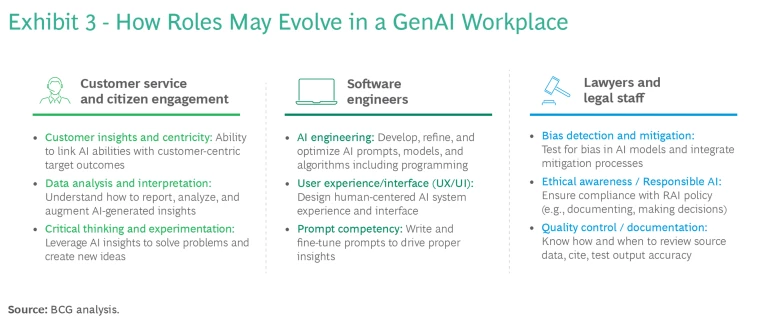
However, being able to undertake these higher-value activities is likely to require additional investments in training, reskilling, and upskilling. Employees and employers are aware that a skills gap exists for GenAI. For example, the United States Office of Personnel Management (OPM) has recognized this challenge resulting from GenAI and AI in general. OPM has released a memorandum for Chief Human Capital Officers that provides guidance to agencies on targeting AI skills—such as data extraction and transformation, testing and validation, and systems design—which are needed to expand government-wide AI and GenAI capabilities.
Additional resources are needed to upskill and train staff on AI tooling. BCG research has revealed that 86% of frontline employees believe they will need training to adapt, but only 14% have received formal training to address how these tools will change their jobs. Some governments are already investing to close the gap. For example, the Dubai government has announced it will provide targeted training in GenAI technology to public sector workers. The training will equip staff with foundational knowledge about GenAI, including the opportunities and risks it presents, to familiarize them with various GenAI tools and to provide them with practical use cases highlighting how the tools can enhance efficiency, effectiveness, and service quality.
GenAI will reshape the future of government work by transforming workflows and reinventing processes. Public sector leaders must act strategically to reorganize their workforces to support this transition. By aligning upskilling and recruitment initiatives with projected skill demands, governments can empower their workforce to excel in augmented and transformed roles.
Partnerships
Experiment and learn. Partnerships with other entities—tech companies, industry, universities, and experts—will be a key enabler. Governments often struggle to attract and retain the required technology skills. A strong employee value proposition (EVP) will be crucial to fill capability gaps, supported by incentives such as compensation, job flexibility, customized and accelerated expert career tracks, and continuous learning programs. In the early stages of implementation, partnerships will also enable the government to rapidly access knowledge and skills that are missing internally. External organizations bring valuable perspectives on policy, regulation, and high-value use cases. For instance, the Canadian government has partnered with and provided funding to the Université de Montréal to develop and implement new AI design and adoption strategies in three priority sectors: drug discovery, environmental emergencies, and health systems logistics.
Refine, scale, and optimize. Partnerships also play a role in roadmap development, upskilling, coordinating panels and other flexible working groups, and streamlining access to leading-edge technology. They are catalysts and accelerators that allow government organizations to scale much faster than they otherwise could on their own. For example, the Australian government has partnered with Microsoft to undertake a six-month trial of Microsoft 365 Copilot. Microsoft is providing training, onboarding, and implementation assistance to participating agencies, with 7,400 public servants participating in the trial from January to June 2024. Exhibit 4 shows different types of partnerships that governments can form and leverage as they progress with deploying GenAI.
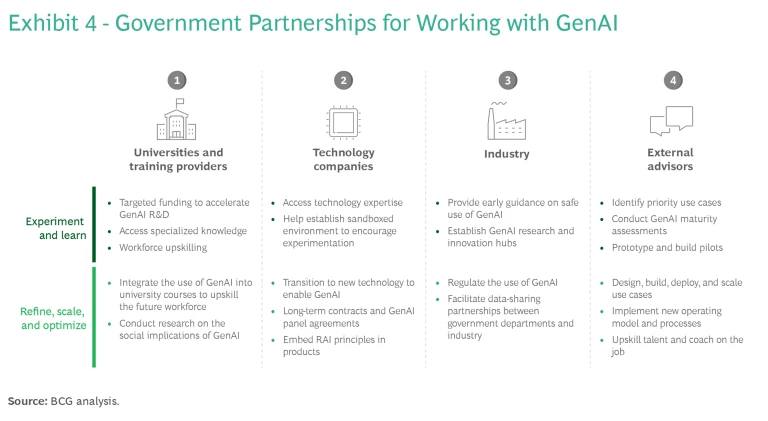
Technology
Experiment and learn. Each government entity has unique needs and use cases, along with its own level of technological readiness as well as constraints around the use of algorithms and data. The high-level components of the GenAI technology stack are outlined in Exhibit 5. They include a user interface (e.g., ChatGPT); GenAI algorithms and foundational models (e.g., OpenAI GPT, Google Gemini, Anthropic Claude); diverse, extensive, and accessible data sets; and computing infrastructure.
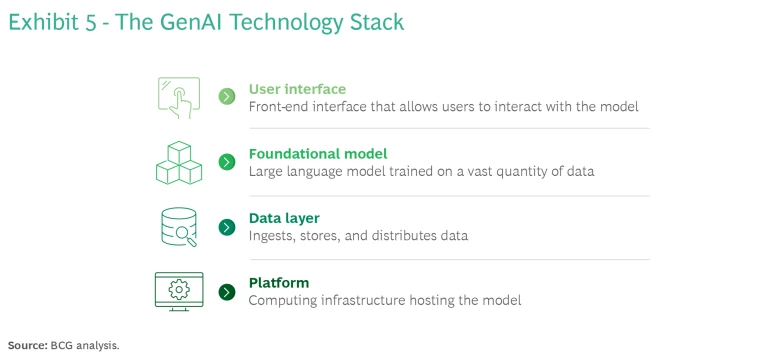
Much can be accomplished already with off-the-shelf GenAI solutions. These GenAI tools can provide faster, easier, and more cost-effective implementation of early use cases. Basic experiments can help governments assess the general feasibility of GenAI solutions, while staff gain GenAI familiarity with minimal commitment and risk.
Refine, scale, and optimize. There are a wide range of platforms, foundation models, and implementation paths to consider for implementing generative AI . The choice of foundation model should be driven by the requirements of the use case being addressed. These requirements might include the types of output it can produce (text, visuals, video, code, or other formats), the level of fine-tuning it will need, and its compliance with data sensitivity and responsible AI guidelines.
Additionally, the department’s level of control over the model should be considered. For example, if a significant degree of control is needed, an open-source model that can be freely altered and changed may be selected over a proprietary model. Platform purchasing decisions should be based on an assessment of whether vendors can securely provide services to your geography in line with your responsible AI and security needs.
Compatibility between existing platforms and models should also be a consideration, as this can reduce implementation barriers. For example, the South Australian Department of Education partnered with Microsoft to develop an AI chatbot, EdChat, as part of an 8-week trial with 180 educators and 1,500 students across 8 schools. Leveraging their existing Microsoft Azure cloud platform, they were able to implement an OpenAI GPT4.0-based solution. Exhibit 6 shows criteria for choosing models and platforms, tailored for the public sector.
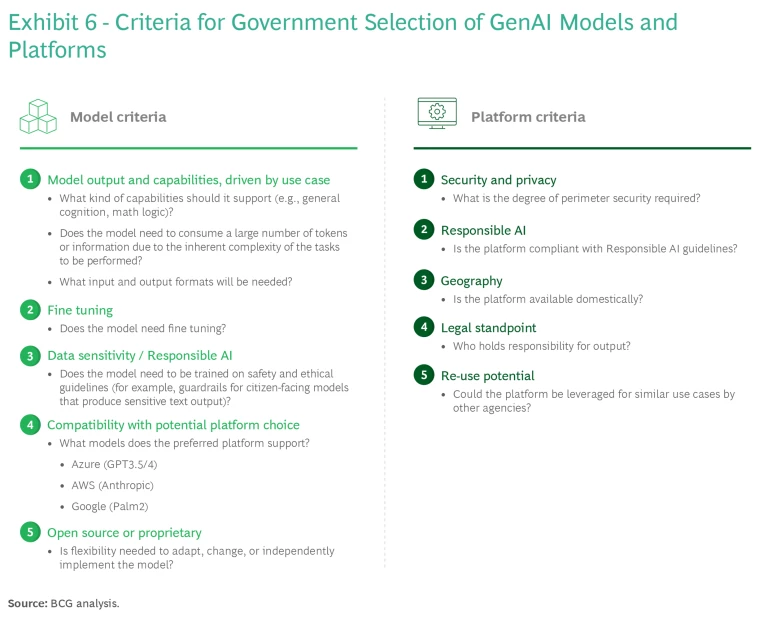
Some jurisdictions are exploring the need to invest in building their own large language models (LLMs). In some countries where English is not the primary language, such as France and Japan, there has been significant focus on developing their own LLMs. Sovereign capability may also be a requirement for some applications in the defense and national security domains. However, the cost of building a new foundational model is very high, and the business case will not be justified in most instances. An alternative to building a completely new model may be to engage a vendor who can fine-tune an on-premises model and leverage the benefits of retrieval augmented generation (RAG).
Cloud-based GenAI deployments are typically more flexible, more cost-effective, and easier to keep up to date with the latest developments in this rapidly-changing domain. Where there is a critical need to sandbox data entirely, and where abstraction layers and data anonymization strategies do not provide sufficient protection at scale, organizations are more likely to pursue an on-premise solution. Many organizations will combine options for different purposes, as shown in Exhibit 7.
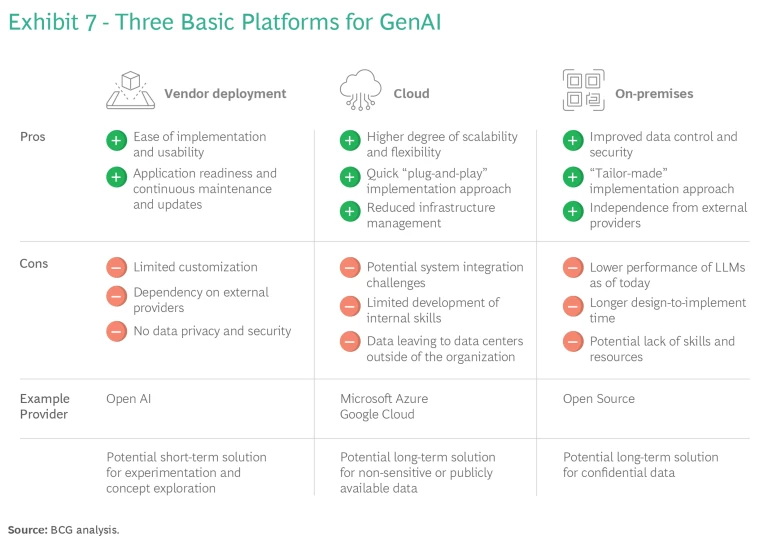
Data
Experiment and learn. Like all AI applications, GenAI’s value is inextricably linked to the volume and quality of the underlying model training data. GenAI models can leverage more unstructured, varied, and multimodal data than traditional (deep learning, machine learning) AI, but the quality of the outputs still depends on the quality of the inputs. The input for most GenAI models includes a combination of text, graphics, audio, video, and other data obtained from the open internet, combined with other data sources that the producers of the models have sourced or acquired. While substantial efforts are made to correct for and reduce unwanted bias, users must understand the potential inherent risks in any AI model based on the underlying data.
Refine, scale, and optimize. The roadmap to scaling GenAI capability may require some investments in upgrading digital and data platforms and modernization of legacy technology environments. A well-organized, common data platform will allow governments to gradually make their data more generally accessible, facilitating the transition from a small number of use cases and pilots to the organization-wide integration of GenAI. This will typically involve enabling access to data that is siloed across the organization, improvements to data governance, adding AI capabilities to the data management platform itself, integrating new advanced software and hardware layers dedicated to AI systems, and providing access to new and varied sources of data.
To fully unlock the power of this data, governments will also require modular platforms that share the data across the organization and liberate it from legacy systems. Some government agency leaders have expressed concerns about their legacy systems and data quality, which may not effectively support GenAI use cases or applications. By deploying an incremental strategy to modernize the technology architecture and using GenAI itself, governments can realize quick wins from this technology and see its benefits early on.
At the same time, governments must manage this new environment carefully. GenAI’s use of unstructured data requires new approaches to governance and data lifecycle management. For example, multi-modal LLMs may use inputs from documents, audio calls, or video contacts. This heightens the risk of collected data being used for something outside of its approved or intended purpose.
The data governance function of an organization should be closely involved. It is important to be able to clearly track and record data provenance, documenting how data has been obtained, from whom, and what permissions or usage constraints apply to it. The function should also manage data classification, making sure the data is labeled appropriately. Data governance is especially important in the public sector to prevent overreach and ensure data use stays within legal, ethical, and privacy boundaries.
Conclusion: A Balance Between Caution and Vision
Given the consequential nature of their decisions, many public sector organizations adopt new technologies cautiously. However, governments must also look at the potential for new technologies to enable them to achieve their intended impact more efficiently and effectively and generate greater public value. The five enablers in this article—leaders, people and skills, partnerships, technology, and data—should be oriented to balance the potential benefits with the risks involved.
Each government’s journey to becoming GenAI-enabled will be different and should be designed based on the unique needs arising from the role, structure, and the services it delivers. Each organization’s starting point and focus areas will be driven by its own AI maturity and specific use case goals. Fortunately, GenAI can be adopted and developed in an iterative approach—adding new GenAI tools, learning how they work, and integrating them into the current technological and organizational platform before moving on to the next iteration—but there is a strong imperative to get started on the learning curve.
As we’ve seen, any implementation of GenAI in a public sector context requires a strong focus on governance, risk management, and security. The third article in this series, “Practices and Policies for Risk and Responsibility,” will discuss these points further.