Intelligence is often seen as humanity’s superpower, the trait that sets us apart from other life forms. This belief also pervades the world of business. Companies fiercely compete for intelligence: from recruiting the brightest minds to amassing vast amounts of information and developing sophisticated algorithms to process it. Embracing artificial intelligence is seen as the natural next step.
However, the true superpower of humans lies in our sociality and aptitude for social learning. Human knowledge is not only individual but also embedded within a broader social context. Our ability to learn from and collaborate with others is what sets us apart as a species. It is also what sets great companies apart from the rest of the pack.
Social Learning Drives Competitive Advantage
The pace of both knowledge generation and obsolescence is accelerating, necessitating a more rapid and effective learning and adaptation process. Competition no longer revolves solely around scale or operational efficiency but also around the rate of learning . Unlike the slower, more costly method of learning through individual trial and error, social learning enables us to acquire knowledge through the experiences of others—by observing what has worked under which circumstances.
Companies are social organisms and are also embedded in a broader social context. Social learning, therefore, happens naturally as individual learnings are codified and disseminated both within and beyond the enterprise. Employees may learn from colleagues on their teams, from the codified knowledge of many such teams, or from collaborators and customers in business ecosystems.
However, few companies have a deliberate and explicit focus on social learning and understand what it takes to get it right. In an era in which AI has the potential to enhance social learning, this focus becomes even more critical. An enhancement of social learning through AI will not happen automatically, however—it requires a deliberate, strategic approach.
How Social Learning Works in Organizations
The concept of knowledge generation and processing in organizations was shaped by the work of organizational theorist Ikujiro Nonaka, who introduced the SECI (socialization, externalization, combination, and internalization) model in
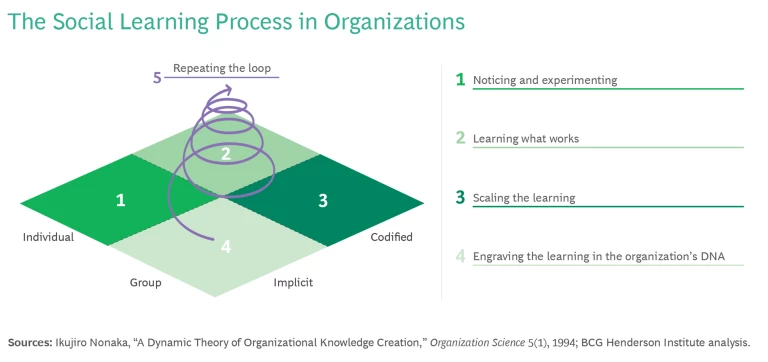
Social learning in an organization requires creating new knowledge, testing and refining it, spreading it across the organization, and institutionalizing it. This process ensures that new learnings are not just fleeting individual thoughts and actions but become lasting, integral, and accessible parts of the organization’s knowledge base and operational framework.
- Noticing and Experimenting. The process starts with an individual in the organization noticing something intriguing or unusual in the business or its
environment.2 2 Martin Reeves, Mihnea Moldoveanu, and Adam Job, “ Radical Optionality,” Harvard Business Review, 2023. This is often in the form of an anomaly—an unexpected occurrence that challenges an existing mentalmodel.3 3 Martin Reeves, Bob Goodson, and Kevin Whitaker, “ The Power of Anomaly,” Harvard Business Review, 2021. This may lead to further, more detailed observation, or spark an idea that leads to an experiment. For these to occur, employees need to be regularly exposed to surprises and new information—ensuring that they do not become prisoners of the assumptions underpinning their current approach, as BCG’s founder Bruce Henderson cautioned back in 1968. - Learning What Works. The individual analyzes and documents the insights arising from their observations or experiments, crystallizing the acquired knowledge. In so doing, individuals implicitly apply selection procedures regarding what knowledge is worth retaining and sharing. To find out what works, individuals need to create collisions of different ideas with reality and be open to learning—while their broader organization has to be accepting of the inefficiencies inherent to experimentation.
- Scaling the Learning. Crystallized learnings are socialized to the wider organization through direct communication—for example, a group meeting or training session for employees—or through integration into a knowledge inventory, such as a knowledge management system. This also entails applying selection at the group level regarding what works and is useful at scale and building scripts to ensure that valuable learnings are properly documented and accessible. To allow knowledge to jump from brain to brain, firms need to ensure that communication barriers—such as organizational or functional boundaries—are broken down. Equally important are mental and cultural barriers such as an overemphasis on short-run efficiency or a lack of curiosity for new ways of doing things.
- Engraving the Learning in the Organization’s DNA. Over time, the new information shared within the organization becomes institutionalized. New ideas are followed implicitly by becoming invisible as parts of other processes—no longer seen as separate or new practices, but instead as part of the shared knowledge that integrates seamlessly into the organization’s culture and operations. Over time, implicit knowledge transfer happens automatically; new employees will effortlessly adopt it by observing their colleagues.
- Repeating the Loop. After an organization has adopted a new way of doing things, new information will continue to emerge—from reactions of the customers and competitors to its application, as well as from the observation of new anomalies in new circumstances. This restarts the loop of observation, ideation, and experimentation, and also creates an opportunity to codify and refine of processes and capabilities of learning itself.
One of the most consequential examples of social learning is the discovery of penicillin and the development of antibiotics. (See “Social Learning in the Discovery and Legacy of Penicillin.”)
Social Learning in the Discovery and Legacy of Penicillin
- Noticing and Experimenting. The discovery of penicillin provides a well-known example of this step. Dr. Alexander Fleming, a bacteriologist, observed an anomaly in 1928 when he noticed that a certain mold called Penicillium notatum had contaminated one of his Staphylococcus culture plates and that the bacteria surrounding the mold had been destroyed. Fleming formed the hypothesis that the mold was producing a substance that could kill bacteria.
- Learning What Works. Fleming documented his observation that the Penicillium-based secretion could kill bacteria in a scientific paper published in 1929. While noting the antibacterial properties of the mold, he also documented the limitations he encountered, such as difficulties in extracting the substance and its instability.
- Scaling the Learning. The broader medical community did not in fact immediately recognize its potential. Researchers at the University of Oxford came across Fleming’s paper nearly a decade after its publication and developed a method to purify the antibiotic substance, conducted clinical trials to demonstrate its efficiency, established a process to mass produce it, and made efforts to show its value to pharmaceutical companies.
- Engraving the Learning in the Organization’s DNA. Penicillin was integrated quickly into medical practice and was widely used to treat infections during the Second World War. 4 The success of penicillin helped establish the framework for the modern pharmaceutical industry: Developing processes for creating new antibiotics, testing them, and incorporating them into medical treatment became a standard part of pharmaceutical organizations’ operations and culture. The knowledge and techniques associated with penicillin and antibiotics research are now routinely passed down through medical education, training, and clinical practice.
- Repeating the Loop. For penicillin, new social learning cycles were initiated, for example, as researchers observed that other substances also had antibacterial properties that were more suitable for different kinds of infections. This has led to the development of more than 100 types of antibiotics, including broad-spectrum variants. As antibiotic resistance in the population increases, new treatment protocols are being developed to combat it, continuing the cycle of innovation.
AI Can Supercharge Corporate Social Learning
For most companies there is huge potential to improve social learning, even before considering the application of new technologies such as AI, by applying the basic disciplines of designing, measuring, and optimizing learning processes and supporting elements like incentives and culture. Indeed, without putting these human learning architectures in place, technology is unlikely to be impactful. But with this firm foundation in place, digital technologies certainly have the potential to supercharge each step of the social learning process detailed above.
Extend the search domain and enhance perception. Digital technologies can expand the reach of the organization by providing access to larger knowledge pools through digital ecosystems or shared data pools. This can also create a competitive advantage by creating critical information not available to others. Complementing this extended knowledge base, AI can enhance the quantity and quality of information extracted from it or reduce the costs of search and analysis. AI can, for example, discern patterns within large datasets, highlighting anomalies or connections that would likely remain hidden to human intelligence.
Moreover, AI can boil down complex information. Large language models (LLMs), for instance, can extract the “average” view on a particular topic by synthesizing the most prevalent perspectives from a vast database of human knowledge. Furthermore, these models can translate this information into outputs that are easily understandable, for example, by transforming it into pictures, infographics, or tables.
Finally, AI can tailor information to the needs of the requester. This may take the form of providing information in a chosen language, style, or providing recommendations tailored to a particular situation. AI can also personalize knowledge extraction, making it more relevant and accessible to the individual user. In essence, AI is not just an extension of our knowledge base; it can potentially transform how we interact with and perceive the world of information.
Supercharge experimentation and the distillation of learnings. Digital technologies can supercharge experimentation, allowing companies to run experiments at increased scale and greater speed—for example, by orchestrating many experiments simultaneously, running simulations, and automating data collection—helping firms rapidly identify what works and what does not.
AI can also be used as a “thought partner” to refine new strategies before they hit the public eye. For example, Google uses AI-powered tools for creative testing, predicting success or failure of marketing pilots.
Finally, AI can support individuals with clarifying and distilling their learnings, enabling the codification of successful strategies in formats easily understandable and accessible to others, such as tables, infographics, presentations, or reports.
Effectively disseminate learnings and determine what works at scale. Some may believe they have the dissemination and management of knowledge across the organization figured out in the form of digital knowledge management systems, which collect and organize information and make it accessible to the whole organization. However, our experience is that the knowledge management systems which many firms have built over the last decade, have generally not yet lived up to expectations. This can be because knowledge is often not properly captured or updated or is often difficult to find or navigate. Most companies are still far from understanding how to capture and grow their collective intelligence. New digital technologies may help make such systems more effective by providing more flexibility in how knowledge is stored and extracted.
Generative AI can improve knowledge archiving, automating the processes of capturing and maintaining information within a knowledge system; it can help with the identification of knowledge gaps and the generation of new content through development of internal knowledge articles, FAQs and graphical representations of interconnections between topics, people and projects. For example, ServiceNow is rolling out an LLM that allows its customers to generate internal help desk articles. AI chatbots can also support effective knowledge dissemination by making knowledge more easily searchable, by extracting the most relevant information, or by tailoring the responses to the individual requester.
Beyond mere knowledge storing and dissemination, AI can also translate learnings directly into action without human intervention. This involves algorithms that are connected with decision engines and can act without human involvement—allowing firms to scale and apply insights quickly and efficiently. The decision engines used by Netflix or Spotify to create new user recommendations are a prime example.
Make mental models explicit and evolvable. Digital technologies can aid collective reflection and help firms better understand their implicit knowledge bases. LLMs can observe and articulate our mental models and assumptions by assessing information from meetings, documents, or internal communications from across the organization, and representing it back to employees. By making these beliefs about business models, the competitive environment, and culture explicit, AI can help make them more malleable—which is crucial for enabling a corporation to reimagine itself.
Draw attention to critical new anomalies. AI can actively monitor changing parameters generated from new behaviors and environmental reactions (customer feedback, market trends, competitor actions), which helps pinpoint new interesting anomalies—thus restarting the social learning loop.
Build and enhance social learning systems. Technologies like AI can supercharge social learning, as described above, but they will not do so automatically. Rather than jumping headfirst into integrating AI applications into each step for their operating processes, companies should think about social learning as a system comprising both humans and machines, focusing on recognizing, improving, and enhancing it.
Map your current learning system. Understand the current learning mind-set and processes in your organization. Questions such as “What are our sources of surprise?” and “How are learnings codified, selected, and disseminated?” are vital in understanding how learning takes place or fails to take place in an organization. It is the foundation for learning how to learn.
Establishing self-awareness of the learning process and immediately can help identify potential improvements. For example, the success of language learning app Duolingo is based on how it adjusts the difficulty, pace, and format to a user’s personal learning style.
Improve traditional learning. In each step of the learning process, ensure that the right elements are in place. Reevaluate and revise existing policies, fostering an environment that not only permits but actively encourages experimentation and sharing of new insights throughout the organization.
Incentivize curiosity and creative thinking, leave room for employees to test out new possibilities, train them on how to properly codify their acquired knowledge, ensure communication pathways exist and are well-functioning, and make processes more malleable to new insights. For example, Investment Day (InDay) at LinkedIn aims to reserve employee time to promote connection, sharing of knowledge, and purposeful projects.
Introduce technology to extend and enhance the learning system. With the introduction of technology, new types of learning become available, such as human-human, human-machine, and machine-machine. These can lead to opportunities for enhancement and reimagination of the learning system.
Establish where each of these types of learning might be best suited depending on the context, defining strategies for human-machine cooperation . For example, a human-human system might be more effective for a collective creativity task, a human-machine system might best suit individual experimentation contexts, and a machine–machine system might best suit contexts where significant and fast scale-up is desirable and possible. The strategic choice and combination of capabilities depending on the situation can enhance the overall learning process.
Establish a strong governance. A social learning system requires regulation and continuous adaptation to ensure its fitness for purpose in a constantly evolving landscape. The need to refresh algorithms or address new domains will inevitably arise, introducing questions about when and how to incorporate these changes. Pitfalls can also arise in cases of over-reliance on algorithms whose historical data and parameters cease to work in volatile environments. For example, LTCM’s over-reliance on models that were mismatched with new circumstances led to the hedge fund’s collapse.
Technology deployment focused on individual operational steps can easily overlook the effectiveness of the overall learning system. Consider an analogy of a spark plug: In isolation, it might not seem that revolutionary. However, when it is part of an internal combustion engine within a car, and that car is driven by a person within a society that has roads, traffic laws, and a culture of automobile use, the spark plug becomes part of a revolutionary technology. It is the system bringing individual components—the car, the driver, the roads, the laws—together in a meaningfully interconnected way that gives each of the individual parts their value.
Be mindful of traps. A singular focus on only one part of the learning system (either AI or human) can lead to a myopic view of the process. The key to unlocking the full potential of social learning lies in an integrated approach that bridges both aspects.
Similarly, enhancing only select steps of the learning process with AI, rather than the entire learning system, means foregoing synergistic potential between the steps.
Finally, traditional metrics that measure the input-output efficiency of processes are no longer sufficient. To compete on the rate of learning, new metrics must be introduced that indicate how quickly and effectively the organization can learn and evolve.
We believe that a well-functioning system of social learning, especially when supercharged by new technologies, can provide a significant competitive advantage in the current dynamic business environment. But new technologies such as AI will only enhance learning competitiveness if embedded in well-designed, holistically conceived learning systems.