As the generative AI map takes shape, the US and China are asserting their dominance. Tech companies from these GenAI superpowers have built a substantial lead in the creation and large-scale commercialization of top-performing large language models (LLMs). In a world where GenAI is quickly becoming a critical resource, the US and China are currently on pace to control the supply.
But something interesting is happening in parallel. A small group of countries—the “GenAI middle powers”—is emerging, each with its own distinct strengths that may enable it to compete on a regional and even global scale as a supplier of the technology. The implications for companies are significant.
For corporate leaders who are integrating GenAI into an increasing share of their products and services, and who are operating across multiple geographies, relying solely on GenAI supplied by companies in the US or China could pose serious challenges, with local regulations, data requirements, and the availability of LLMs all subject to shifts in government policy. Although a more multipolar supply of GenAI increases complexity, it would also create critical optionality.
CEOs need to understand this dynamic—and be able to navigate the evolving geopolitics of GenAI. The traditional approach—determining which country can acquire the most advanced semiconductors, for example, or which has the most favorable regulatory environment—won’t suffice. Company leaders can assess the relative strength of GenAI superpowers and middle powers across the six key enablers of GenAI supply: capital power, talent, intellectual property (IP),
data
,
energy
, and computing
This is not about a country’s rate of GenAI adoption, which can often be decoupled from its ability to supply the technology. The former was assessed in a recent BCG study of 73 global economies’ exposure to and readiness to deploy GenAI . (See the sidebar “The Adoption Advantage.”)
The Adoption Advantage
BCG’s recent AI Maturity Index offers a comprehensive assessment of countries’ exposure to AI-driven economic disruption across industries as well as their readiness to seize the economic advantage the technology makes available.
As generative AI matures, foundation LLMs will increasingly be put to use through industry- or function-specific applications. Robust ecosystems of companies working on this application layer of the technology will be an important driver of success for countries looking to make their industries more competitive through AI.
India is a powerful example of a country that is well-positioned to foster such an ecosystem. The government’s IndiaAI Mission is committed to supporting extensive adoption through the creation of centers of AI excellence, which aim to integrate the technology into key sectors like agriculture and education. India also benefits from its historical strength in tech talent, as it is home to a large pool of 215,000 AI specialists.
While Indian companies have already released some LLMs, the country is likely to increasingly focus on fostering an ecosystem of companies heavily invested in industry-specific GenAI applications, such as Setu’s Sesame for finance and insurance, Fractal’s Vaidya.ai for health care, and Lexlegis’ Lexlegis.ai for legal services.
Focusing our research on supply enables us to help CEOs determine how to ensure their company has reliable and flexible access to this game-changing technology. Here is a closer look at the major players and the other contenders.
Subscribe to our Artificial Intelligence E-Alert.
The GenAI Superpowers
The US and China are currently the only players with robust access to and control over sizeable portions of the whole GenAI value chain. They produce the most IP and they have the largest AI talent pools; they have some of the richest data ecosystems in the world and the most data center infrastructure capacity, and they lead in capital access.
Today’s Default Is “Made in the USA”
The US has had a pronounced head start on GenAI, building on decades of
AI
leadership. Nearly 70% of the world’s notable AI
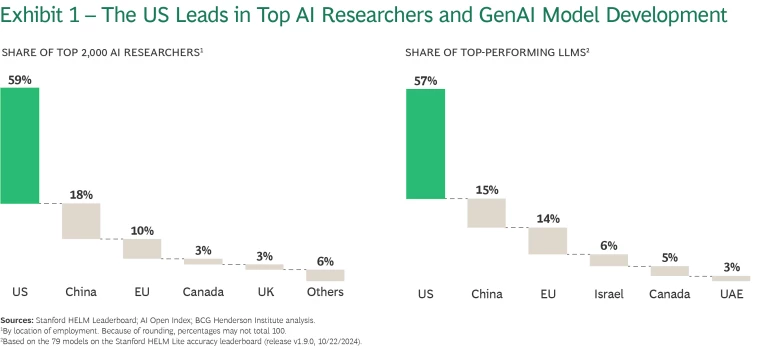
The US is home to some 60% of the top 2,000 AI scholars in the world and attracted roughly one-quarter of all AI specialists that relocated globally between 2022 and 2024; its total AI talent pool has grown to nearly half a million people, the largest in the world. And US-based AI scholars have contributed 35% of the world’s most influential papers in the field of AI since its
US-based GenAI startups have also received unparalleled private investment: a total of $65 billion since 2019. In addition, AI-directed capital expenditures within established tech firms like Alphabet, Amazon, Meta, and Microsoft are expected to exceed $200 billion for 2024. With massive computing capacity, a full-stack approach extending to hardware codesign, deep pockets, global reach, access to the world’s top talent, and in-house model developers or deep partnership with outside developers, these companies are singularly positioned to support US leadership in GenAI in the foreseeable future.
Beyond talent and capital, the US also has the infrastructure to support inference at scale—that is, to supply GenAI on an ongoing basis to model users. It is the largest data center hub in the world, with an estimated capacity of approximately 45 gigawatts in 2024. The US has reliable access to cutting-edge hardware (through US-based Nvidia and strong geopolitical ties to Taiwan-based TSMC) to support data center expansion, though long lead times to connect to the electrical grid may create challenges.
Made-in-China Models Are Catching Up
Some signs indicate that China is catching up to the US on some key enablers and making rapid progress in the production of top LLMs. Two Chinese companies, Alibaba and the GenAI startup 01.AI, contribute over one-quarter of the world’s top open-source models. Established tech giants Baidu and Tencent have also released high-performing models, as have a new generation of GenAI startups, the so-called AI tigers: Zhipu AI, Baichuan AI, Moonshot AI, and MiniMax AI. Top Chinese models have substantially reduced the gap compared to state-of-the-art alternatives in the last year. (See Exhibit 2.) And top Chinese models have effectively closed the gap in Chinese language benchmarks.
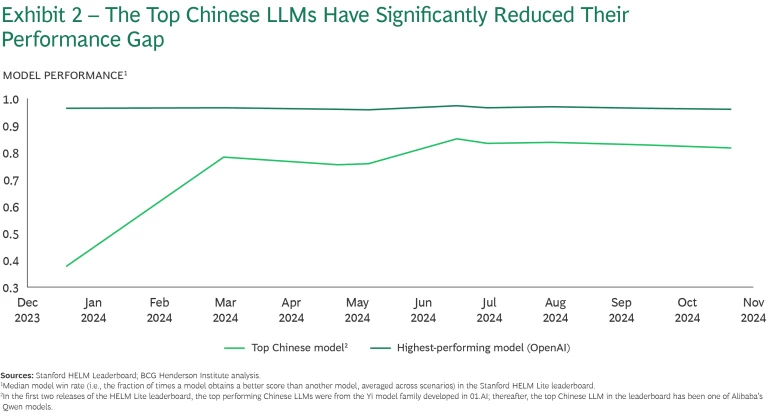
The Chinese GenAI ecosystem has benefitted from access to foreign open-source or open-weights foundation models: 01.AI’s Yi model family, for example, is based on Meta’s Llama. (See the sidebar “ A Landscape of Open-Source and Closed Models .”) The Chinese government has signaled its intent to ensure an ample supply of domestic open-source options that play a similar role in the future, supporting a growing number of smaller companies as they ramp up their technical capabilities.
Although it trails the US, China still has a strong AI talent bench—and potent national research champions like Tsinghua University and Shanghai Jiao Tong University. The four AI tigers are all founded by Tsinghua faculty or alumni. China’s strength in AI talent is apparent in its leadership on patents, with more than 76,000 AI patents filed with the World Intellectual Property Organization between 2019 and 2023, about four times as many as the US.
China also has ample data center infrastructure, with approximately 20 gigawatts of capacity, and significant capital through its public R&D budget, which has averaged $50 billion per year between 2016 and 2023. In fact, government venture capital funds demonstrate China’s commitment to boosting AI innovation through public investment. Government VCs have invested nearly a quarter of their total funds in AI firms, exceeding $180 billion in total since 2000, of which roughly $100 billion was invested between 2019 and
Methodology
The quantitative assessment of relative strength across enablers draws in part on the history of countries’ strengths in and contribution to AI as a broad family of technologies, of which GenAI is only a subset. This in part reflects limitations in reliable transnational data, given the recency of GenAI’s rise in importance. But, more importantly, indicators associated with the long arc of the history of AI speak to the endurance of critical capabilities, particularly on talent and innovation ecosystems.
Since our primary objective was to develop a relative sense of strength across enablers, we developed normalized scores by enabler country or region pairs in the following way:
- Each indicator leads to an absolute value by country or region. These values are not normalized by population, size of the economy, or other such factors because competition in the supply of GenAI is largely a function of scale.
- Values for each indicator are then (linearly) normalized into country or region scores on a scale from 0 to 1, where 1 equals the highest actual country value in our data set and 0 is set as absolute 0 (except for the energy enabler, which reflects industrial electricity prices: in this case, 0 equals the lowest actual price.
- Then, for each country or region, we take the average normalized score across indicators to generate the enabler score. A country would only have a score of 1 in an enabler if it had the highest absolute value of all countries in our data set for all indicators associated with the enabler in question.
Talent:
- Share of the top 2,000 AI researchers worldwide, based on the location of the authors of leading AI publications. (AMiner)
- Share of the top 300 AI institutions, also based on the location of authors of leading AI publications. (AMiner)
- Size of the AI-specialized talent pool working within a country (though not necessarily employed by a local company). (BCG Talent Tracker)
- Share of notable machine learning models developed since 1959 by, or in partnership with, researchers or institutions from a particular country. (Epoch AI)
- Total number of AI publications produced by authors and/or institutions from a particular country between 2019 and 2023. (SCImago)
- Total number of citations of AI publications produced by authors and/or institutions in each country between 2019 and 2023, excluding self-citations. (SCImago)
- Total number of AI patents filed through the WIPO between 2019 and 2023. (WIPO)
- Ranking in the United Nations e-Government Development Index, which measures government digitization. (United Nations)
- Total number of active handset-based and computer-based (i.e., connected by USB/dongle) mobile-broadband subscriptions, to gauge relative magnitudes of digital data generation. (International Telecommunication Union)
Energy:
- The cost of electricity for a typical commercial/industrial user per kilowatt-hour, based on the “largest business city” within each country. (fDi Benchmark)
Computing Power:
- Existing data center capacity, including hyperscaler, colocation, and enterprise facilities, measured in gigawatts. The only exception is China, for which demand has been used instead, as overall capacity in China is difficult to estimate. (BCG Henderson Institute analysis of country and industry reports)
- Binary score for access to cutting-edge semiconductors optimized for AI workloads, such as Nvidia’s A100 or H100 chips. Countries and regions with no formal access barriers receive a score of 1; those facing such barriers (in the form of export restrictions, for example) receive a score of 0. (US Department of Commerce; BCG Henderson Institute analysis)
- Binary score for access to tier 2 semiconductors. All countries in our analysis received a score of 1, as they all have formally open access to this type of hardware. (BCG Henderson Institute analysis)
- Venture capital funding, from 2019 to 2024, based on the observed AI-directed share of investments by venture capital funds, by country (including, in the case of China, the sizeable pool of government VC funds devoted to AI). (Pitchbook)
- Corporate R&D spending by the 20 largest publicly traded tech companies, by country. (European Commission; BCG Henderson Institute)
- Sovereign wealth and public pension-fund investment power, adjusted for the share of assets under management in equities and alternative investments (therefore excluding bonds, real estate and infrastructure investments, and risk-free assets). (BCG Henderson Institute)
Current limitations in China’s access to state-of-the-art chips for AI model training and inference are likely to delay rather than impede further progress. Many Chinese companies reportedly retain access to chips, and Nvidia has designed models not covered by US trade restrictions; Chinese companies acquired $5 billion worth of these chips in 2023.
Furthermore, China is investing aggressively in its domestic chip manufacturing capacity: the government has pledged approximately $40 billion, and Huawei recently released its Ascend 910 chips, optimized for AI workloads. Regardless of whether it can compete with Nvidia at the cutting edge of computing hardware—and especially the software layer on top of it—Huawei has reached a milestone in recent months with the training of a high-performing LLM, iFlytek’s Xinghuo 4, entirely on its Ascend platform.
Chinese companies also have ambitions outside their home market. Alibaba, for example, is expanding its data center footprint in Malaysia, Philippines, Thailand, South Korea, and Mexico—extending the reach of its Qwen family of generative AI models.
GenAI Middle Powers on the Rise
This US–China story has fueled the “two superpowers” narrative—and convincingly so. But momentum is building in other parts of the world. The EU, for example, has strong complementarity among member states with established strengths. In the Gulf, highly concentrated and agile capital, coupled with affordable energy, is accelerating progress even in the absence of a robust, established tech sector. South Korea and Japan both have strong technology sectors, with the capital to scale.
Made in the European Union
For some, what may be out of reach at the individual country level becomes feasible at the bloc level. The EU stands to benefit from the complementarity of enablers among member states and the scale it can achieve as a unified market.
Building on Distributed Strength. The EU is already home to a nascent GenAI startup ecosystem. France-based Mistral has contributed seven of the world’s top LLMs and has received $1.2 billion in funding to date. The German startup Aleph Alpha has also developed powerful foundation models (two of the world’s top LLMs), though it has most recently pivoted to industry-specific applications.
Smaller GenAI startups are also attracting investment. Kyutai, founded in 2023, has received around $350 million and already released its Moshi AI model, specialized in advanced speech; with $220 million in seed capital, H is pushing to develop productivity-enhancing AI agents; Poolside, meanwhile, has received investments worth $626 million to build a leading model for code generation; and Black Forest Labs has obtained around $150 million to further its text-to-image foundation model.
While these companies are modest in size and funding compared to US and Chinese counterparts, they have a strong foundation of talent on which to build. The EU has an extensive and growing pool of AI specialists, of which there are more than 100,000 in France and Germany alone. (See Exhibit 3.)
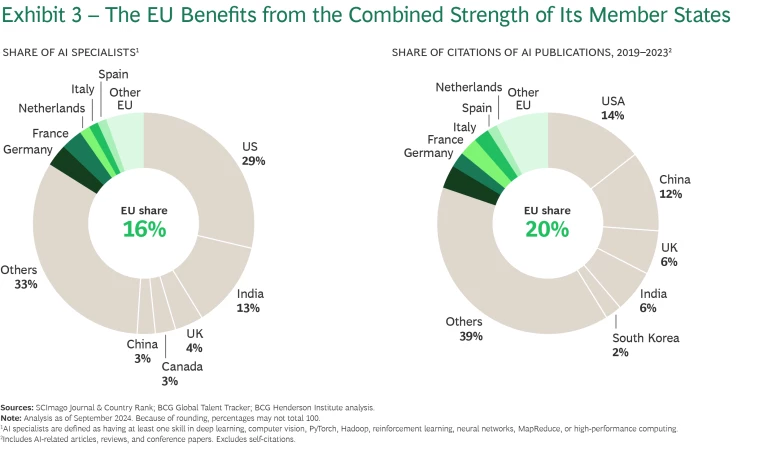
The EU also has the advantage of being a massive market, with a combined GDP of $18 trillion. It’s so large that its comparatively demanding regulatory framework for AI, including the General Data Protection Regulation (GDPR) and the recently enacted EU AI Act, is unlikely to dissuade GenAI suppliers from operating
The EU has the world’s third-largest data center capacity, after the US and China, with 8 gigawatts in 2024. While electricity prices in the region are high, considerable variability can be exploited: industrial electricity can cost 20% less in France, and close to 60% less in Sweden and Finland, than in Germany. Moreover, companies looking to supply GenAI models to the EU market may have no alternative to scaling data centers there for the aforementioned regulatory reasons.
Building on the above strengths, we already see complementarity in action: Mistral’s models, for example, are designed in France, trained on Italian supercomputers, and served to clients largely out of Swedish data centers—powered by the chips manufactured using EUV lithography equipment produced exclusively by Netherlands-based ASML.
Bridging the Funding Gap. The EU’s greatest challenge lies in securing the investments necessary to keep up with model scaling and expand data center infrastructure (which includes upgrading the power grid). The EU has a history of lagging investment in the tech sector, and therefore it has been unable to scale tech champions: while US GDP is 1.5 times greater than that of the EU, the market cap of the US share of the world’s 1,000 largest public tech companies is close to 18 times greater than the EU
As a result, EU-based technology firms have only a fraction of the investment muscle of their US counterparts: The 20 largest EU tech companies spent a combined $40 billion on R&D in 2022, compared to $200 billion for the 20 largest tech companies in the US. Unsurprisingly, GenAI startups in the EU have only received $3.5 billion in investments since 2019, or 5% of the private investment received by US-based GenAI startups.
There are signs of positive momentum, however. The Draghi report on European competitiveness and recent announcements by the European Investment Bank and the European Commission all stress the need for a stronger EU tech ecosystem—with the funding necessary for startups to scale within the bloc. There are pools of resources the EU could tap into, notably public R&D spending across member states, which averaged about $40 billion per year between 2016 and 2022—but doing so will require a concerted effort in a complex environment of distributed decision making.
Precedents for such collaboration exist, particularly in joint efforts like the creation of the European airplane consortium Airbus. But orchestration across national boundaries has also at times been challenging, as with the Gaia X initiative to develop trustworthy and sovereign cloud infrastructure for the EU. It remains to be seen whether the present policy momentum translates into timely action.
Made in Saudi Arabia or the UAE
Because of the high cost of building a top-performing GenAI ecosystem, some countries can use publicly available IP and invest strategically in talent and computing power. Countries may also rely on strong access to capital to outsource the development of models which they can nevertheless own and serve at scale. This approach is best suited to economies such as Saudi Arabia and the UAE, which have centralized access to significant capital resources like sovereign wealth funds.
Investing in Growth. In the Gulf region, Saudi Arabia and the UAE are each making significant investments to diversify their economies and contribute to the region’s broader technological acceleration.
The commitment to direct investments toward AI is clear. The UAE has launched a $10 billion AI VC fund—more than eight times the total funding received to date by Mistral, for example. Saudi Arabia, meanwhile, plans to invest $40 billion in AI development, on top of a recently announced plan to invest $100 billion in data center expansion (Project Transcendence) and its earlier $100 billion tech fund (Project Alat). These committed investments draw on much larger pools of capital: of the 20 largest sovereign wealth funds worldwide, UAE is home to five, totaling roughly $2 trillion in assets under management, while Saudi Arabia’s Public Investment Fund (PIF) manages $920 billion.
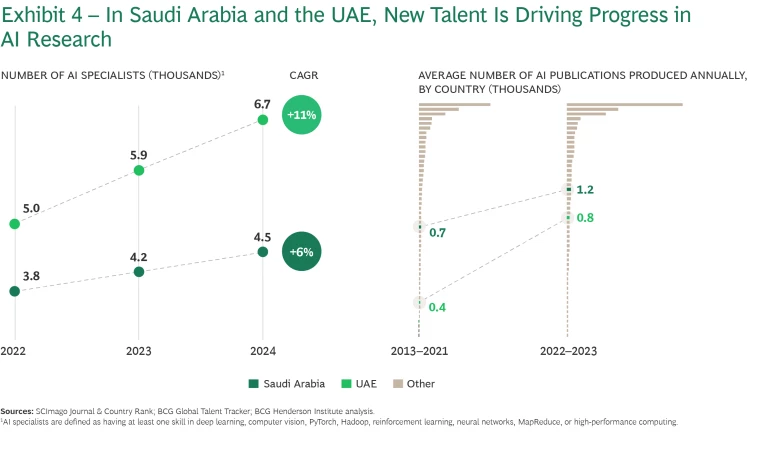
Both countries have invested heavily in building an AI R&D ecosystem through universities and sovereign-wealth-backed startups like G42 in the UAE. Since 2022, the AI talent pool in the UAE and Saudi Arabia has grown by 36% and 17%, respectively, and incoming net AI talent migration has grown by 40% and 70%. (See Exhibit 4.)
Data center infrastructure in the Gulf remains small in absolute terms, with Saudi Arabia and the UAE each around 0.4 gigawatts in capacity. But it is growing rapidly, in part enabled by the region’s low energy costs, which can be 30% to 50% lower than in the US on average. The recent easing of cutting-edge chip export restrictions to the UAE—which the Saudi government expects will eventually extend to Saudi Arabia as well—will contribute to sustained access to hardware optimized for AI workloads. Still, the pace of actual data center buildout is an open question.
Progress to date has been notable: Saudi Arabia’s Aramco has produced what is reportedly the largest industrial GenAI model in the world, and the Saudi Data and AI Authority released the leading Arabic LLM family, ALLaM, the largest versions of which are built on the basis of Meta’s Llama-2. Two of the Emirati Technology Innovation Institute’s Falcon models continue to be counted among the world’s top LLMs, and the UAE’s G42 has developed a high-performing Arabic model family, Jais—which speaks to the ability of developers in the region to access a robust corpus of Arabic data for model
Reaching Critical Mass. It remains to be seen whether Saudi Arabia and the UAE can sustain the AI talent pipeline needed to foster a self-sustaining model-development ecosystem. For all the impressive growth in this regard—with roughly 7,000 and 5,000 AI specialists now residing in the UAE and Saudi Arabia, respectively—the Gulf’s talent pool remains small compared to countries such as Germany (55,000 AI specialists) or France (50,000).
More important, for Saudi Arabia and the UAE to become profitable suppliers of GenAI, they have to reach sizeable technology export markets to justify the growing capital demands of GenAI model development. The UAE’s G42 appears to be doing precisely this with its Nanda LLM, designed to cater to Hindi-speaking consumers in India.
Made in Japan or South Korea
Some countries have capabilities across critical GenAI enablers but lack scale. For these nations, which include South Korea and Japan, investing to achieve this scale can create greater competitiveness, at least at a domestic or regional level. South Korea and Japan also have large internal markets and sizeable capital pools to provide the investment required.
The path for these countries is less certain given the challenges they face—but their strength in some key enablers suggests that it’s too soon to count them out.
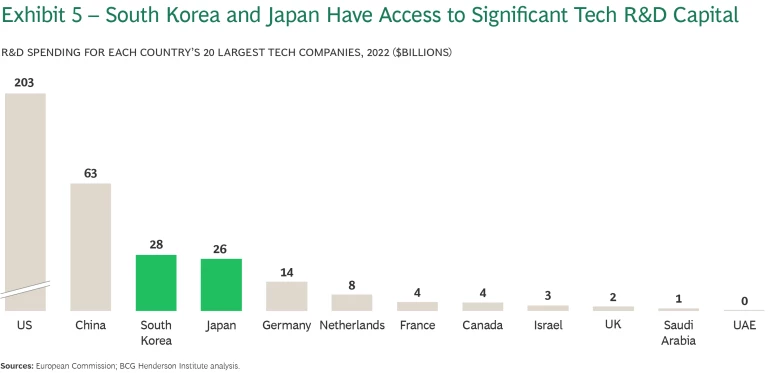
Capitalizing on a Legacy of Tech Strength. Both South Korea and Japan have strong, heavily concentrated tech ecosystems, dominated by conglomerates that are large R&D spenders. The annual R&D spending of South Korea and Japan’s 20 largest tech companies is greater than that of other GenAI middle powers, at $28 and $26 billion, respectively, compared to $14 billion in Germany and $4 billion in France. (See Exhibit 5.) This focus on R&D translates into strong performance on patents: South Korea and Japan rank first and third among GenAI middle powers in AI patents since 2013.
South Korea and Japan also benefit from their important positions along the hardware value chain, which gives them reliable access to top-quality chips. South Korea holds an approximately 30% market share in high-end (sub-10 nanometer) chip manufacturing, although it trails Nvidia on cutting-edge chips and is expected to lose share in the coming years . Both South Korea and Japan are leading exporters of critical inputs in chip making: indium for South Korea and photoresist processing, material removal and cleaning, manufacturing automation equipment, and arsenic for Japan.
While neither country has produced world-class LLMs to date, there are visible efforts around foundation model development. In South Korea, Samsung and Naver have built their own LLMs—as has telecom giant KT Corp, using AI chips manufactured domestically. Naver, as the largest internet search engine in the country, benefits from its access to data (South Korea is one of only four countries where Google doesn’t dominate the Internet search market).
In Japan, a partnership between universities and private companies recently released the open-source Fugaku-LLM, a model that has strong performance in Japanese-language benchmarks and trained using the Riken supercomputer Fugaku. Others, like tech conglomerate Rakuten, have used foreign open-source models like Llama and Mistral, which are then optimized for the Japanese language.
Finding Sufficient Market Demand and Computing Power. To justify the requisite investments, South Korea and Japan would need to secure large enough target markets for their generative intelligence. South Korea is less advantaged here than Japan, whose economy is roughly two and a half times as large. But South Korean developers appear to be more aggressively moving to secure export markets for GenAI: Naver, for instance, is partnering with Saudi Arabia to jointly develop an Arabic language LLM, while other South Korean companies are building specialized models targeting the Thai, Vietnamese, and Malaysian markets, among others.
Japan has a larger market and greater data center infrastructure, at 1.9 gigawatts of capacity for 2024. This is on par with Germany and well ahead of South Korea’s 0.9 gigawatts. (Planned construction could double capacity in South Korea in the coming years, capitalizing on comparatively low energy prices.) It is not clear yet, however, whether Japanese model developers will be able to compete as suppliers of GenAI with US hyperscalers, many of which are increasing investments to expand their footprint in Japan; to date, no obvious champion for domestic GenAI model development has emerged there.
In South Korea, where Naver and Samsung are clearly making inroads, it remains to be seen if funding will suffice to allow these companies to keep pace with the world’s leading models, which continue to scale. This will largely depend on Samsung, which accounts for 70% of total R&D spending among the 20 largest tech companies in the country.
In both South Korea and Japan, the odds of securing a place as competitive domestic and possibly regional suppliers of GenAI may depend on a concerted effort that capitalizes on the history of strong partnership between the private and public sectors around strategic national priorities. Both governments have voiced strong government-backed AI ambitions; it remains to be seen how those ambitions translate into action, and how quickly.
Betting on Research Breakthroughs
LLMs are largely developed using open IP; the architecture on which they are built was first published by Google in 2017. Increasingly, however, companies are improving LLMs through engineering innovations that are layered onto that open IP. Yet even those improvements fall within the current paradigm of scale, which makes capital intensity and computing-power capacity critical sources of advantage.
This paradigm is not the end of fundamental innovation in AI. It is possible that new approaches that don’t rely on the original architecture of today’s LLMs can deliver superior performance at lower computational cost. Such innovation, if kept proprietary, would reshuffle today’s sources of advantage—privileging whoever is in possession of it.
Breakthrough innovations of this kind could come from the US, China, or the GenAI middle powers—but also from countries with a strong legacy of AI R&D but limited strength across today’s key enablers. Notably, the UK, Canada, and (to a lesser extent) Israel are strong centers of AI research and have made important contributions to the field. (See Exhibit 6.)
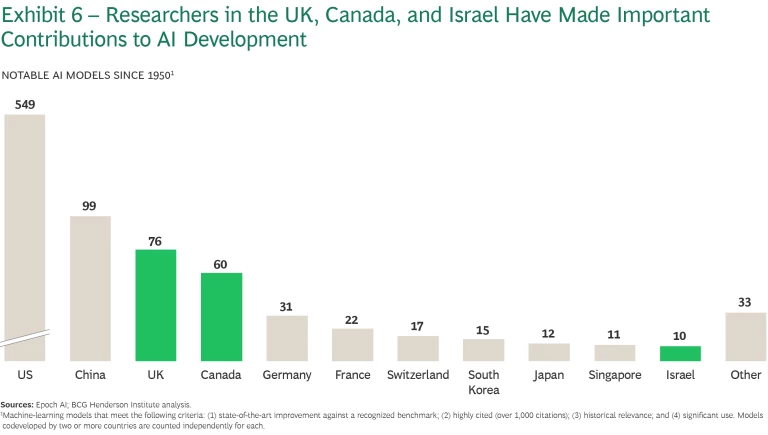
Canada and the UK are home to a sizable share of the world’s top AI scholars; they have produced many notable AI models and have published some of the most important AI research papers. Both countries also rank highly in terms of the number of AI specialists residing there—the UK is fourth in the world and Canada is seventh.
This strong bench of talent has driven economic benefit for both countries. Canadian-based GenAI startup Cohere has produced four of the world’s top LLMs (all from the Command model family). The UK-produced DeepMind, one of the world’s top AI research labs, was acquired by Google in 2014 and has been at the heart of the company’s AI innovations, including AlphaGo, AlphaFold, and Gemini—one of the world’s top LLM families.
Israel, with a significantly smaller population than the UK and Canada, is still among the 15 countries with the most top AI researchers and has one of the highest numbers of AI specialists on a per capita basis. Despite its small scale, Israel’s strong concentration of AI talent has enabled it to develop five of the top LLMs (from the Jamba and Jurassic model families) through AI21 Labs.
A Call to Action for Leaders
Both company and country leaders need to be able to navigate the new geopolitics of AI by building their geopolitical muscle: the ability to sense coming shifts and adapt their operating model.
On the one hand, companies have a vested interest in a diversified supply of GenAI. The lessons of Covid are stark: a massive disruption can create severe supply-chain chokepoints and destroy value, which underlines the importance of optionality.
Executives will also need to be able to ensure smooth operations across the geographies in which they do business, despite differences in regulation, language, and legacy tech infrastructure. Certain models may be available in some countries but not in others, which argues for building regional GenAI supply chains and localizing part of a multinational business’s tech operations.
If all options originate in just two countries, it could lead to disruptions in the technology’s availability due to geopolitical shocks. CEOs should therefore consider a portfolio approach to generative AI. Utilizing a variety of models—even a combination of open source and closed—can enable companies to exploit individual models’ specific strengths while increasing options for model access across jurisdictions.
A Landscape of Open-Source, Open-Weights, and Closed Models
Historically, open-source software refers to freely available source code that can be distributed and modified at the user’s discretion. By contrast, proprietary or closed-source software cannot be publicly accessed nor modified without the creator’s permission.
In the case of generative AI, an open-source LLM would involve the free release of the model architecture, source code for training, data for training, and pretrained weights. Fully open-source models, like HuggingFace’s SmolLM, are the exception. More common are open-weights models like Meta’s Llama; with open weights, the model can be fine-tuned for specific purposes but cannot be fully recreated. While partially or fully open models have tended to lag proprietary ones in terms of capabilities and performance, that gap appears to be closing, driven particularly by Meta’s Llama model family.
Open-source and open-weights models have helped accelerate and spread innovations, often across national borders. For example, San Francisco-based Abacus AI has recently released a model adapted from Alibaba’s open-source Qwen model.
The strategic rationale for releasing open-source models varies across developers. For Alibaba, open source is widely regarded as a play to drive its models’ uptake—which can then be monetized through Alibaba’s cloud infrastructure for inference. For Meta, which does not provide cloud services to third parties, its open-weights Llama models are primarily a strategic bet on its own technological self-sufficiency, given the impact GenAI is expected to have on its core advertising business.
From the point of view of companies using rather than developing GenAI, open-source and (to a lesser extent) open-weights models offer some advantages for customization and security, as they can be hosted on premises—but maintaining these models requires more investment in talent and computing power compared to off-the-shelf, proprietary ones.
The regulation of open-source models is a complex policy question, with some arguing that even partially open models give too much power to “bad actors” and others claiming that the best countermeasure to malicious AI use is widespread access to AI technology itself.
On the other hand, because AI sovereignty may soon become a critical source of national security, economic value, and soft power, governments of countries or in regions with potential to become GenAI middle powers should consider what it would take to claim the space. Ensuring robustness across enablers will involve different immediate priorities for each: some will need to focus on attracting and retaining the right talent, others will need to emphasize investment to boost domestic AI champions, and still others may need to prioritize expanding their data center infrastructure.
The choices that both private and public sector leaders make will be shaped by regulatory and policy action that may create drastic shifts, for example, in tariffs, the restriction of international talent flows, or data regulations. All the while, the tech landscape is quickly evolving, and the cost of entry is rising just as rapidly. In this environment, the ability to understand the full GenAI landscape will be critical.